Toward Generalizable Robotic Dual-Arm Flipping Manipulation
IEEE TRANSACTIONS ON INDUSTRIAL ELECTRONICS(2024)
摘要
Robotic dual-arm manipulation often requires close cooperation between the arms. Dual-arm manipulation tasks are always difficult to program in advance, and then, executed autonomously by the robots. Learning from demonstration is an efficient programming method for robots that can transfer human skills to robots. However, conventional skill learning methods, e.g., dynamic movement primitives (DMPs) can only characterize the motion information of each dimension independently, and cannot take into account the relationship between multidimensional information. Participially, flipping manipulation is quite common in industry production lines, but it has not been well addressed yet to provide a robotics solution to this task. In this article, we propose an improved DMP model, called the object-level constrained DMP, which effectively preserves the association between multidimensional information. Similarly, we also propose an orientation generalization method for the flipping task. In addition, we show how to demonstrate the flipping task via a teleoperation system. Finally, experiments are performed on a Baxter robot to verify the effectiveness of the methods.
更多查看译文
关键词
Dynamic movement primitive (DMP),flipping task,learning from demonstration (LfD),skill generalization
AI 理解论文
溯源树
样例
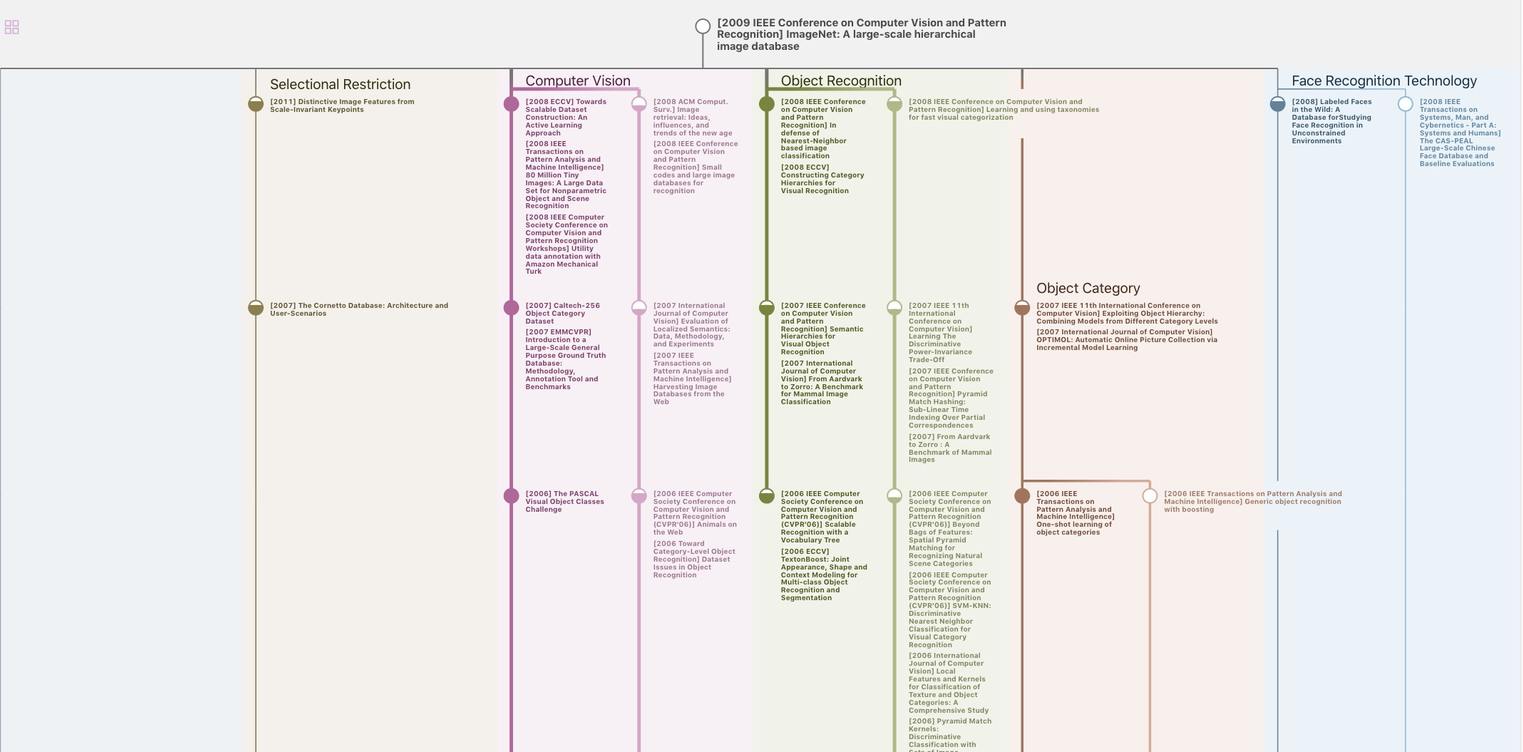
生成溯源树,研究论文发展脉络
Chat Paper
正在生成论文摘要