ObVi-SLAM: Long-Term Object-Visual SLAM
IEEE ROBOTICS AND AUTOMATION LETTERS(2024)
摘要
Robots responsible for tasks over long time scales must be able to localize consistently and scalably amid geometric, viewpoint, and appearance changes. Existing visual SLAM approaches rely on low-level feature descriptors that are not robust to such environmental changes and result in large map sizes that scale poorly over long-term deployments. In contrast, object detections are robust to environmental variations and lead to more compact representations, but most object-based SLAM systems target short-term indoor deployments with close objects. In this letter, we introduce ObVi-SLAM to overcome these challenges by leveraging the best of both approaches. ObVi-SLAM uses low-level visual features for high-quality short-term visual odometry; and to ensure global, long-term consistency, ObVi-SLAM builds an uncertainty-aware long-term map of persistent objects and updates it after every deployment. By evaluating ObVi-SLAM on data from 16 deployment sessions spanning different weather and lighting conditions, we empirically show that ObVi-SLAM generates accurate localization estimates consistent over long time scales in spite of varying appearance conditions.
更多查看译文
关键词
Simultaneous localization and mapping,Visualization,Feature extraction,Trajectory,Optimization,Robots,Ellipsoids,SLAM,localization,semantic scene understanding
AI 理解论文
溯源树
样例
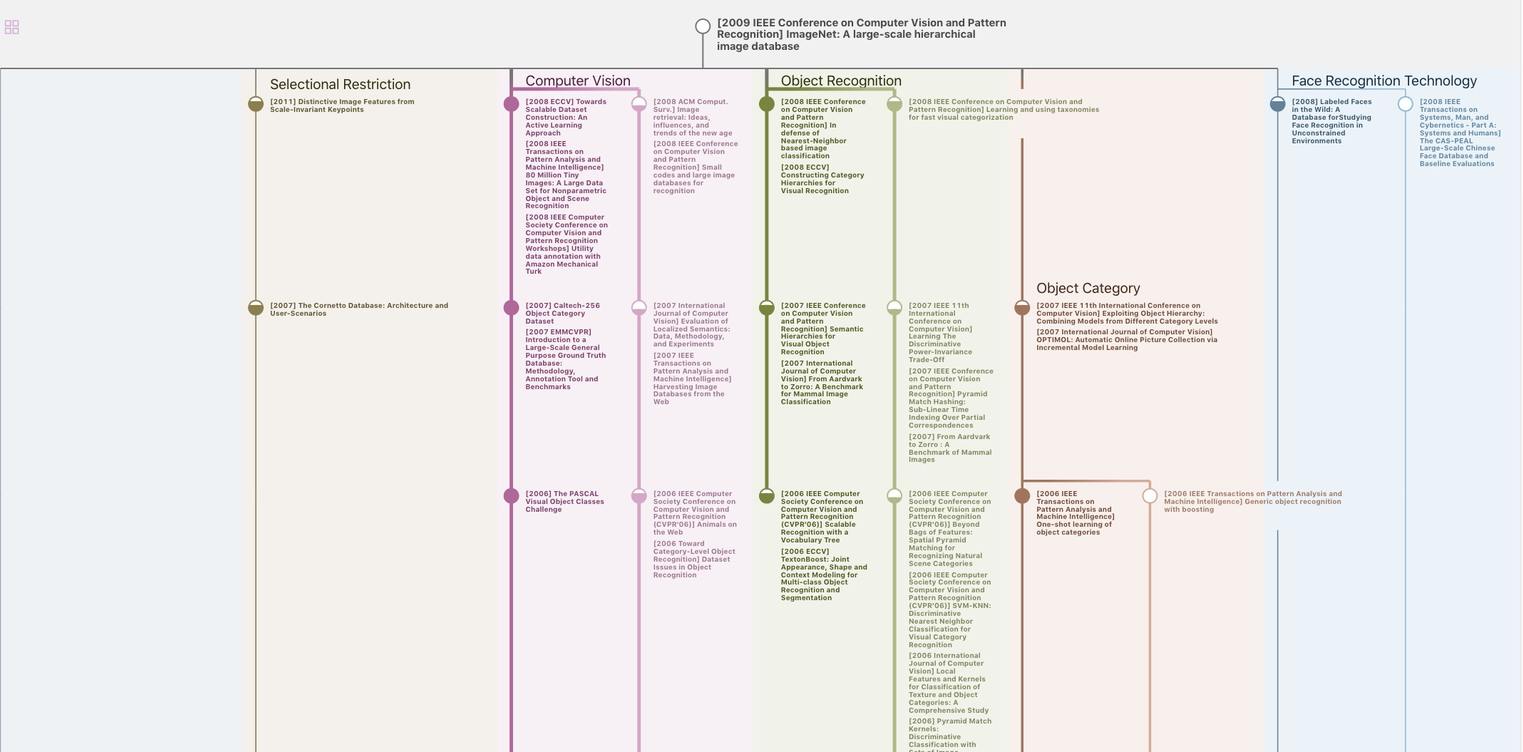
生成溯源树,研究论文发展脉络
Chat Paper
正在生成论文摘要