Association of Biomarker-Based Artificial Intelligence With Risk of Racial Bias in Retinal Images
JAMA Ophthalmology(2023)
摘要
Importance Although race is a social construct, it is associated with variations in skin and retinal pigmentation. Image-based medical artificial intelligence (AI) algorithms that use images of these organs have the potential to learn features associated with self-reported race (SRR), which increases the risk of racially biased performance in diagnostic tasks; understanding whether this information can be removed, without affecting the performance of AI algorithms, is critical in reducing the risk of racial bias in medical AI. Objective To evaluate whether converting color fundus photographs to retinal vessel maps (RVMs) of infants screened for retinopathy of prematurity (ROP) removes the risk for racial bias. Design, Setting, and Participants The retinal fundus images (RFIs) of neonates with parent-reported Black or White race were collected for this study. A u-net, a convolutional neural network (CNN) that provides precise segmentation for biomedical images, was used to segment the major arteries and veins in RFIs into grayscale RVMs, which were subsequently thresholded, binarized, and/or skeletonized. CNNs were trained with patients’ SRR labels on color RFIs, raw RVMs, and thresholded, binarized, or skeletonized RVMs. Study data were analyzed from July 1 to September 28, 2021. Main Outcomes and Measures Area under the precision-recall curve (AUC-PR) and area under the receiver operating characteristic curve (AUROC) at both the image and eye level for classification of SRR. Results A total of 4095 RFIs were collected from 245 neonates with parent-reported Black (94 [38.4%]; mean [SD] age, 27.2 [2.3] weeks; 55 majority sex [58.5%]) or White (151 [61.6%]; mean [SD] age, 27.6 [2.3] weeks, 80 majority sex [53.0%]) race. CNNs inferred SRR from RFIs nearly perfectly (image-level AUC-PR, 0.999; 95% CI, 0.999-1.000; infant-level AUC-PR, 1.000; 95% CI, 0.999-1.000). Raw RVMs were nearly as informative as color RFIs (image-level AUC-PR, 0.938; 95% CI, 0.926-0.950; infant-level AUC-PR, 0.995; 95% CI, 0.992-0.998). Ultimately, CNNs were able to learn whether RFIs or RVMs were from Black or White infants regardless of whether images contained color, vessel segmentation brightness differences were nullified, or vessel segmentation widths were uniform. Conclusions and Relevance Results of this diagnostic study suggest that it can be very challenging to remove information relevant to SRR from fundus photographs. As a result, AI algorithms trained on fundus photographs have the potential for biased performance in practice, even if based on biomarkers rather than raw images. Regardless of the methodology used for training AI, evaluating performance in relevant subpopulations is critical.
更多查看译文
关键词
racial bias,artificial intelligence,biomarker-based
AI 理解论文
溯源树
样例
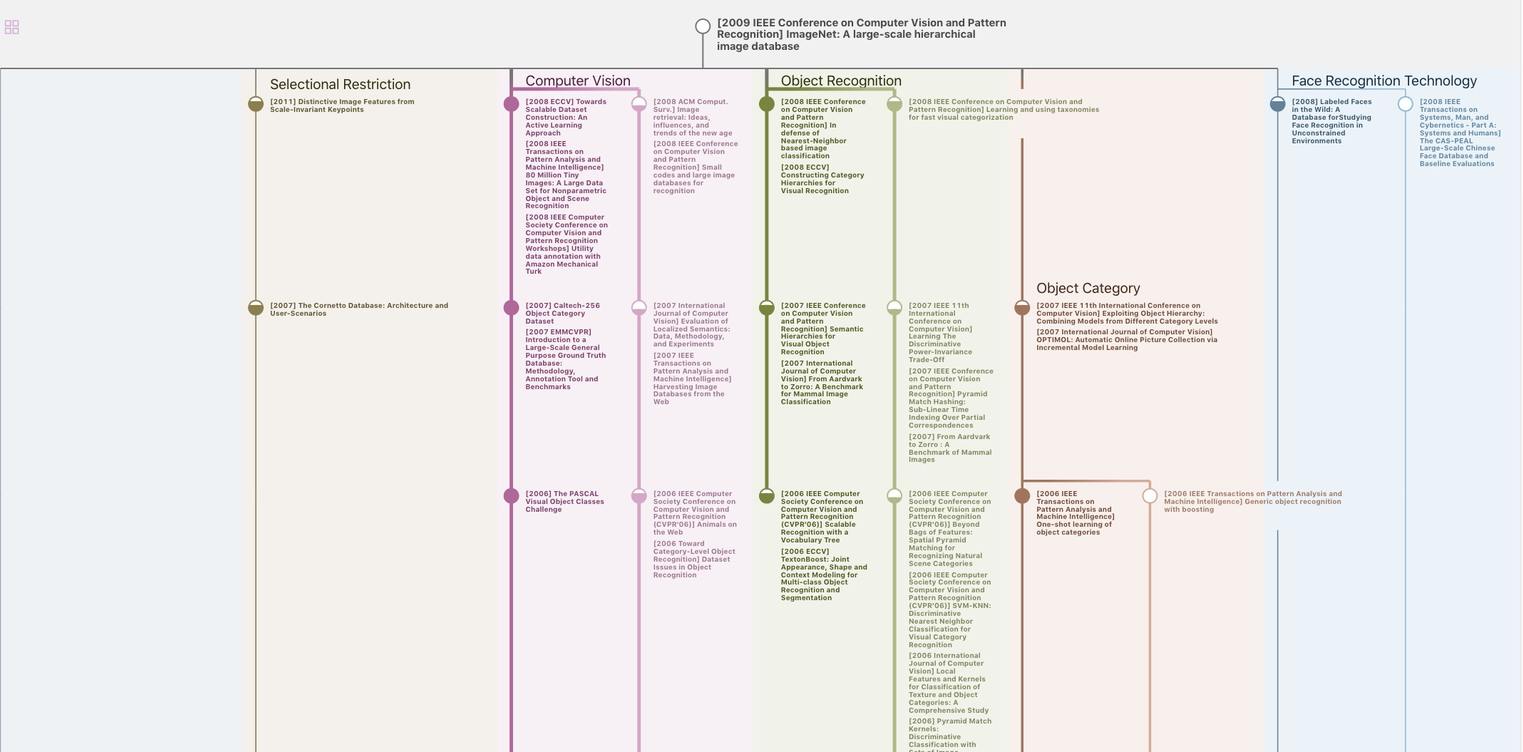
生成溯源树,研究论文发展脉络
Chat Paper
正在生成论文摘要