Enhancing 5G Radio Planning with Graph Representations and Deep Learning
PROCEEDINGS OF THE 2023 3RD ACM WORKSHOP ON 5G AND BEYOND NETWORK MEASUREMENTS, MODELING, AND USE CASES, 5G-MEMU 2023(2023)
摘要
The roll out of new mobile network generations poses hard challenges due to various factors such as cost-benefit tradeoffs, existing infrastructure, and new technology aspects. In particular, one of the main challenges for the 5G deployment lies in optimal 5G radio coverage while accounting for diverse service performance metrics. This paper introduces a Deep Learning-based approach to assist in 5G radio planning by utilizing data from previous-generation cells. Our solution relies on a custom graph representation to leverage the information available from existing cells, and employs a Graph Neural Network (GNN) model to process such data efficiently. In our evaluation, we test its potential to model the transition from 4G to 5G NSA using real-world data from a UK mobile network operator. The experimental results show that our solution achieves high accuracy in predicting key performance indicators in new 5G cells, with a Mean Absolute Percentage Error (MAPE) <17% when evaluated on samples from the same area where it was trained. Moreover, we test its generalization capability over various geographical areas not included in the training, achieving a MAPE <19%. This suggests beneficial properties for achieving robust solutions applicable to 5G planning in new areas without the need of retraining.
更多查看译文
关键词
Mobile Networks,Deep Learning,Graph Representation,Network Modeling
AI 理解论文
溯源树
样例
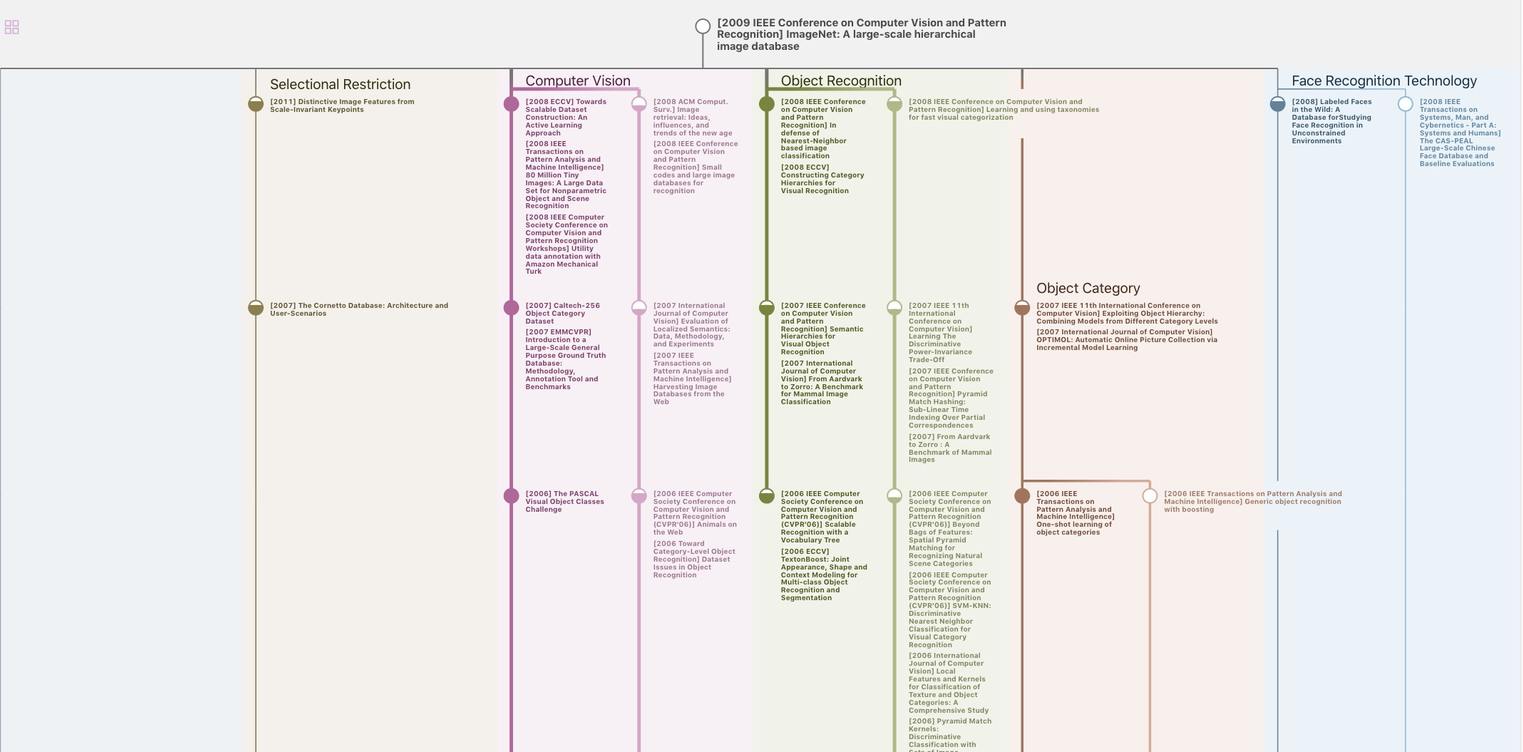
生成溯源树,研究论文发展脉络
Chat Paper
正在生成论文摘要