Conditional Data Synthesis with Deep Generative Models for Imbalanced Dataset Oversampling
2023 IEEE 35TH INTERNATIONAL CONFERENCE ON TOOLS WITH ARTIFICIAL INTELLIGENCE, ICTAI(2023)
摘要
The problem of data imbalance is defined as the uneven distribution of the training examples to the existing classes of a dataset. Among a wide variety of solutions, the oversampling techniques try to mitigate the problem by synthesizing artificial examples associated with the minority class. The huge success of Generative Adversarial Networks (GANs) rendered them an attractive choice for oversampling and numerous researchers proposed modifications of GANs for imbalanced datasets. Nevertheless, the existing models employ the entire minority class for sample generation, thus being vulnerable to outliers and noisy data instances. In addition, the majority of the relevant research concerns image classification tasks, leaving a large gap for research with tabular data. Finally, another powerful and popular generative model, the Variational Autoencoder (VAE) has been rather overlooked by the community in class imbalance solutions. In this paper we present SB-GAN and SB-VAE, two generative models that identify borderline and noisy samples before they are trained. In this manner SB-GAN and SB-VAE learn better class distributions that are not distorted by the existence of outliers. The experimental evaluation of SB-GAN and SB-VAE with 4 tabular datasets revealed a superior performance against 8 state-of-the-art oversampling techniques.
更多查看译文
关键词
imbalanced datasets,oversampling,generative models,GAN,VAE
AI 理解论文
溯源树
样例
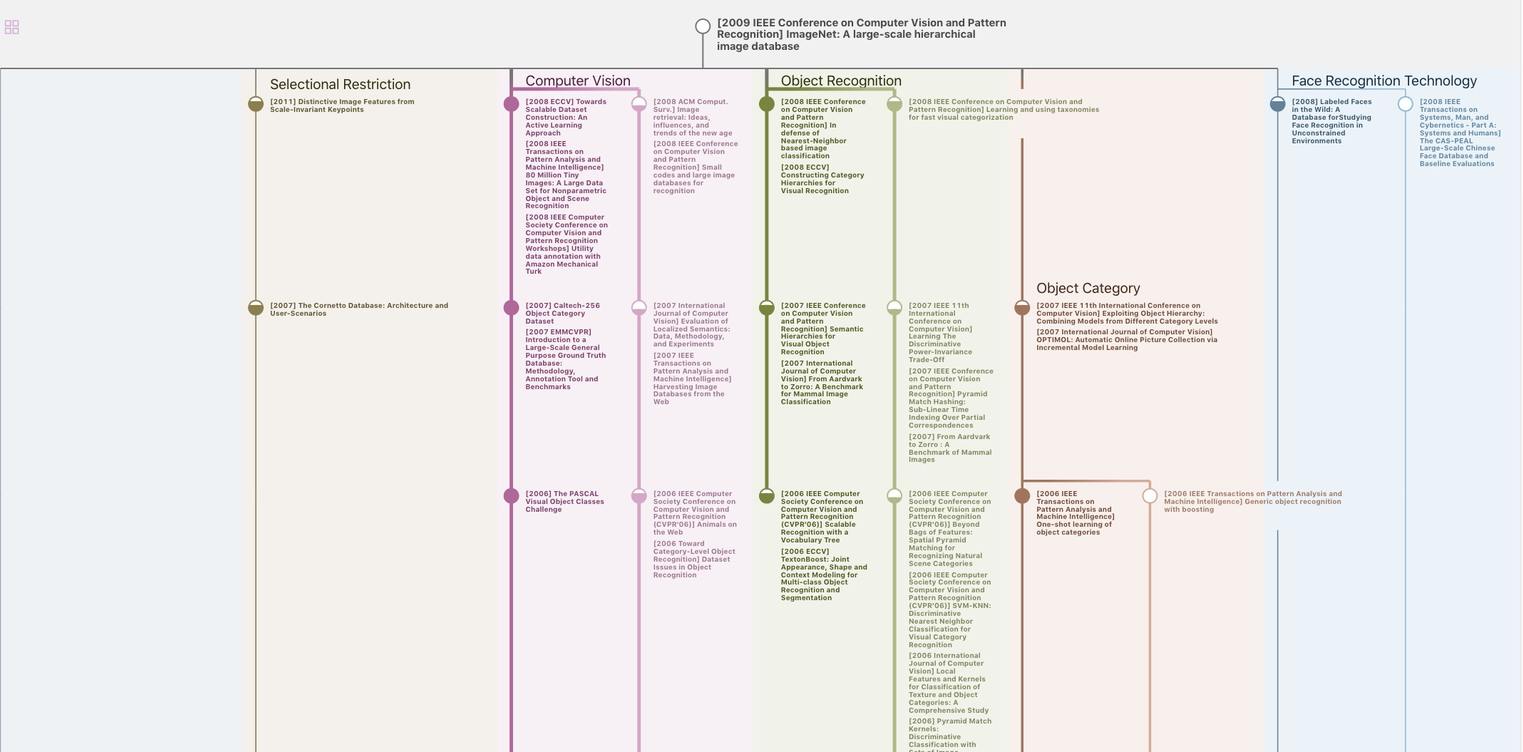
生成溯源树,研究论文发展脉络
Chat Paper
正在生成论文摘要