FPGA Accelerating Multi-Source Transfer Learning with GAT for Bioactivities of Ligands Targeting Orphan G Protein-Coupled Receptors
2023 33rd International Conference on Field-Programmable Logic and Applications (FPL)(2023)
摘要
Machine learning has been used extensively in the bioactivity value (BAV) prediction of G Protein-Coupled Receptors (GPCR) targeting ligands. However, the performance of over 140 types of GPCR endogenous ligands, also called orphan GPCRs (oGPCRs), is still unsatisfactory due to the limited sample size. Also, current works are far from meeting the demand for fast inference time and energy efficiency. We propose the Multi-Source Transfer-Graph Attention Network (MSTL-GAT), as well as its FPGA-based accelerator. Firstly, we make use of the three ideal data sources for transfer learning, oGPCRs, experimentally validated GPCRs, and invalidated GPCRs similar to the former one. Secondly, we transform GPCRs from the SIMLEs format to graphics as the input of GAT to improve prediction accuracy. Moreover, we propose an FPGA-based accelerator tailored for the inference phase of MSTL-GAT. Finally, our experimental results show that MSTL-GAT remarkably improves the prediction of GPCRs ligand activity value compared with previous studies. On average, the two evaluation indexes we adopt, R2 and RMSE, improve by 34.76% and 13.16%, respectively. The proposed FPGA accelerator achieves 2.7× and 4.7× speedup, 29.7×, and 3.6× energy efficiency compared with works on GPU implementation and the state-of-the-art FPGA accelerator, respectively.
更多查看译文
关键词
FPGA, Accelerator, Graph Attention Network, Bioactivitie, G Protein-Coupled Receptors
AI 理解论文
溯源树
样例
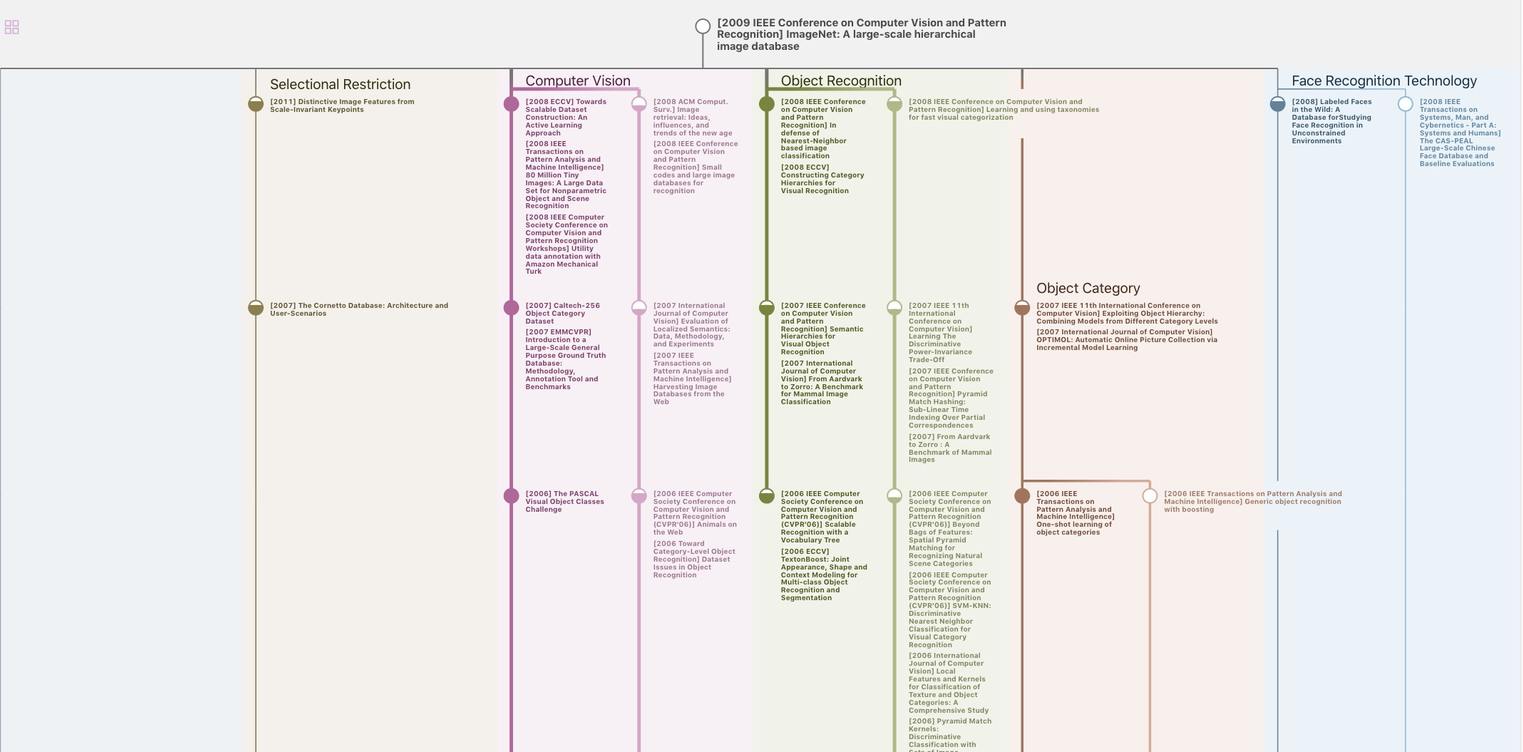
生成溯源树,研究论文发展脉络
Chat Paper
正在生成论文摘要