Feature Space Augmentation and Old Class Space Preservation for Class Incremental Learning
2023 42nd Chinese Control Conference (CCC)(2023)
摘要
The ability of continuously learning new knowledge without forgetting old ones is crucial to adapt to an ever-changing world. This scenario becomes more challenging when the previous data are not available. Current class incremental learning (CIL) approaches tend to incorporate new classes with backward compatibility, i.e., maintaining discriminability of old ones. By contrast, we focus on the extensibility and compatibility for future new classes in the early stage. Our proposed method achieves this by augmenting feature space. In detail, a large number of pseudo-new classes are generated via real example mixture and then train the initial spatially augmented model using pseudo-new classes and the base classes. Besides, considering the fact that it is impossible to maintain backward compatibility with only one prototype for each old class, the updated model also needs to preserve old class space in incremental stages. We employ a certain perturbation to the old class prototypes to effectively avoid old class space from being over-squeezed by the samples of new classes. The experiments on CIFAR-100 and ImageNet-Subset (100 classes) demonstrate that our method substantially reduces the overlap of old and new classes, outperforming state-of-the-art various baselines.
更多查看译文
关键词
Class incremental learning,Catastrophic forgetting,Feature space augmentation,Pseudo-new classes,Old class space preservation
AI 理解论文
溯源树
样例
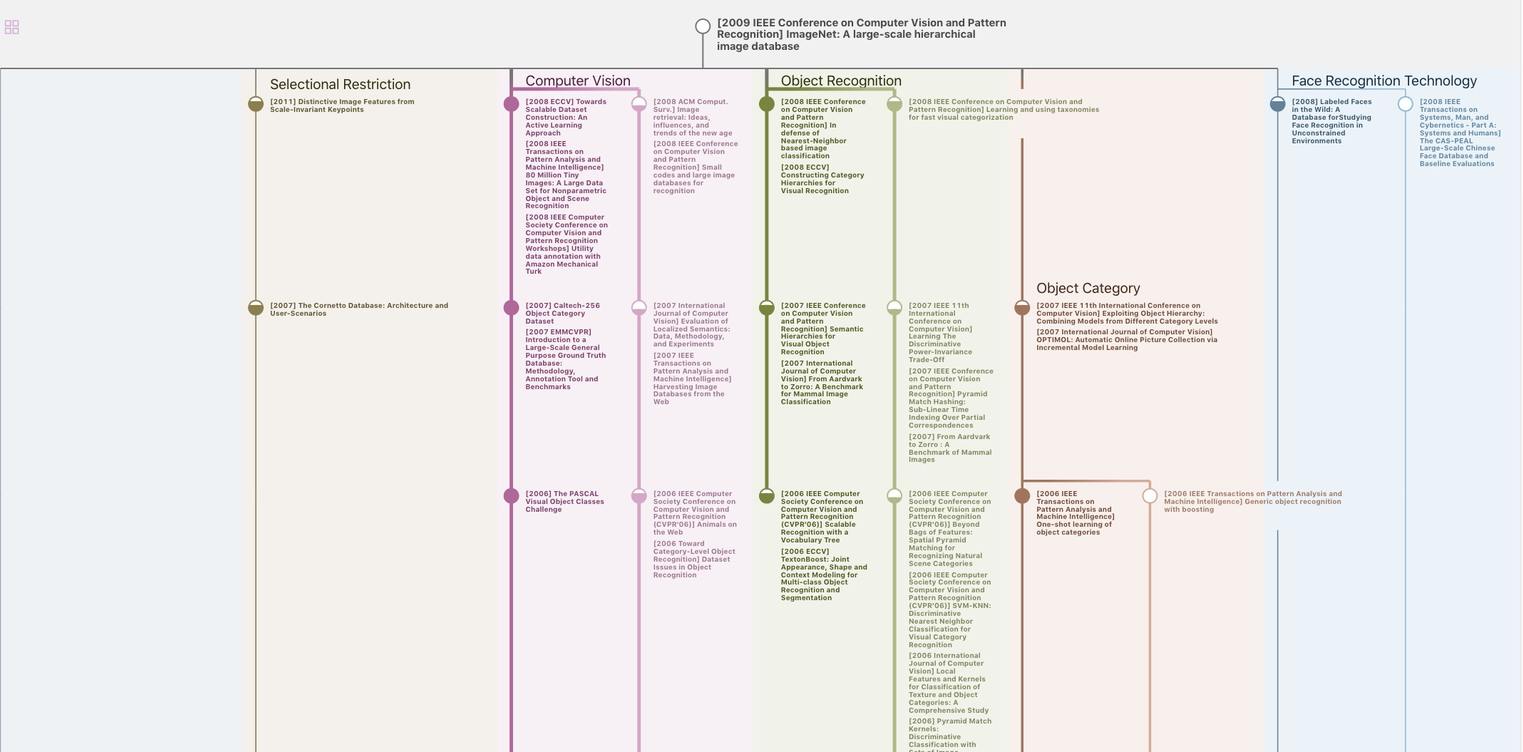
生成溯源树,研究论文发展脉络
Chat Paper
正在生成论文摘要