Multi-sensor fusion federated learning method of human posture recognition for dual-arm nursing robots
INFORMATION FUSION(2024)
摘要
Human posture estimation plays a significant role in the growth of intelligent nursing robot, a field that demands high accuracy and respect for privacy. Nevertheless, traditional approaches to enhancing datadriven studies in this domain often face challenges, primarily due to privacy concerns in sensitive healthcare environments. Federated Learning rises as the solution to the problem, as it not only improves the model learning ability but also protects the data privacy. In this paper, we proposed a Federated Learning Human Posture Recognition (FL-HPR) framework according to image and point cloud fusion. FL-HPR significantly enhances the information flow in the global model while ensuring the data privacy in local models, the benefits highlight the framework's potential in sensitive applications. Furthermore, the key innovation of FL-HPR is the optimization of the local dynamic graph edge convolution network of robot, which improves the recognition accuracy of individual body limb and enhance overall robustness. Experiments on Non-IID datasets illustrate that the presented FL-HPR remarkably outperforms non-federated learning methods, suggesting its potential to improve the accuracy of human joint estimation. The breakthrough indicates that the proposed FL-HPR can be integrated into the intelligent nursing robot in practical applications. For further explorations, the open-source code and videos are available at https://github.com/Hebut-LEVO/FL-HPR.
更多查看译文
关键词
Federated learning,Activity recognition,Motion capture,Sensor fusion,Nursing robots,Dynamic Graph CNN
AI 理解论文
溯源树
样例
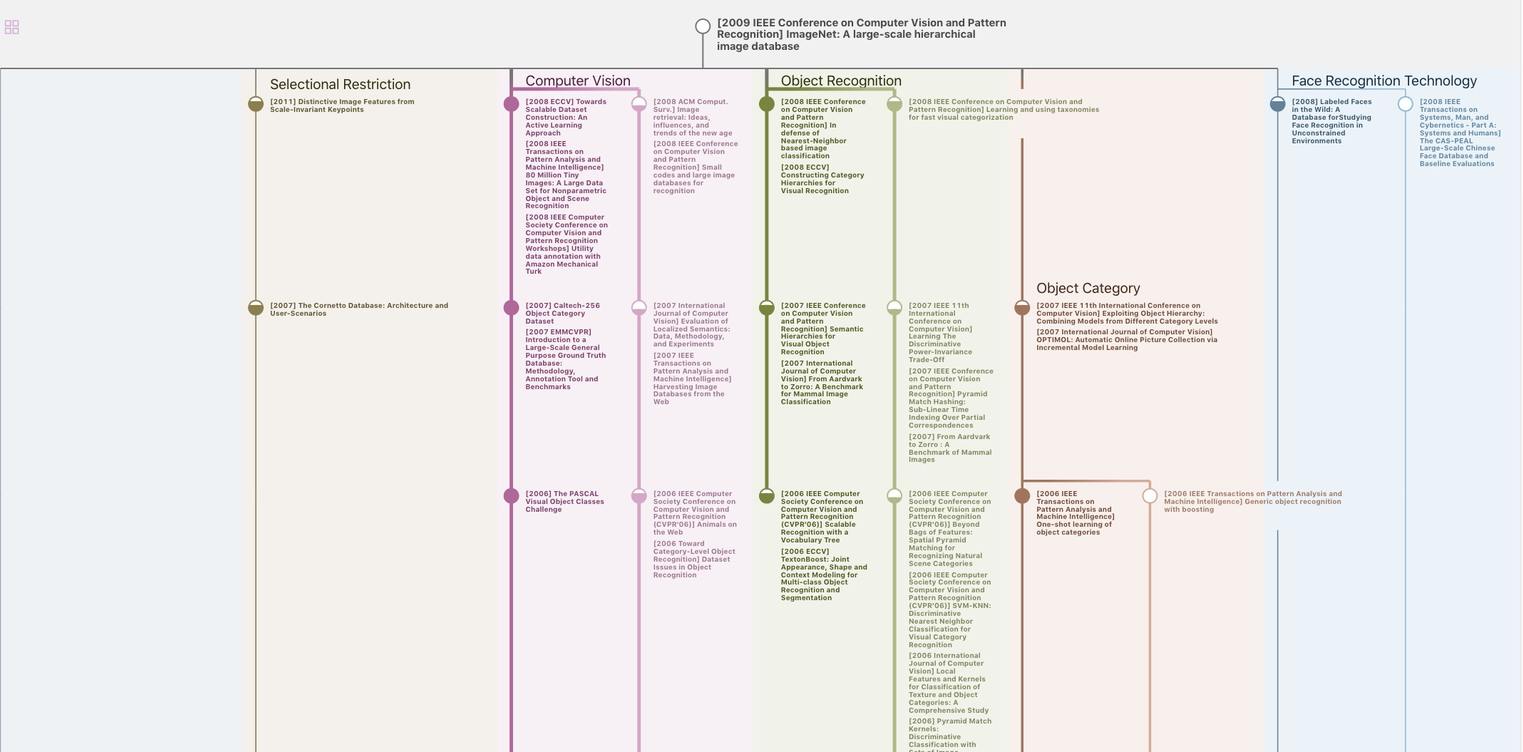
生成溯源树,研究论文发展脉络
Chat Paper
正在生成论文摘要