HiSTGNN: Hierarchical spatio-temporal graph neural network for weather forecasting
Information Sciences(2023)
摘要
Weather forecasting is an attractive yet challenging task due to its significant impacts on human life and the intricate nature of atmospheric motion. Deep learning-based techniques, utilizing abundant observations, have gained popularity in recent years. However, many existing methods mainly explore temporal patterns of meteorological variables while neglecting the interactions between variables across regions. To address this limitation, we propose HiSTGNN, a novel Hierarchical Spatio-temporal Graph Neural Network, which enables accurate predictions of multiple variables and stations over multiple time steps. HiSTGNN incorporates an adaptive graph learning module that constructs a self-learning hierarchical graph, comprising a global graph representing regions and a local graph capturing meteorological variables for each region. By leveraging graph convolution and gated temporal convolution with a dilated inception as the backbone, we effectively capture hidden spatial dependencies and diverse long-term meteorological trends. Additionally, we introduce dynamic interactive learning to facilitate bidirectional information between the two-level graphs. Experiments on three real-world meteorological datasets demonstrate the superiority of our proposed method compared to seven well-known baselines, including convolutional neural networks, recurrent neural networks, and spatio-temporal forecasting methods. Notably, HiSTGNN achieves remarkable improvements over the state-of-the-art weather forecasting method on the WD_BJ dataset, with a 4.25% decrease in MAE and a 5.34% decrease in RMSE.
更多查看译文
关键词
Weather forecasting,Spatio-temporal forecasting,Hierarchical graph neural network
AI 理解论文
溯源树
样例
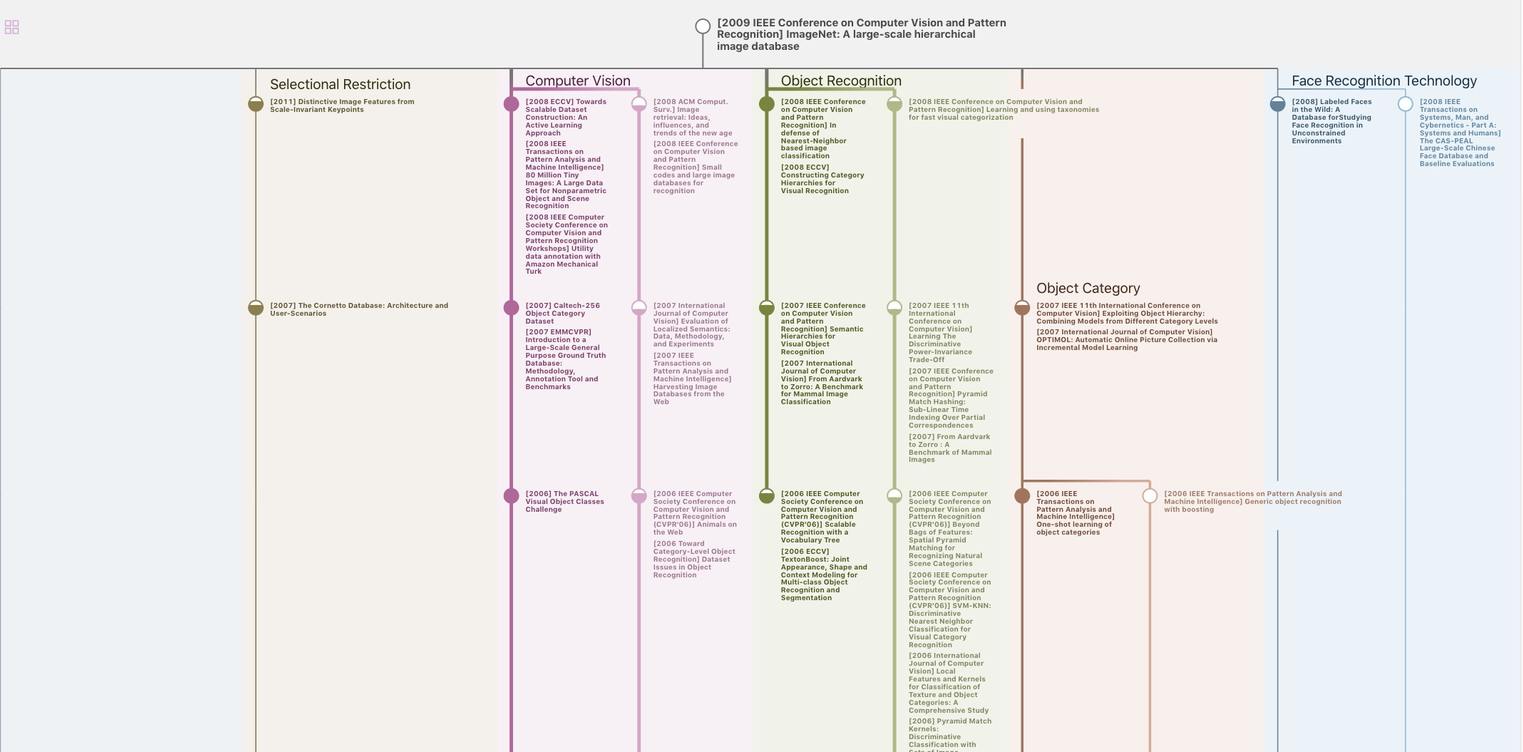
生成溯源树,研究论文发展脉络
Chat Paper
正在生成论文摘要