Abstract 6184: A novel approach to Barrett's esophagus risk stratification: Whole-slide image analysis for aneuploidy detection
Cancer Research(2024)
摘要
Abstract Background Barrett’s esophagus (BE) is the only known precursor of esophageal adenocarcinoma (EAC) and there is a need for biomarkers in BE for risk stratification for cancer progression. Aneuploidy has been suggested as a factor in the development, initiation or progression of EAC in patients with BE, and it has been found predictive for EAC progression (Hadjinicolaou, et al. 2020, Sikkema, et al. 2009). However, current assays for detecting aneuploidy require valuable tissue, hence validation studies are limited. We hypothesise that routine histology images can be used to detect ploidy status. We aim to develop a sensitive, accurate, and easy-to-use deep learning tool for this purpose. Methods We used a weakly supervised deep learning-based approach called clustering-constrained-attention multiple-instance learning (CLAM) (Lu et al., 2021) to detect aneuploidy on hematoxylin and eosin-stained whole slide images of endoscopical biopsies from BE, for which the ploidy status had been determined by flow cytometry. To benchmark the model’s performance, we also trained a traditional fully supervised algorithm, ResNet50 (He et al., 2016), with the same dataset. The models were trained on 388 slides (51 aneuploid) from the Seattle Barrett’s Esophagus Annotated Resource (BEAR) and then applied to an independent test cohort of BEAR patients, consisting of 279 slides (36 aneuploid). Results The multi-attention-branch CLAM model achieved AUC of 0.82 and 76.4% balanced accuracy for aneuploidy on the internal test subset (10% of the cohort). On the independent test dataset, the model achieved AUC of 0.85 and 79.3% balanced accuracy, while performance of the fully supervised model was AUC of 0.65 and 33.1 balanced accuracy. In a challenging subset for image-based diagnosis, including 253 samples (29 aneuploid) without dysplasia or atypical mitosis (a major feature of aneuploidy samples) the model achieved a 67.6% accuracy and an AUC of 0.83. Both the flow results (p=2.84e-7) and the model's predictions (p=2.18e-3) revealed a correlation between progression to EAC and ploidy status. Conclusion We developed a deep learning model for predicting aneuploidy in BE biopsies. The weakly-supervised approach can perform much better compared to the traditional fully-supervised model. Aneuploidy is correlated with cancer progression and stands as a promising candidate for the prediction of cancer progression in BE patients. Although atypical mitosis is the primary histological indicator of aneuploidy, our model can still make aneuploid predictions in the absence of atypical mitosis, as it doesn't rely solely on a single parameter. Our model is efficient, capable of processing hundreds of samples resource-effectively, and thus an ideal adjunct to standard histologic evaluation. This classifier may facilitate molecular-based improvements in identifying individuals at high risk of progression. Citation Format: Caner Ercan, Xiaoxi Pan, Thomas G. Paulson, Matthew D. Stachler, Carlo C. Maley, Yinyin Yuan. A novel approach to Barrett's esophagus risk stratification: Whole-slide image analysis for aneuploidy detection [abstract]. In: Proceedings of the American Association for Cancer Research Annual Meeting 2024; Part 1 (Regular Abstracts); 2024 Apr 5-10; San Diego, CA. Philadelphia (PA): AACR; Cancer Res 2024;84(6_Suppl):Abstract nr 6184.
更多查看译文
AI 理解论文
溯源树
样例
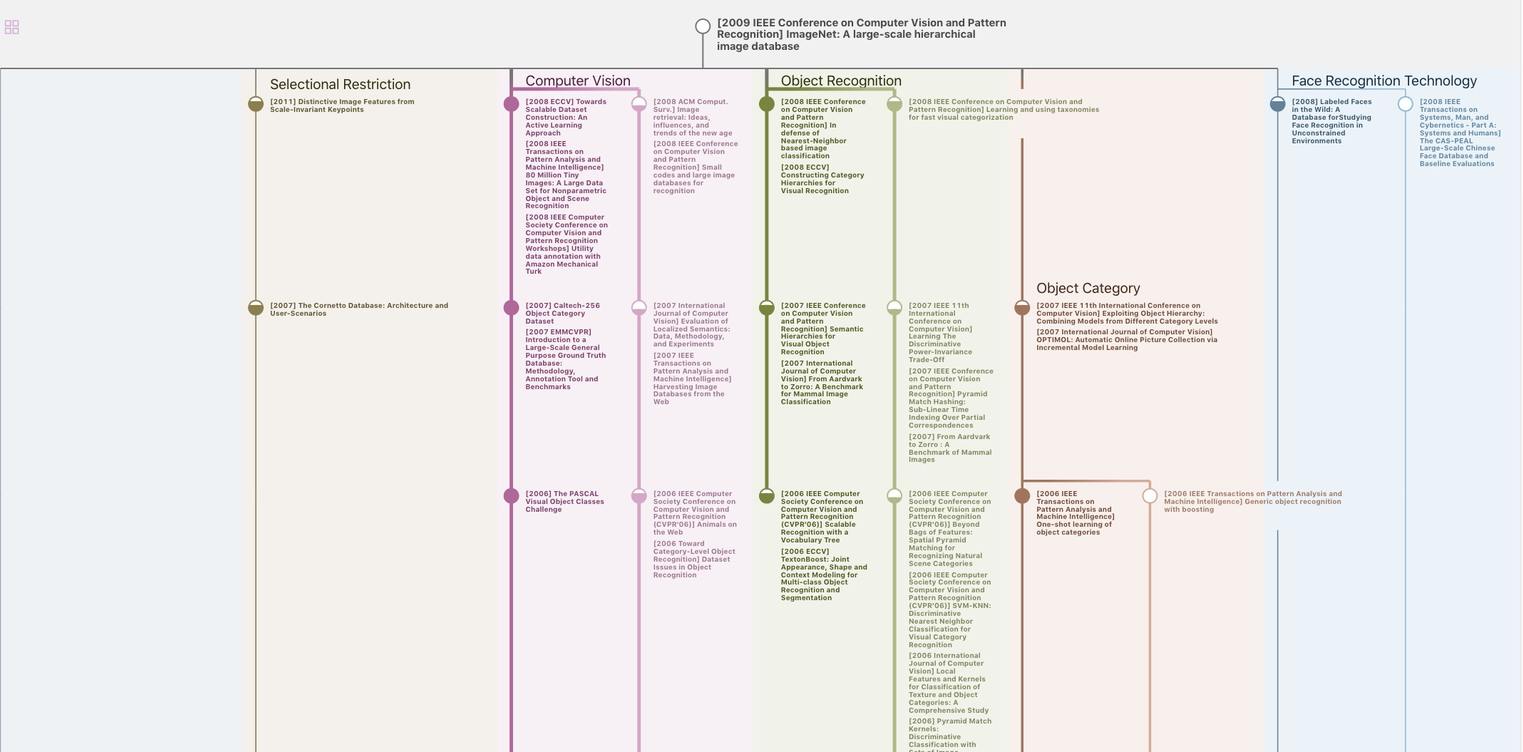
生成溯源树,研究论文发展脉络
Chat Paper
正在生成论文摘要