Automatically Discovering Novel Visual Categories With Adaptive Prototype Learning
IEEE TRANSACTIONS ON PATTERN ANALYSIS AND MACHINE INTELLIGENCE(2024)
摘要
This article targets the task of novel category discovery (NCD), which aims to discover unknown categories when a certain number of classes are already known. The NCD task is challenging due to its closeness to real-world scenarios, where we have only encountered some partial classes and corresponding images. Unlike previous approaches to NCD, we propose a novel adaptive prototype learning method that leverages prototypes to emphasize category discrimination and alleviate the issue of missing annotations for novel classes. Concretely, the proposed method consists of two main stages: prototypical representation learning and prototypical self-training. In the first stage, we develop a robust feature extractor that could effectively handle images from both base and novel categories. This ability of instance and category discrimination of the feature extractor is boosted by self-supervised learning and adaptive prototypes. In the second stage, we utilize the prototypes again to rectify offline pseudo labels and train a final parametric classifier for category clustering. We conduct extensive experiments on four benchmark datasets, demonstrating our method's effectiveness and robustness with state-of-the-art performance.
更多查看译文
关键词
Image recognition,novel category discovery,transfer learning
AI 理解论文
溯源树
样例
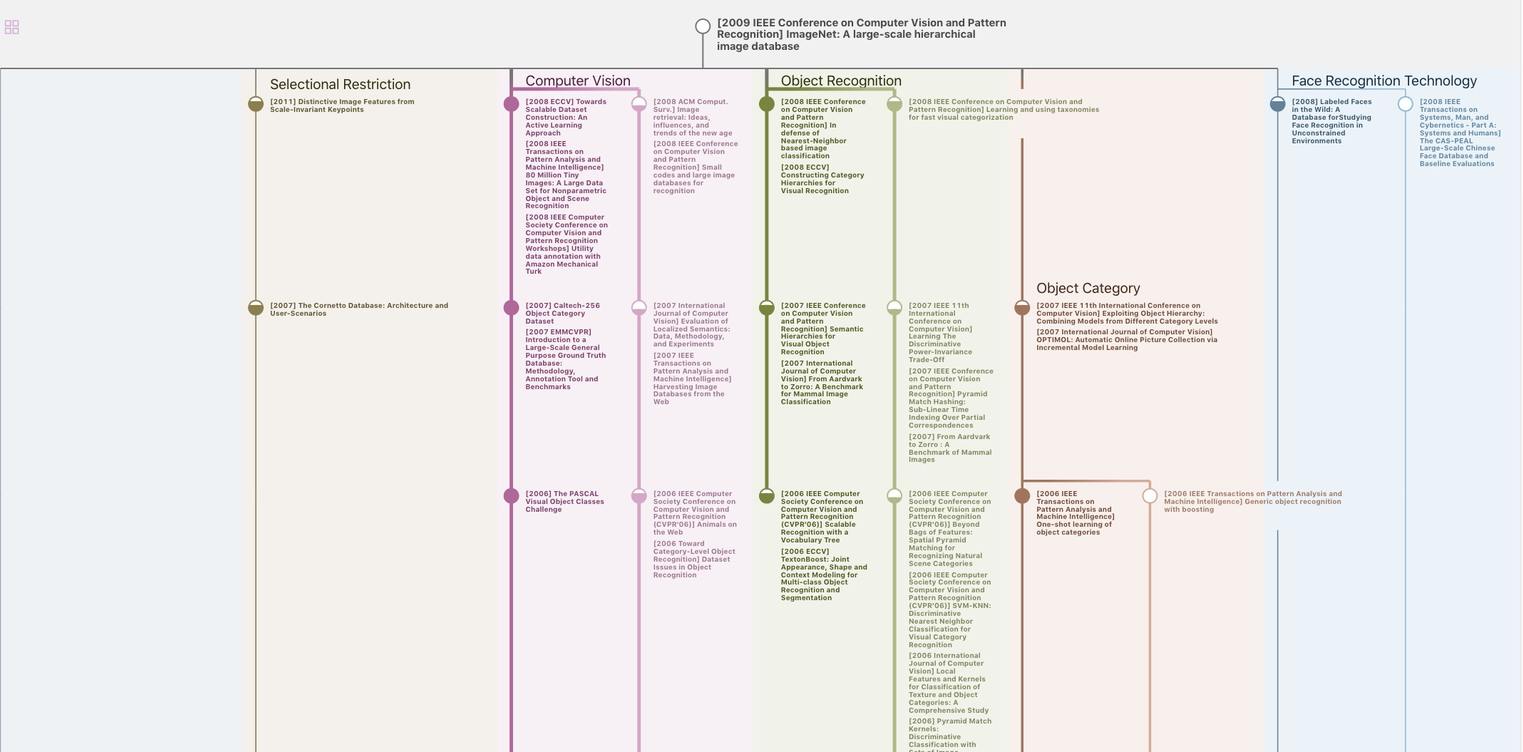
生成溯源树,研究论文发展脉络
Chat Paper
正在生成论文摘要