Few-Shot Learning of Diagnostic Rules for Neurodegenerative Diseases Using Inductive Logic Programming
INDUCTIVE LOGIC PROGRAMMING, ILP 2023(2023)
摘要
Traditional machine learning methods heavily rely on large amounts of labelled data for effective generalisation, posing a challenge in few-shot learning scenarios. In many real-world applications, acquiring large amounts of training data can be difficult or impossible. This paper presents an efficient and explainable method for few-shot learning from images using inductive logic programming (ILP). ILP utilises logical representations and reasoning to capture complex relationships and generalise from sparse data. We demonstrate the effectiveness of our proposed ILP-based approach through an experimental evaluation focused on detecting neurodegenerative diseases from fundus images. By extending our previous work on neurodegenerative disease detection, including Alzheimers disease, Parkinsons disease, and vascular dementia disease, we achieve improved explainability in identifying these diseases using fundus images collected from the UK Biobank dataset. The logical representation and reasoning inherent in ILP enhances the interpretability of the detection process. The results highlight the efficacy of ILP in few-shot learning scenarios, showcasing its remarkable generalisation performance compared to a range of other machine learning algorithms. This research contributes to the field of few-shot learning using ILP and paves the way for addressing challenging real-world problems.
更多查看译文
AI 理解论文
溯源树
样例
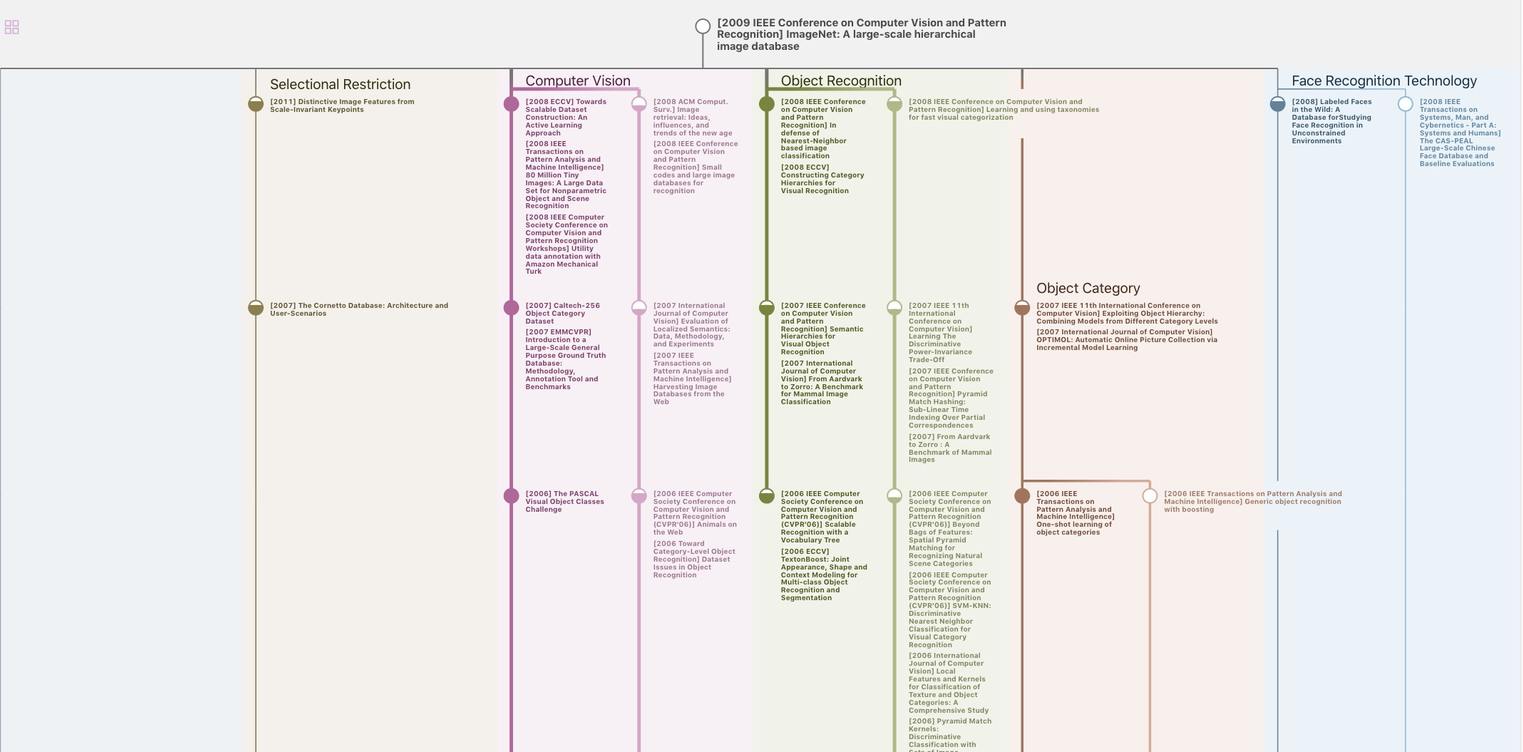
生成溯源树,研究论文发展脉络
Chat Paper
正在生成论文摘要