Neuromorphic Incremental on-chip Learning with Hebbian Weight Consolidation
CoRR(2023)
摘要
As next-generation implantable brain-machine interfaces become pervasive on
edge device, incrementally learning new tasks in bio-plasticity ways is
urgently demanded for Neuromorphic chips. Due to the inherent characteristics
of its structure, spiking neural networks are naturally well-suited for
BMI-chips. Here we propose Hebbian Weight Consolidation, as well as an on-chip
learning framework. HWC selectively masks synapse modifications for previous
tasks, retaining them to store new knowledge from subsequent tasks while
preserving the old knowledge. Leveraging the bio-plasticity of dendritic
spines, the intrinsic self-organizing nature of Hebbian Weight Consolidation
aligns naturally with the incremental learning paradigm, facilitating robust
learning outcomes. By reading out spikes layer by layer and performing
back-propagation on the external micro-controller unit, MLoC can efficiently
accomplish on-chip learning. Experiments show that our HWC algorithm up to
23.19% outperforms lower bound that without incremental learning algorithm,
particularly in more challenging monkey behavior decoding scenarios. Taking
into account on-chip computing on Synsense Speck 2e chip, our proposed
algorithm exhibits an improvement of 11.06%. This study demonstrates the
feasibility of employing incremental learning for high-performance neural
signal decoding in next-generation brain-machine interfaces.
更多查看译文
AI 理解论文
溯源树
样例
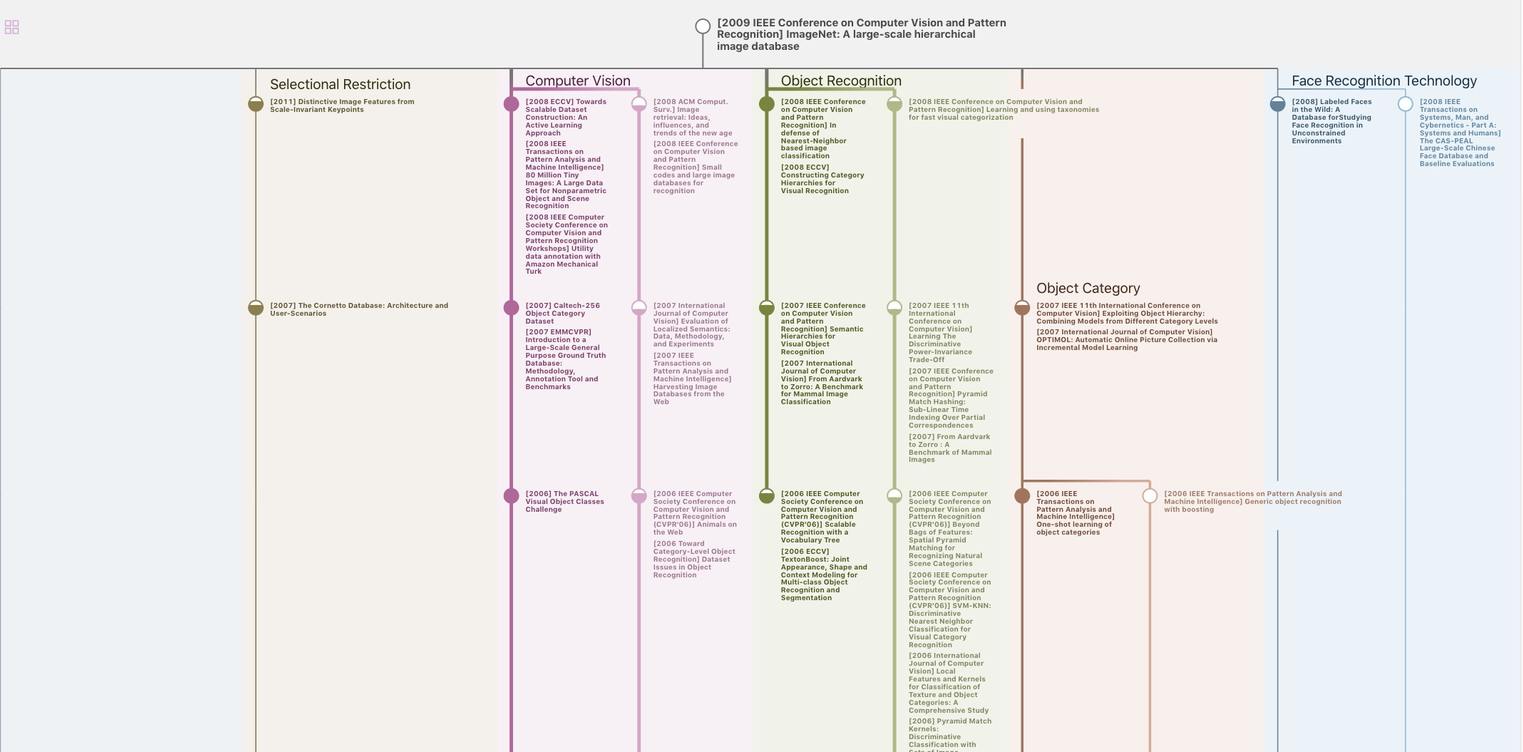
生成溯源树,研究论文发展脉络
Chat Paper
正在生成论文摘要