Optimizing methanol synthesis from CO2 using graphene-based heterogeneous photocatalyst under RSM and ANN-driven parametric optimization for achieving better suitability
RSC ADVANCES(2024)
摘要
Assessment of the performance of linear and nonlinear regression-based methods for estimating in situ catalytic CO2 transformations employing TiO2/Cu coupled with hydrogen exfoliation graphene (HEG) has been investigated. The yield of methanol was thoroughly optimized and predicted using response surface methodology (RSM) and artificial neural network (ANN) model after rigorous experimentation and comparison. Amongst the different types of HEG loading from 10 to 40 wt%, the 30 wt% in the HEG-TiO2/Cu assisted photosynthetic catalyst was found to be successful in providing the highest conversion efficiency of methanol from CO2. The most influencing parameters, HEG dosing and inflow rate of CO2, were found to affect the conversion rate in the acidic reaction regime (at pH of 3). According to RSM and ANN, the optimum methanol yields were 36.3 mg g(-1) of catalyst and 37.3 mg g(-1) of catalyst, respectively. Through the comparison of performances using the least squared error analysis, the nonlinear regression-based ANN showed a better determination coefficient (overall R-2 > 0.985) than the linear regression-based RSM model (overall R-2 similar to 0.97). Even though both models performed well, ANN, consisting of 9 neurons in the input and 1 hidden layer, could predict optimum results closer to RSM in terms of agreement with the experimental outcome.
更多查看译文
AI 理解论文
溯源树
样例
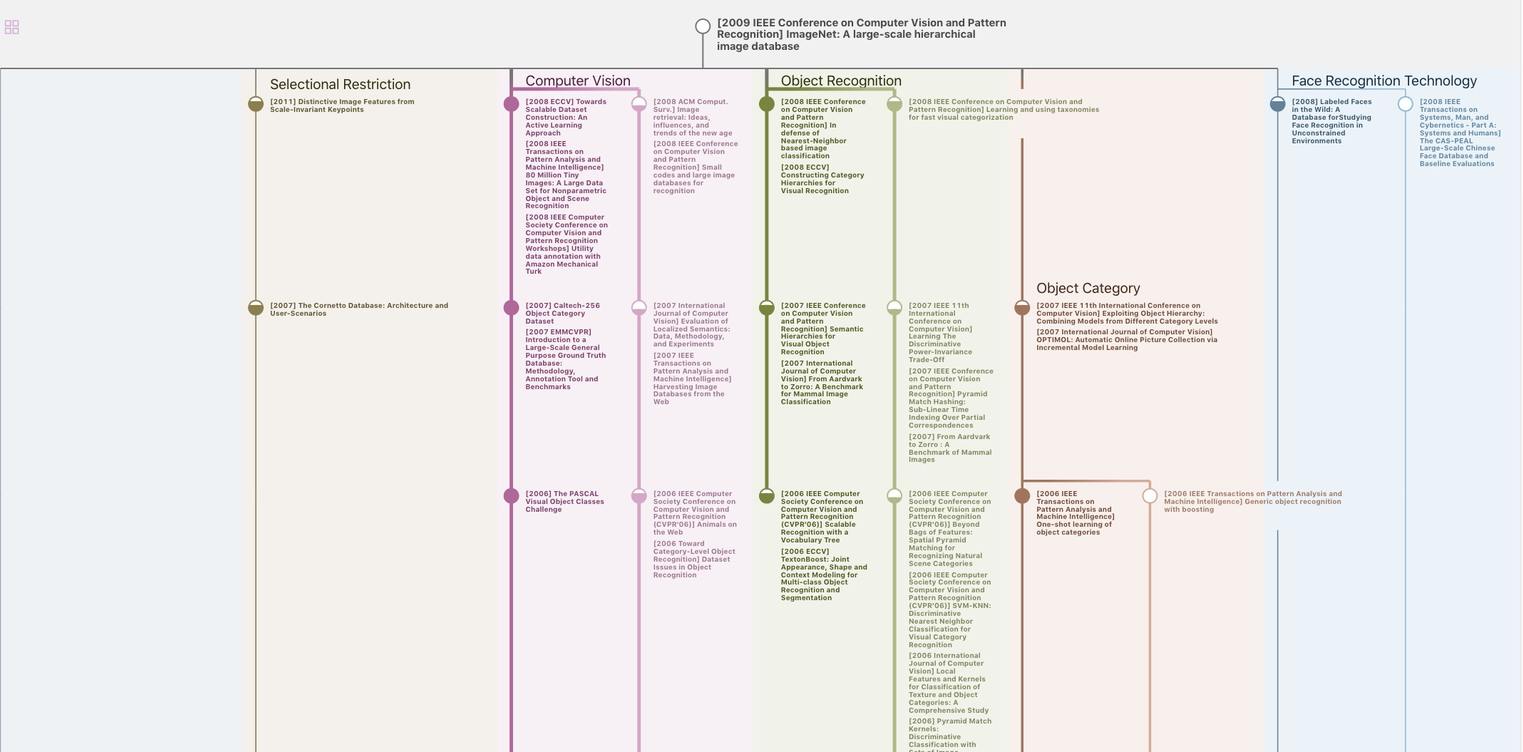
生成溯源树,研究论文发展脉络
Chat Paper
正在生成论文摘要