Dynamic job-shop scheduling using graph reinforcement learning with auxiliary strategy
JOURNAL OF MANUFACTURING SYSTEMS(2024)
摘要
The unpredictable variety of dynamic events in manufacturing systems poses a great challenge for tackling the job-shop scheduling problem (JSP), while most prior arts fail to strike a good balance between solution efficiency and dynamic adaptation. To this end, this paper outlines a graph reinforcement learning framework for solving dynamic JSP (DJSP) with stochastic processing time and machine breakdowns. The framework depicts DJSP as a Markov decision process (MDP) and expands the disjunctive graph representation of the state. Then a mixed graph Transformer network is proposed to extract state embeddings coupled with dynamic events, which combines the merits of two attention mechanisms and a spatial pyramid pooling module to flexibly fit different scheduling configurations. Further, a promising training algorithm called Phase Proximal Policy Optimization with Rollback is advanced to learn the optimal scheduling policy, which introduces an additional auxiliary phase to train the policy and value networks alternately for higher sample efficiency. Comprehensive experiments both on static benchmarks and dynamic instances as well as an actual engineering case indicate that the proposed framework exhibits significant superiority in fidelity and generalization compared to previous work in terms of solving DJSP.
更多查看译文
关键词
Dynamic job -shop scheduling,Deep reinforcement learning,Attention mechanism,Graph neural network,Auxiliary strategy
AI 理解论文
溯源树
样例
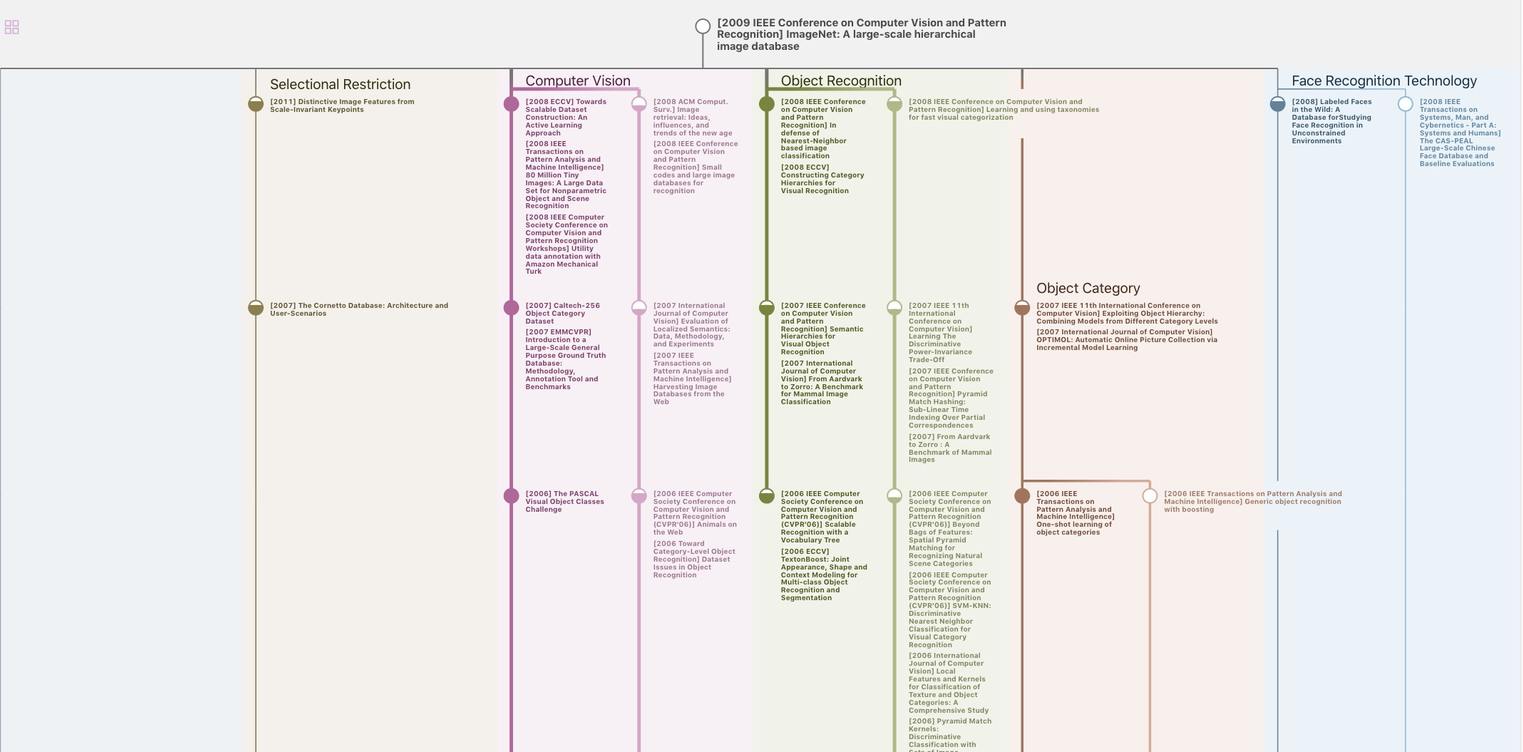
生成溯源树,研究论文发展脉络
Chat Paper
正在生成论文摘要