Federated temporal-context contrastive learning for fault diagnosis using multiple datasets with insufficient labels
ADVANCED ENGINEERING INFORMATICS(2024)
摘要
Insufficient data, lack of labelled data, and limited data sharing hinder deep-learning-based fault-diagnosis methods. Most of the existing methods focus on addressing only one of these issues and consequently lack practical applicability. The method proposed in this study addresses these issues simultaneously. First, a temporal-context contrastive learning method is proposed that combines the concepts of few-shot and selfsupervised learning. This method assists the feature extractor in learning fault data representations from unlabelled data through a specially designed loss function, thereby enabling the training of deep-learning models on small-scale, label-deficient datasets. Next, a federated learning framework is introduced to train a global model from multiple datasets without data sharing. In addition, a novel client contrastive loss function is proposed to address the issue of model performance degradation caused by different distributions among client datasets. Finally, experimental evaluations are conducted on public datasets, and the results demonstrate the effectiveness and superiority of the proposed method.
更多查看译文
关键词
Fault diagnosis,Deep learning,Self-supervised learning,Federated learning
AI 理解论文
溯源树
样例
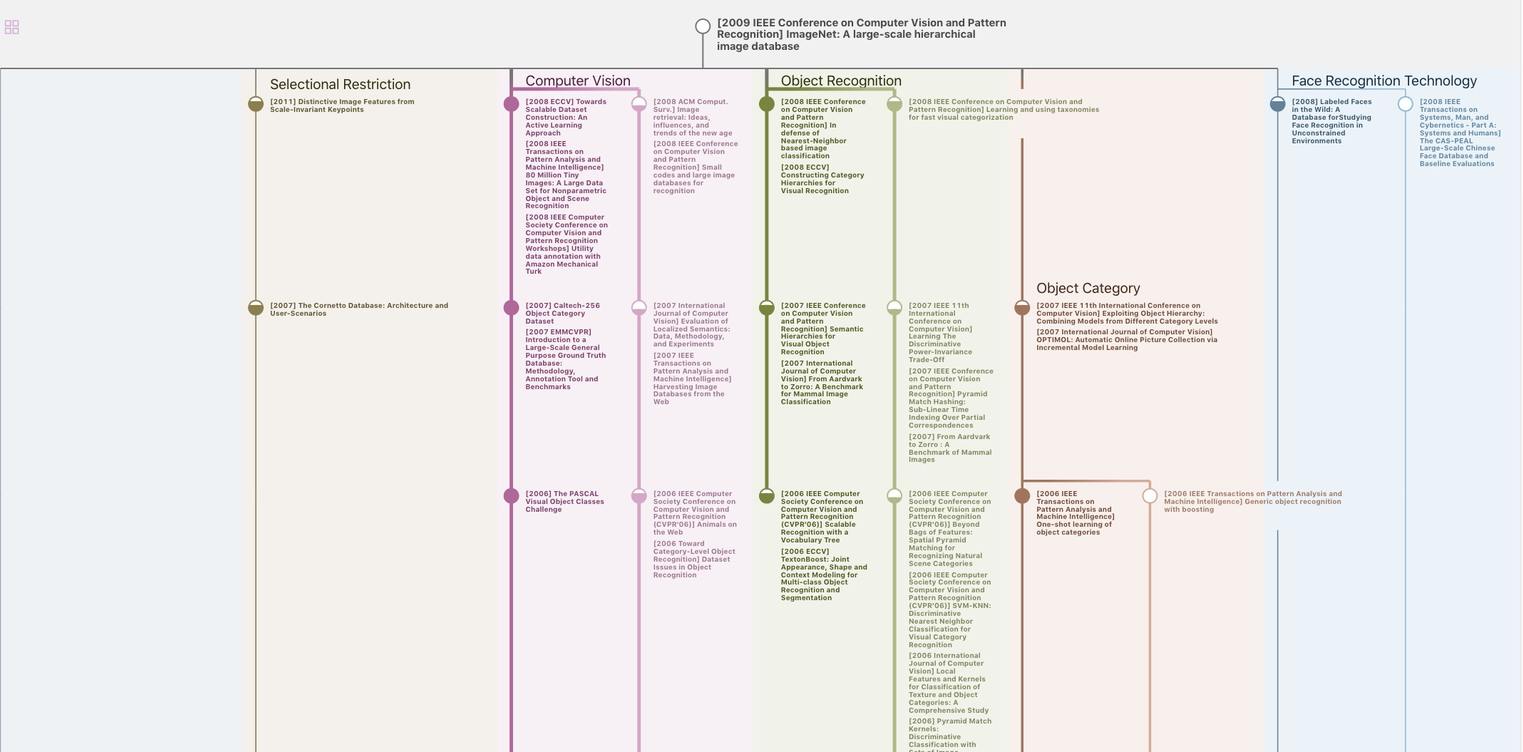
生成溯源树,研究论文发展脉络
Chat Paper
正在生成论文摘要