Pixel-Level Contrastive Pretrainer for Industrial Image Representation
IEEE TRANSACTIONS ON INSTRUMENTATION AND MEASUREMENT(2024)
摘要
Industrial quality inspection aims to identify defective parts in industrial production processes. Commonly used methods for industrial quality inspection rely on feature representations that have been pretrained on natural image datasets, such as ImageNet. However, these pretrained models are not specifically tailored for industrial scenarios and therefore do not transfer well to downstream industrial tasks. In this study, we have curated a large-scale industrial production dataset called Ind-2M, which is specifically collected from industrial scenarios. This dataset serves to enhance the industrial representation of pretraining models. Additionally, we propose a Pixel-level COntrastive (PiCO) pretrainer for industrial image representation. PiCO not only improves the global industrial representation through industrial production classification, but also enhances the local industrial representation through pixel-level self-supervision. Experimental results demonstrate that PiCO effectively transfers to downstream industrial tasks, such as multilabel defect classification and anomaly detection, outperforming existing pretrained methods. We hope PiCO can initiate a new paradigm for industrial image pretraining.
更多查看译文
关键词
Task analysis,Feature extraction,Anomaly detection,Transformers,Production,Inspection,Convolutional neural networks,Ind-2M,industrial image representation,Pixel-level COntrastive (PiCO),pixel-level contrastive learning,pretrainer
AI 理解论文
溯源树
样例
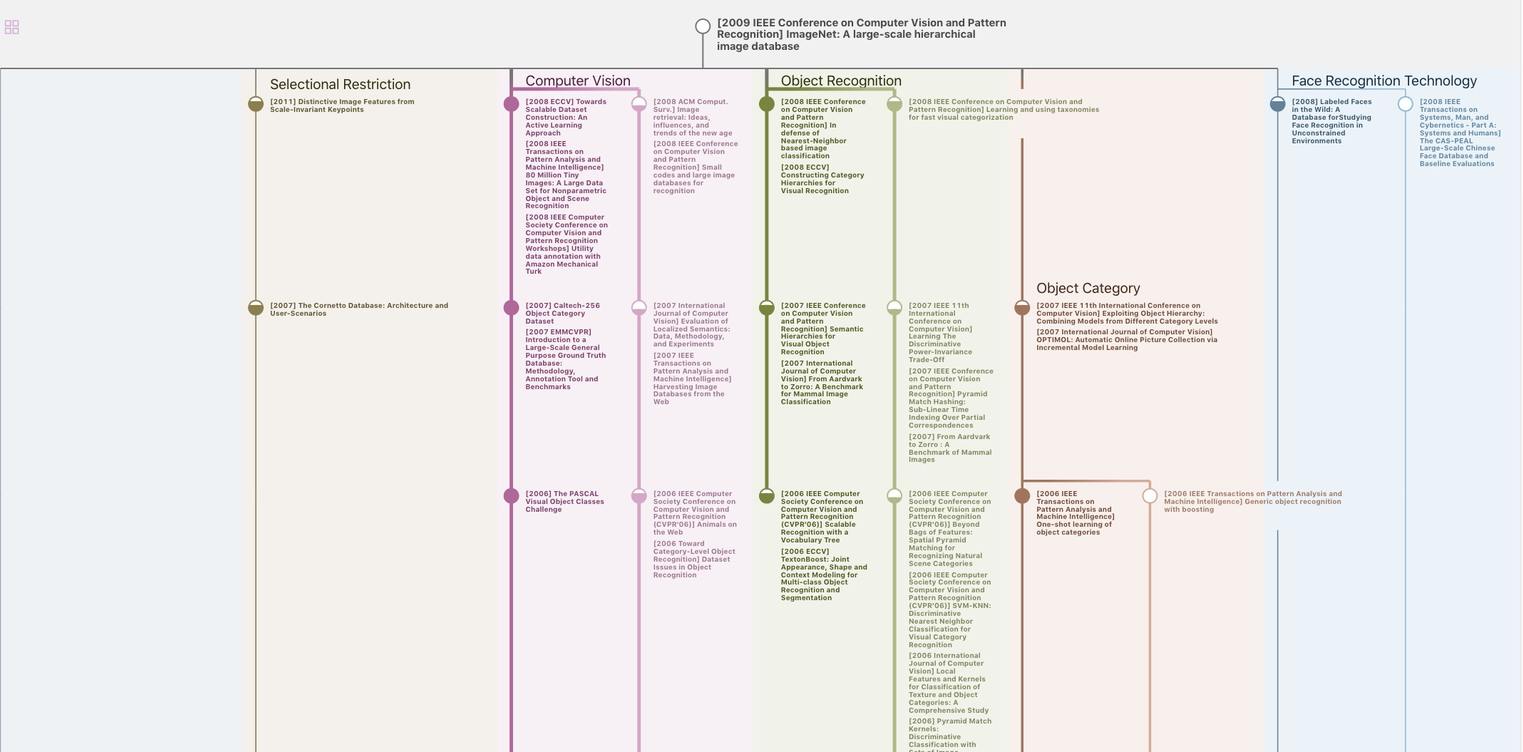
生成溯源树,研究论文发展脉络
Chat Paper
正在生成论文摘要