A Mathematical Morphological Network Fault Diagnosis Method for Rolling Bearings Based on Acoustic Array Signal
Applied Sciences(2023)
摘要
To extract valuable characteristic information from the acoustic radiation signal of rolling bearings, a novel mathematical morphological network (MMNet) is proposed. First, a mathematical morphological network layer is constructed by leveraging the advantages of a multi-scale enhanced top-hat morphological operator (MEAVGH) that can extract positive and negative pulses, which are then integrated into the deep learning network. Second, the input signal undergoes processing with different scale structural elements (SEs) to obtain multi-branch data. This is followed by channel attention and spatial attention mechanism-based weighting of the generated multi-branch data. Finally, the fused information is fed to the neural network to yield the final result. The experimental results demonstrate the efficacy of the proposed method in extracting fault feature information, achieving a fault classification accuracy of 98.56%. Furthermore, the algorithm exhibits robustness and high training efficiency. Comparative analysis reveals that the proposed method outperforms other approaches regarding cluster analysis, accuracy, recall rate, and computational efficiency. These findings further highlight the advantages of MMNet in acoustic signal-based fault diagnosis for rolling bearings.
更多查看译文
关键词
fault diagnosis,rolling bearings,mathematical morphological,deep learning
AI 理解论文
溯源树
样例
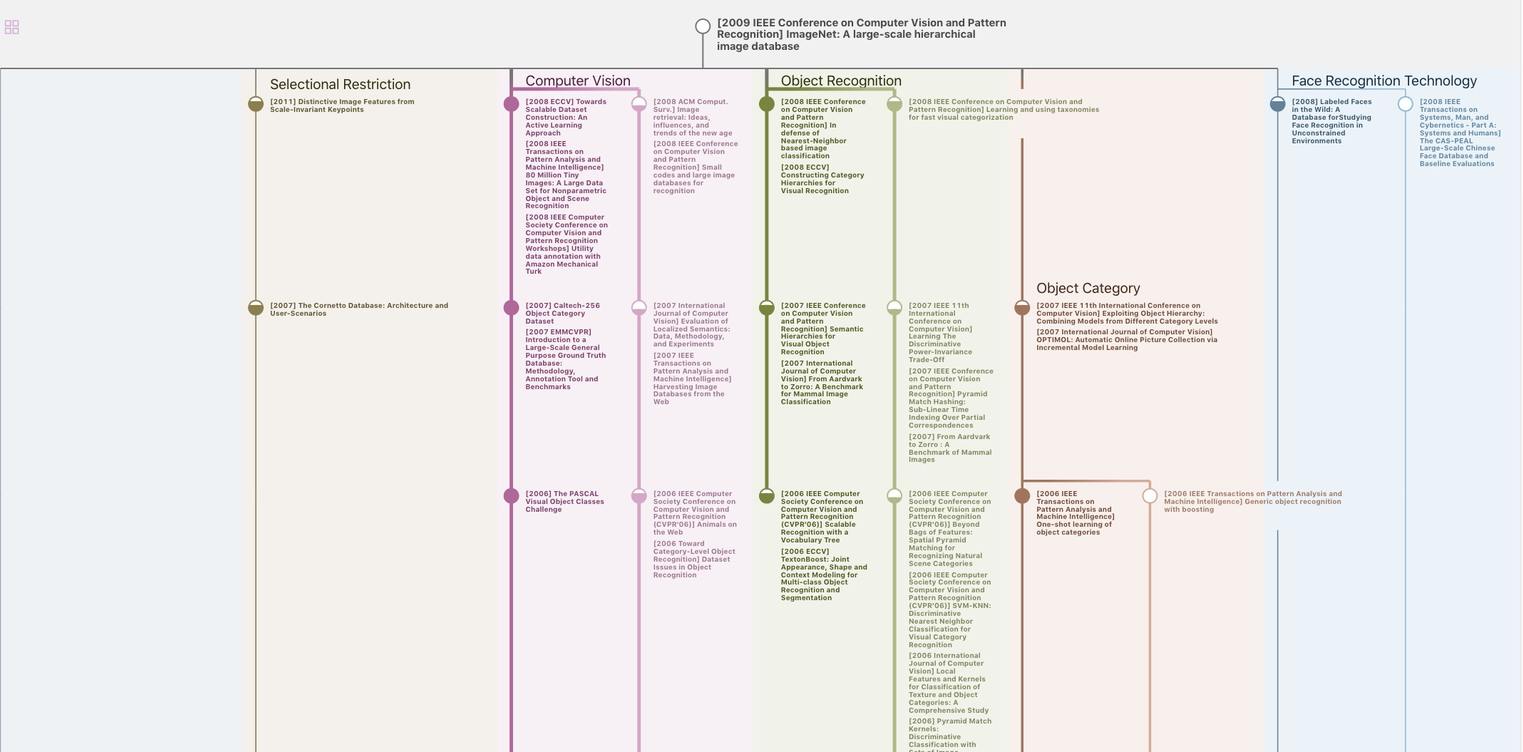
生成溯源树,研究论文发展脉络
Chat Paper
正在生成论文摘要