Accelerated Objective Gap and Gradient Norm Convergence for Gradient Descent via Long Steps
arxiv(2024)
摘要
This work considers gradient descent for L-smooth convex optimization with
stepsizes larger than the classic regime where descent can be ensured. The
stepsize schedules considered are similar to but differ slightly from the
concurrently developed silver stepsizes of Altschuler and Parillo. For one of
our stepsize sequences, we prove a O(1/N^1.2716…)
convergence rate in terms of objective gap decrease and for the other, we show
the same rate of decrease for the squared-gradient-norm. This first result
improves on the recent result of Altschuler and Parillo by a constant factor,
while the second results improve on the exponent of the prior best
squared-gradient-norm convergence guarantee of O(1/N).
更多查看译文
AI 理解论文
溯源树
样例
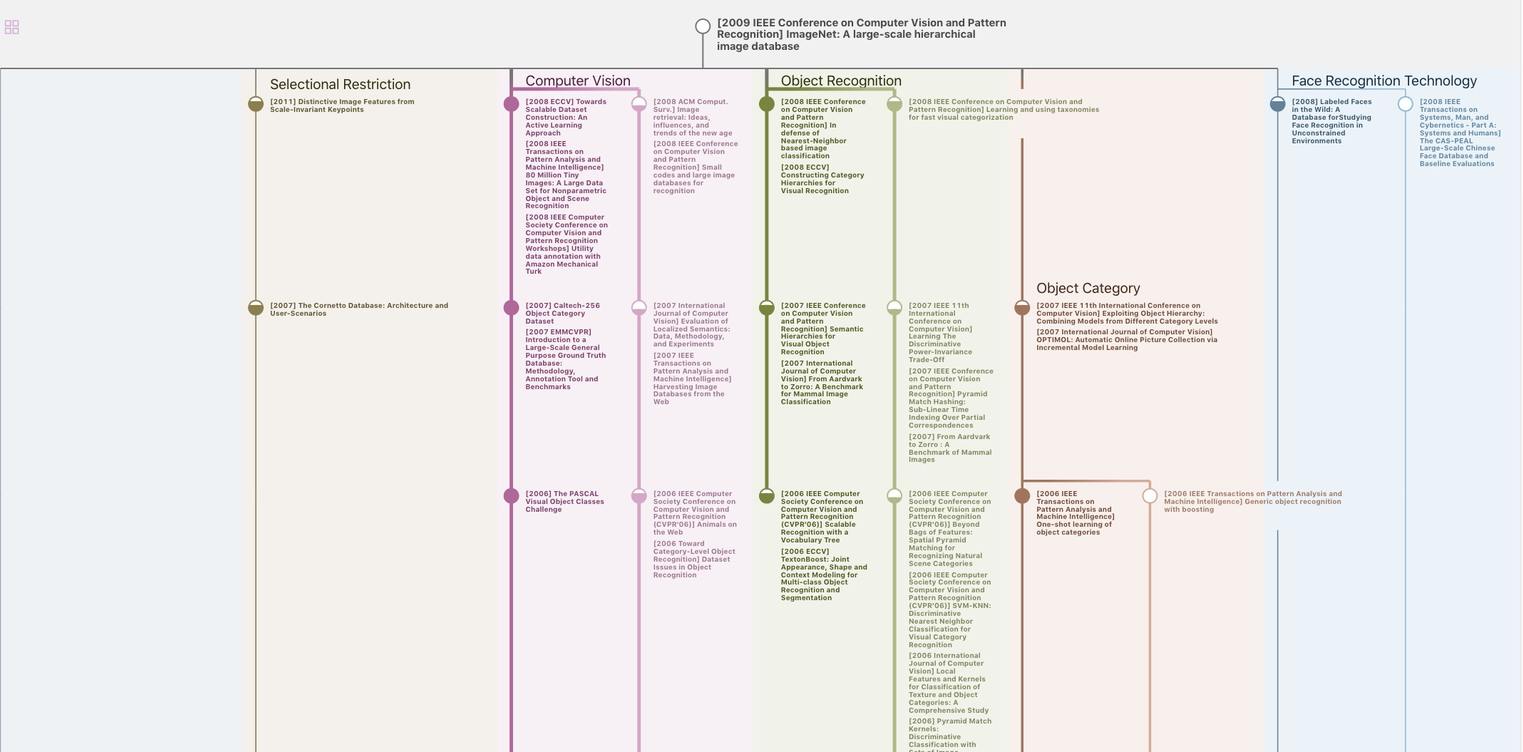
生成溯源树,研究论文发展脉络
Chat Paper
正在生成论文摘要