Towards Efficient On-Chip Learning for Spiking Neural Networks Accelerator with Surrogate Gradient.
2023 IEEE International Conference on Integrated Circuits, Technologies and Applications (ICTA)(2023)
摘要
Spiking Neural Networks (SNNs) have advantages in low power consumption, however, there is a problem of non-differentiability in the backpropagation (BP) of SNN. In this work, we propose a high-performance, low-cost SNN accelerator supports on-chip learning and utilizes a surrogate gradient (SG) for supervised learning, addressing the non-differentiability issue. The design is implemented on the VC707 FPGA, operating at a clock frequency of 115 MHz with a power consumption of 798 mW, achieving an accuracy of 95.49% on the MNIST dataset. The training and inference speeds are 1183 frame/s and 4163 frame/s. The training energy is 0.67 mJ/img and the inference energy is 0.21 mJ/img, reducing the energy consumption by 10–40 times compared to state-of-the-art (SOTA) unsupervised works.
更多查看译文
关键词
on-chip learning,surrogate gradient,spiking neural network
AI 理解论文
溯源树
样例
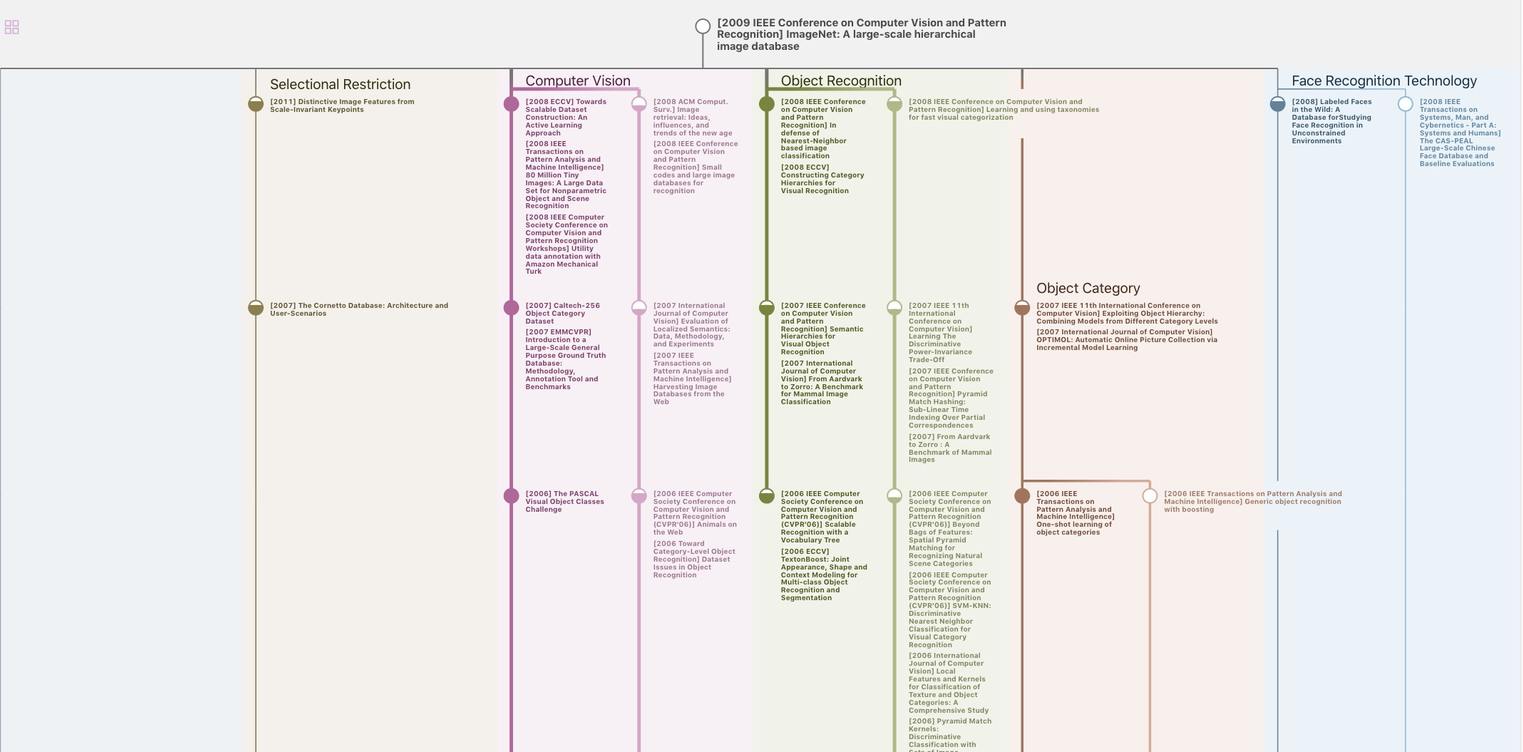
生成溯源树,研究论文发展脉络
Chat Paper
正在生成论文摘要