Spatio-Temporal Contrastive Self-Supervised Learning for POI-level Crowd Flow Inference
arXiv (Cornell University)(2023)
摘要
Accurate acquisition of crowd flow at Points of Interest (POIs) is pivotal for effective traffic management, public service, and urban planning. Despite this importance, due to the limitations of urban sensing techniques, the data quality from most sources is inadequate for monitoring crowd flow at each POI. This renders the inference of accurate crowd flow from low-quality data a critical and challenging task. The complexity is heightened by three key factors: 1) The scarcity and rarity of labeled data, 2) The intricate spatio-temporal dependencies among POIs, and 3) The myriad correlations between precise crowd flow and GPS reports. To address these challenges, we recast the crowd flow inference problem as a self-supervised attributed graph representation learning task and introduce a novel Contrastive Self-learning framework for Spatio-Temporal data (CSST). Our approach initiates with the construction of a spatial adjacency graph founded on the POIs and their respective distances. We then employ a contrastive learning technique to exploit large volumes of unlabeled spatio-temporal data. We adopt a swapped prediction approach to anticipate the representation of the target subgraph from similar instances. Following the pre-training phase, the model is fine-tuned with accurate crowd flow data. Our experiments, conducted on two real-world datasets, demonstrate that the CSST pre-trained on extensive noisy data consistently outperforms models trained from scratch.
更多查看译文
关键词
flow,learning,spatio-temporal,self-supervised,poi-level
AI 理解论文
溯源树
样例
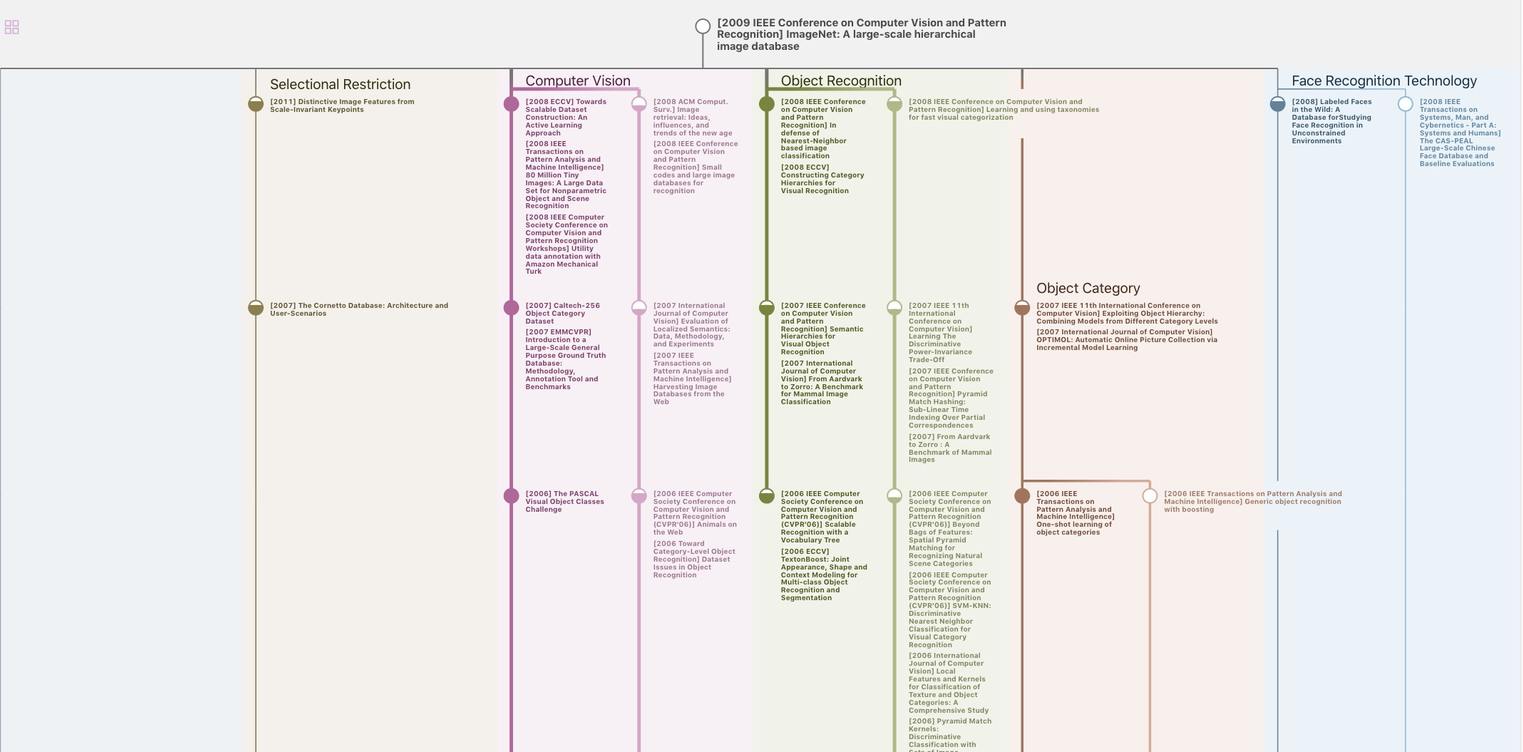
生成溯源树,研究论文发展脉络
Chat Paper
正在生成论文摘要