Collision avoidance control for limited perception unmanned surface vehicle swarm based on proximal policy optimization
Journal of the Franklin Institute(2024)
摘要
In order to ensure the safe and coordinated operation of unmanned surface vehicle (USV) swarm in complex marine environments, the primary problem is collision avoidance control (CAC). However, the limited perception, environmental uncertainty and multi-source complexity bring significant challenges to the efficient collaboration and CAC of the USV swarm. To overcome the above challenges, this paper aims to propose a distributed CAC method for USVs based on the proximal policy optimization (PPO). This method does not necessitate precise system models and is capable of autonomous learning, effectively adapting to unknown environments. In terms of CAC, unlike designing reward functions based solely on the distance from obstacles, we additionally consider the velocity of obstacles, and combine optimal reciprocal collision avoidance (ORCA) to design a reward function. We further consider the limited perception range of USVs and construct a bidirectional gated recurrent unit (BiGRU) network to extract features of variable length observation data, effectively overcoming the problem of dimensionality in observable data. Moreover, we construct a high-quality USV swarm simulation environment using the Gazebo 3D physics engine, which is used for testing the generalization capability of collision avoidance policy. Finally, to verify the effectiveness of the policy learning and optimization, a series of experiments are conducted in various scenarios, network architectures, and control methods. The experimental results indicate that our approach has remarkable superiority in terms of travel time, average velocity, average reward, and success rate.
更多查看译文
关键词
USV swarm,Optimal reciprocal collision avoidance,Proximal policy optimization,Deep reinforcement learning
AI 理解论文
溯源树
样例
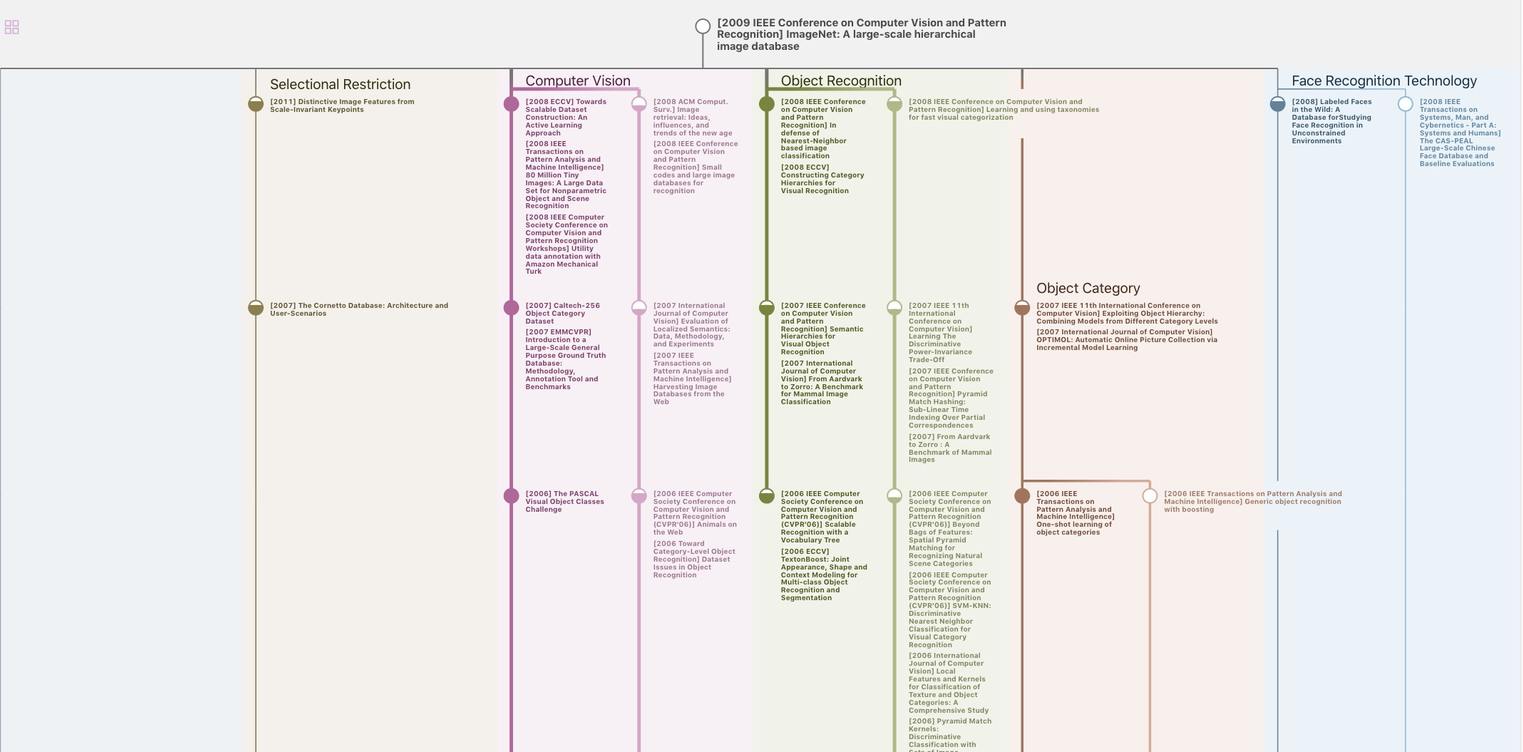
生成溯源树,研究论文发展脉络
Chat Paper
正在生成论文摘要