Lower Limb Activity Recognition Based on sEMG Using Stacked Weighted Random Forest
IEEE TRANSACTIONS ON NEURAL SYSTEMS AND REHABILITATION ENGINEERING(2024)
摘要
The existing surface electromyography-based pattern recognition system (sEMG-PRS) exhibits limited generalizability in practical applications. In this paper, we propose a stacked weighted random forest (SWRF) algorithm to enhance the long-term usability and user adaptability of sEMG-PRS. First, the weighted random forest (WRF) is proposed to address the issue of imbalanced performance in standard random forests (RF) caused by randomness in sampling and feature selection. Then, the stacking is employed to further enhance the generalizability of WRF. Specifically, RF is utilized as the base learner, while WRF serves as the meta-leaning layer algorithm. The SWRF is evaluated against classical classification algorithms in both online experiments and offline datasets. The offline experiments indicate that the SWRF achieves an average classification accuracy of 89.06%, outperforming RF, WRF, long short-term memory (LSTM), and support vector machine (SVM). The online experiments indicate that SWRF outperforms the aforementioned algorithms regarding long-term usability and user adaptability. We believe that our method has significant potential for practical application in sEMG-PRS.
更多查看译文
关键词
Biomedical signal,pattern recognition,lower limb activity,electromyography,random forest
AI 理解论文
溯源树
样例
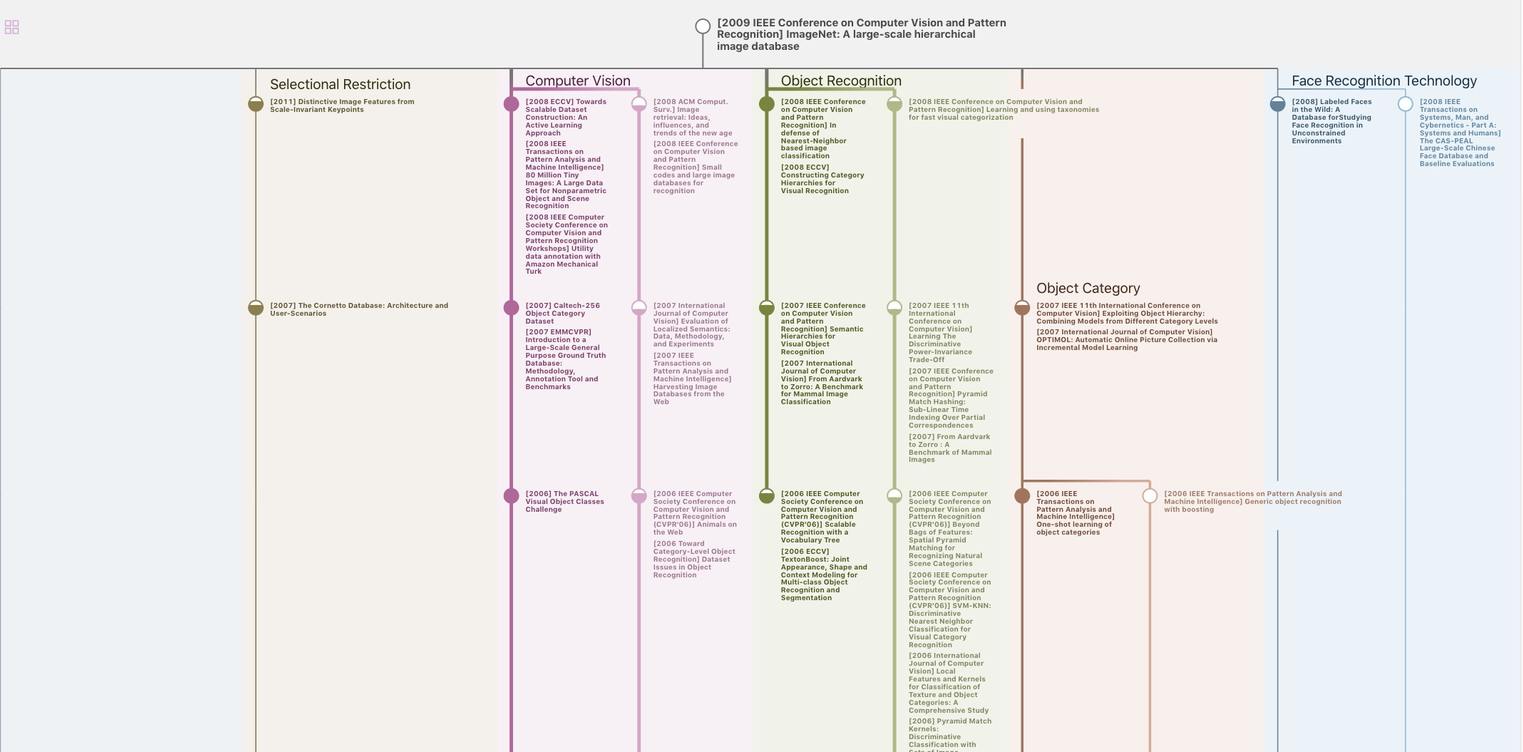
生成溯源树,研究论文发展脉络
Chat Paper
正在生成论文摘要