X-GRL: An Empirical Assessment of Explainable GNN-DRL in B5G/6G Networks
2023 IEEE Conference on Network Function Virtualization and Software Defined Networks (NFV-SDN)(2023)
摘要
The rapid development of artificial intelligence (AI) techniques has triggered a revolution in beyond fifth-generation (B5G) and upcoming sixth-generation (6G) mobile networks. Despite these advances, efficient resource allocation in dynamic and complex networks remains a major challenge. This paper presents an experimental implementation of deep reinforcement learning (DRL) enhanced with graph neural networks (GNNs) on a real 5G testbed. The method addresses the explainability of GNNs by evaluating the importance of each edge in determining the model's output. The custom sampling functions feed the data into the proposed GNN-driven Monte Carlo policy gradient (REINFORCE) agent to optimize the gNodeB (gNB) radio resources according to the specific traffic demands. The demo demonstrates real-time visualization of network parameters and superior performance compared to benchmarks.
更多查看译文
关键词
B5G/6G,AI/ML,XAI,GNN-DRL,Resource Allocation
AI 理解论文
溯源树
样例
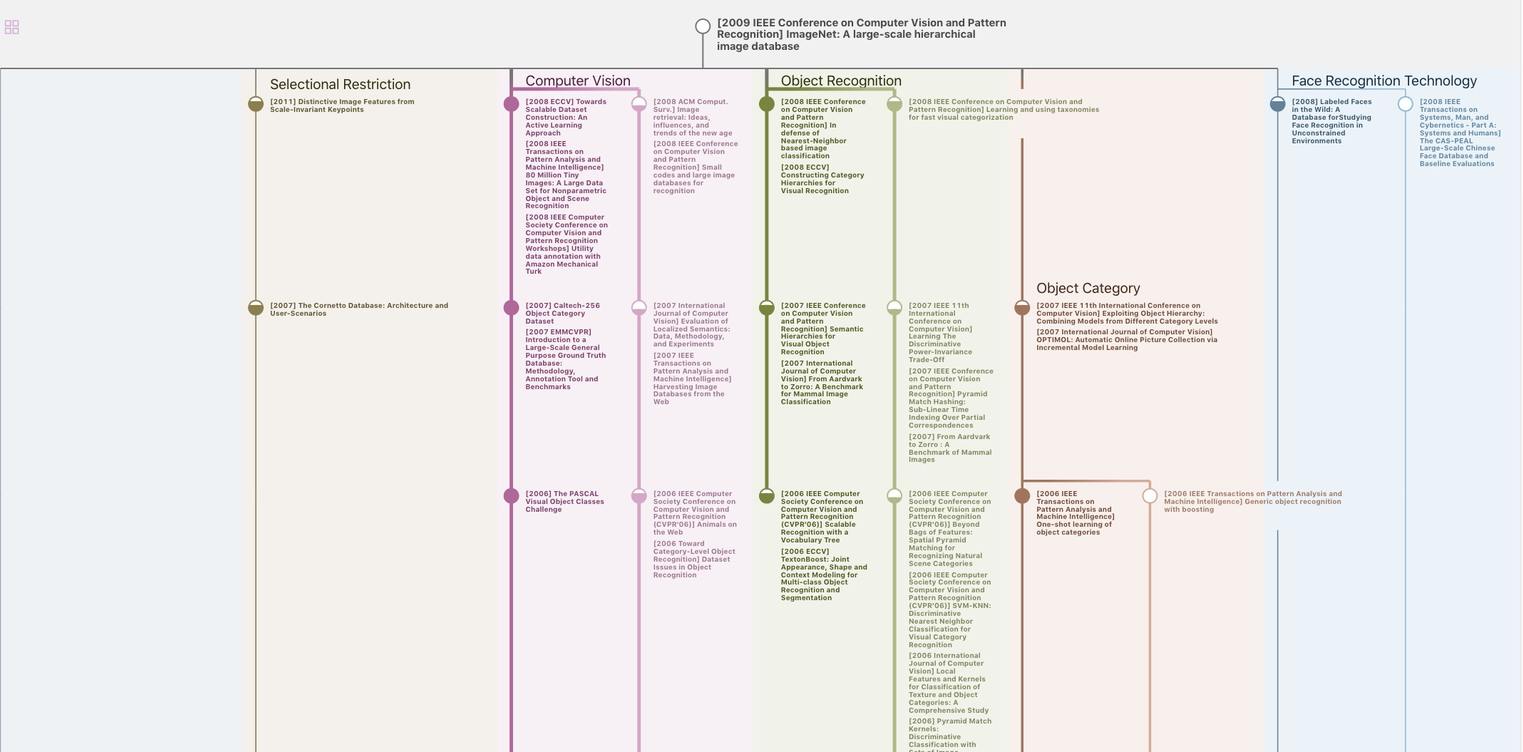
生成溯源树,研究论文发展脉络
Chat Paper
正在生成论文摘要