Learning Hierarchical Graph-Based Policy for Goal-Reaching in Unknown Environments.
IEEE Robotics Autom. Lett.(2024)
摘要
Goal-reaching in unknown environments is one of the essential tasks in robot applications. Large-scale perception and long-horizon decision-making are the keys to solving this task as the operation scope expands or complexity rises. Existing navigation methods may suffer from degraded performance in complicated environments induced by scalability-limited map representation or greedy decision strategy. We propose the path-extended graph as a compact map representation providing sufficient structural information within a reasonable receptive field and incorporate it into a hierarchical policy for higher efficiency and generalizability. The path-extended graph contains the concise topology of environment structure and frontier layout for large-scale perception, avoiding the impact of redundant information. The hierarchical policy solves long-horizon non-myopic decision-making through a high-level frontier selection policy using deep reinforcement learning (DRL) and a low-level motion controller that handles path planning and collision avoidance. Simulation and real-world experiments demonstrate that our method outperforms other competitive approaches in avoiding redundant movement and achieves efficient goal-reaching, especially in complex environments.
更多查看译文
关键词
Service Robotics,Reinforcement Learning,Integrated Planning and Learning
AI 理解论文
溯源树
样例
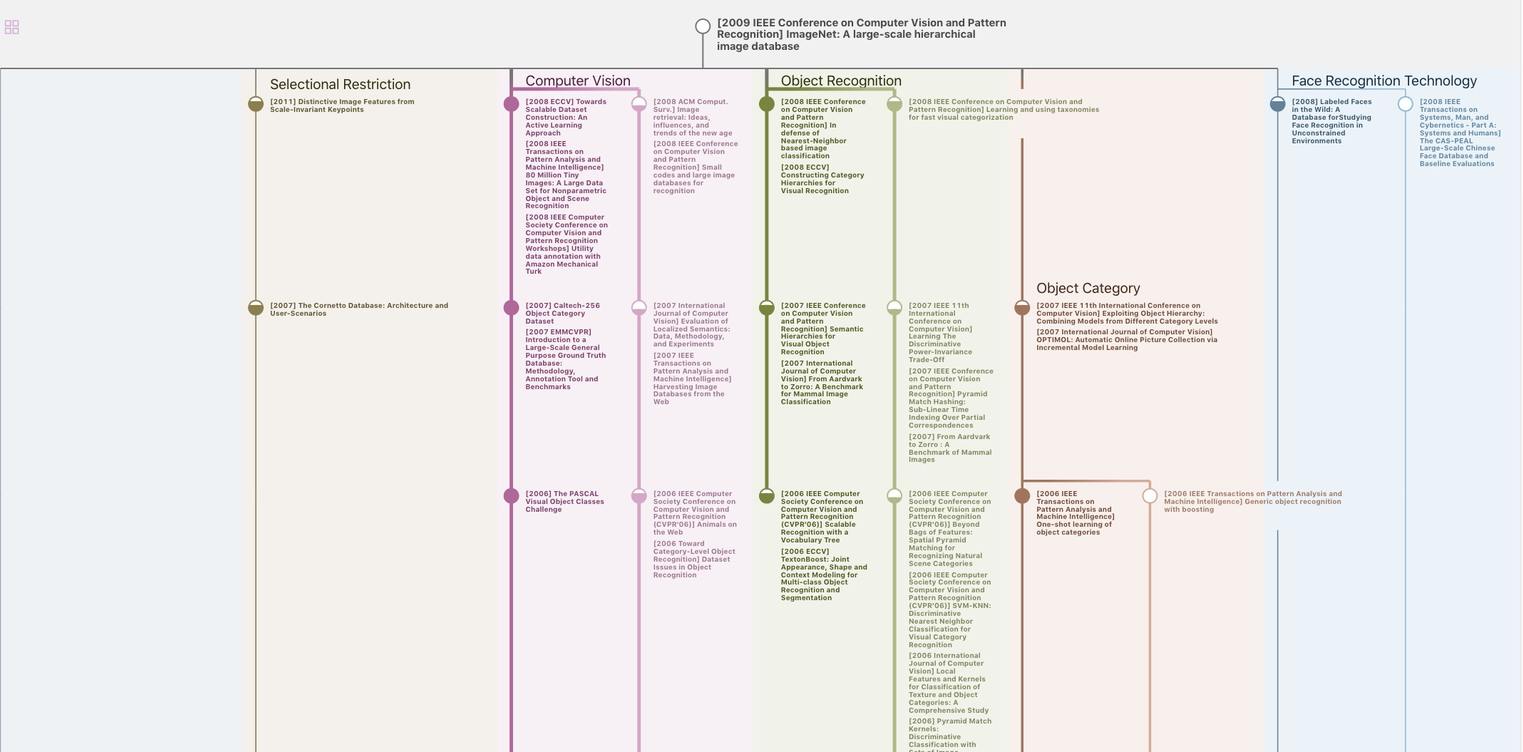
生成溯源树,研究论文发展脉络
Chat Paper
正在生成论文摘要