Phenotype Execution and Modelling Architecture (PhEMA) to support disease surveillance and real-world evidence studies: English sentinel network evaluation
crossref(2023)
摘要
AbstractObjectiveTo evaluate Phenotype Execution and Modelling Architecture (PhEMA), to express sharable phenotypes using Clinical Query Language (CQL) and intensional SNOMED CT Fast Healthcare Interoperability Resources (FHIR) valuesets, for exemplar chronic disease, sociodemographic risk factor and surveillance phenotypes.MethodWe curated three phenotypes: Type 2 diabetes (T2DM), excessive alcohol use and incident influenza-like illness (ILI) using CQL to define clinical and administrative logic. We defined our phenotypes with valuesets, using SNOMED’s hierarchy and expression constraint language (ECL), and CQL, combining valuesets and adding temporal elements where needed. We compared the count of cases found using PhEMA with our existing approach using convenience datasets.ResultsThe T2DM phenotype could be defined as two intensionally defined SNOMED valuesets and a CQL script. It increased the prevalence from 7.2% to 7.3%. Excess alcohol phenotype was defined by valuesets that added qualitative clinical terms to the quantitative conceptual definitions we currently use; this change increased prevalence by 58%, from 1.2% to 1.9%. We created an ILI valueset with SNOMED concepts, adding a temporal element using CQL to differentiate new episodes. This increased the weekly incidence in our convenience sample (weeks 26 to 38) from 0.95 cases to 1.11 cases per 100,000 people.ConclusionsPhenotypes for surveillance and research can be described fully and comprehensibly using CQL and intensional FHIR valuesets. Our use case phenotypes identified a greater number of cases, whilst anticipated from excessive alcohol this was not for our other variable. This may have been due to our use of SNOMED CT hierarchy.
更多查看译文
AI 理解论文
溯源树
样例
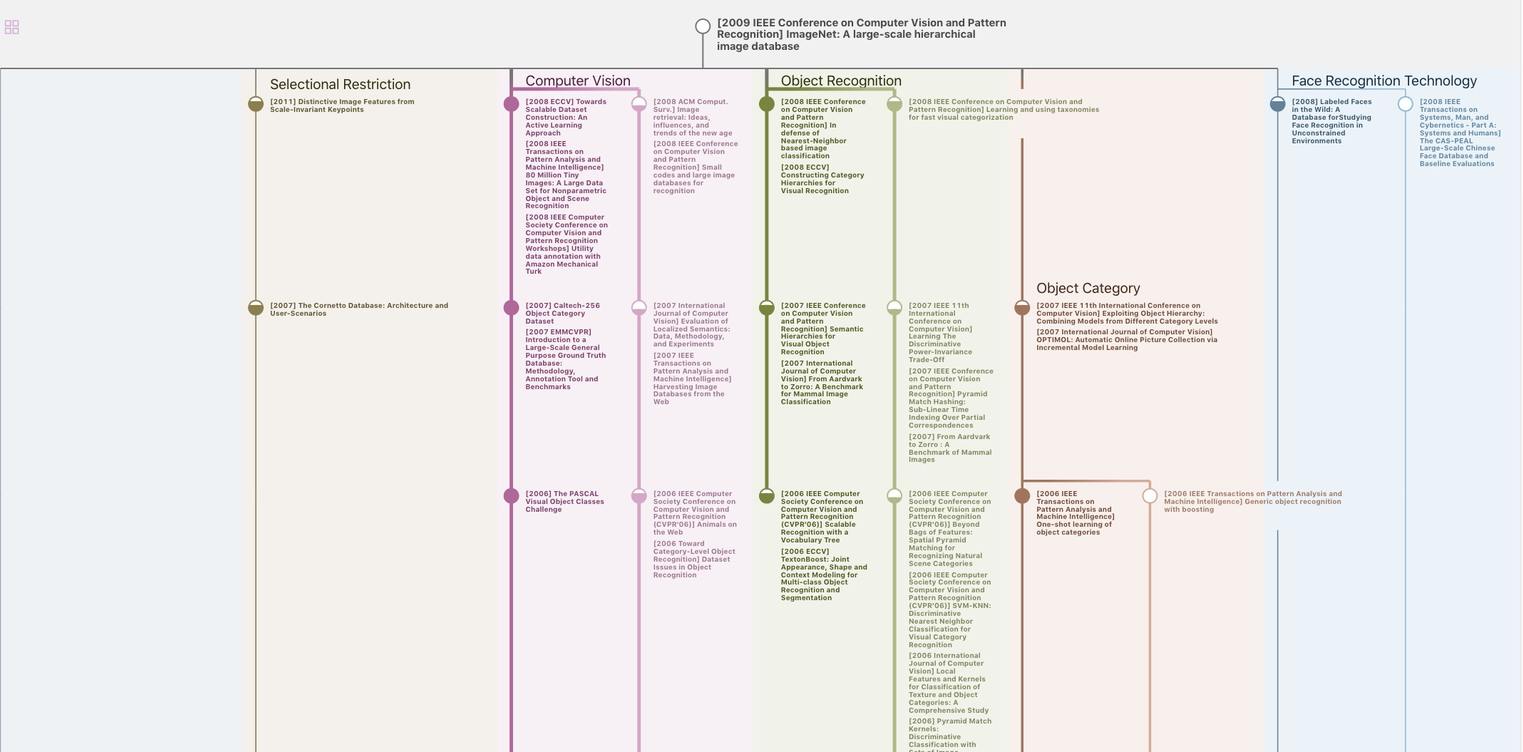
生成溯源树,研究论文发展脉络
Chat Paper
正在生成论文摘要