An energy-efficient floating-point compute SRAM with pipelined in-memory bit-parallel exponent and bitwise mantissa processing
ELECTRONICS LETTERS(2023)
摘要
The promise of compute-in-memory (CIM) for energy-efficient deep neural network (DNN) tasks has been demonstrated. However, most previous CIM works typically focus on low-precision DNN computing. To enable high-precision DNN computing, this work presents a novel SRAM-CIM design that fully supports half-precision floating-point (FP16) MAC operations. To maximize the energy efficiency, an efficient in-memory bit-parallel approach for conducting exponent operations and the bitwise in-memory booth encoder for reducing mantissa multiplication latency are proposed. Moreover, by enabling the pipeline of exponent and mantissa processing, the hardware utilization is improved with high throughput achieved. The proposed design is analyzed in 40 nm CMOS technology. The evaluation shows that the SRAM-CIM achieves a frequency of 714 MHz and a peak energy efficiency of 1.53 TFLOPS/W.
更多查看译文
关键词
digital circuits, memory architecture, SRAM chips
AI 理解论文
溯源树
样例
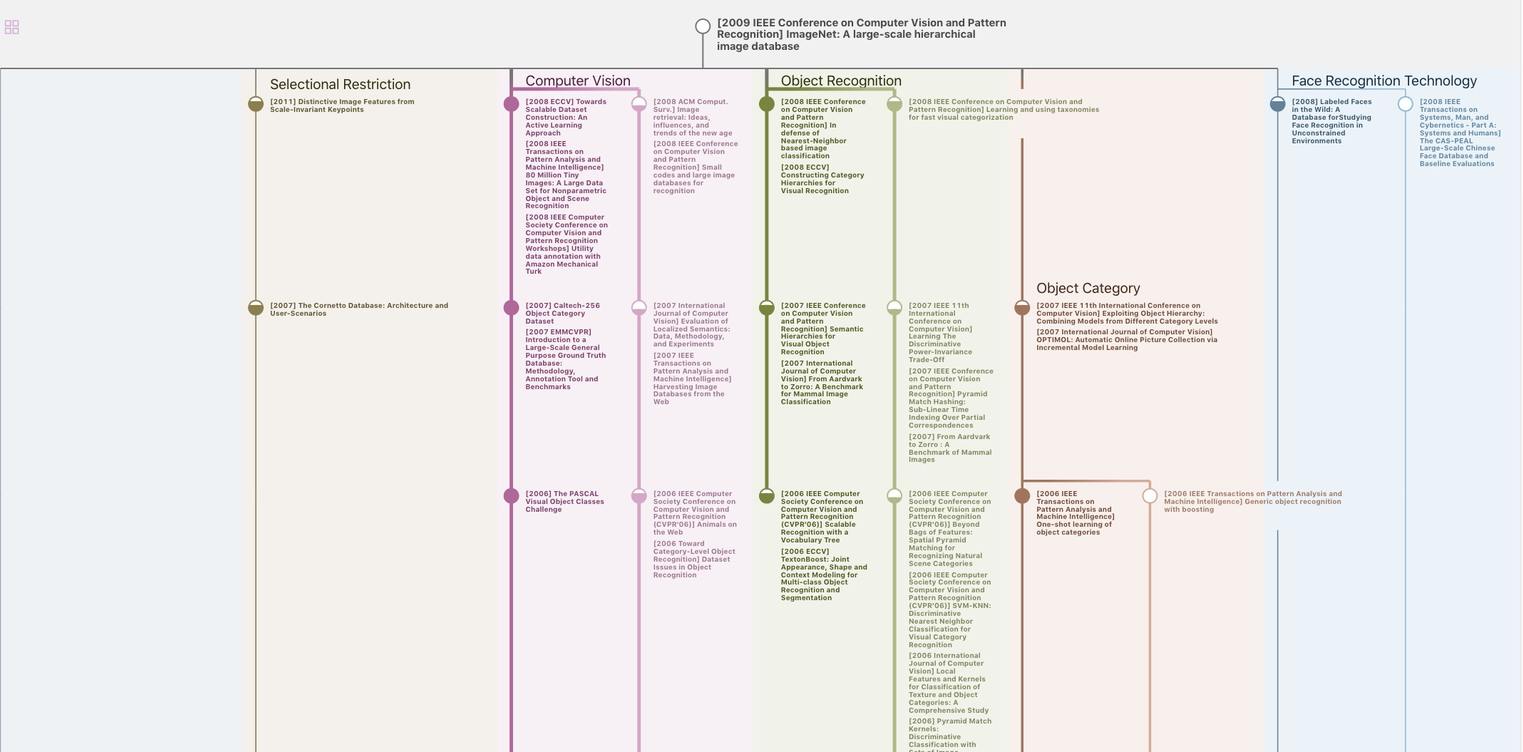
生成溯源树,研究论文发展脉络
Chat Paper
正在生成论文摘要