Robust Convex Model Predictive Control for Quadruped Locomotion Under Uncertainties
IEEE TRANSACTIONS ON ROBOTICS(2023)
摘要
This article considers quadruped locomotion control in the presence of uncertainties. Two types of structured uncertainties are considered, namely, uncertain friction constraints and uncertain model dynamics. Then, a min-max optimization model is formulated based on robust optimization, and a robust min-max model predictive controller is proposed by recurrently solving the optimization model. We prove that the min-max optimization model is equivalent to a convex quadratic constrained quadratic program by exploiting the structure of uncertainties. Moreover, a two-stage optimization algorithm is proposed to solve the optimization problem efficiently, allowing for the deployment of the controller onto the real robot. The results show that the proposed optimization algorithm can improve solving frequency by
$\sim$
11× compared with Gurobi. The proposed controller is able to stabilize quadruped locomotion in challenging scenarios where the uncertainties are caused by significant disturbances and unknown environments.
更多查看译文
关键词
quadruped locomotion,predictive control
AI 理解论文
溯源树
样例
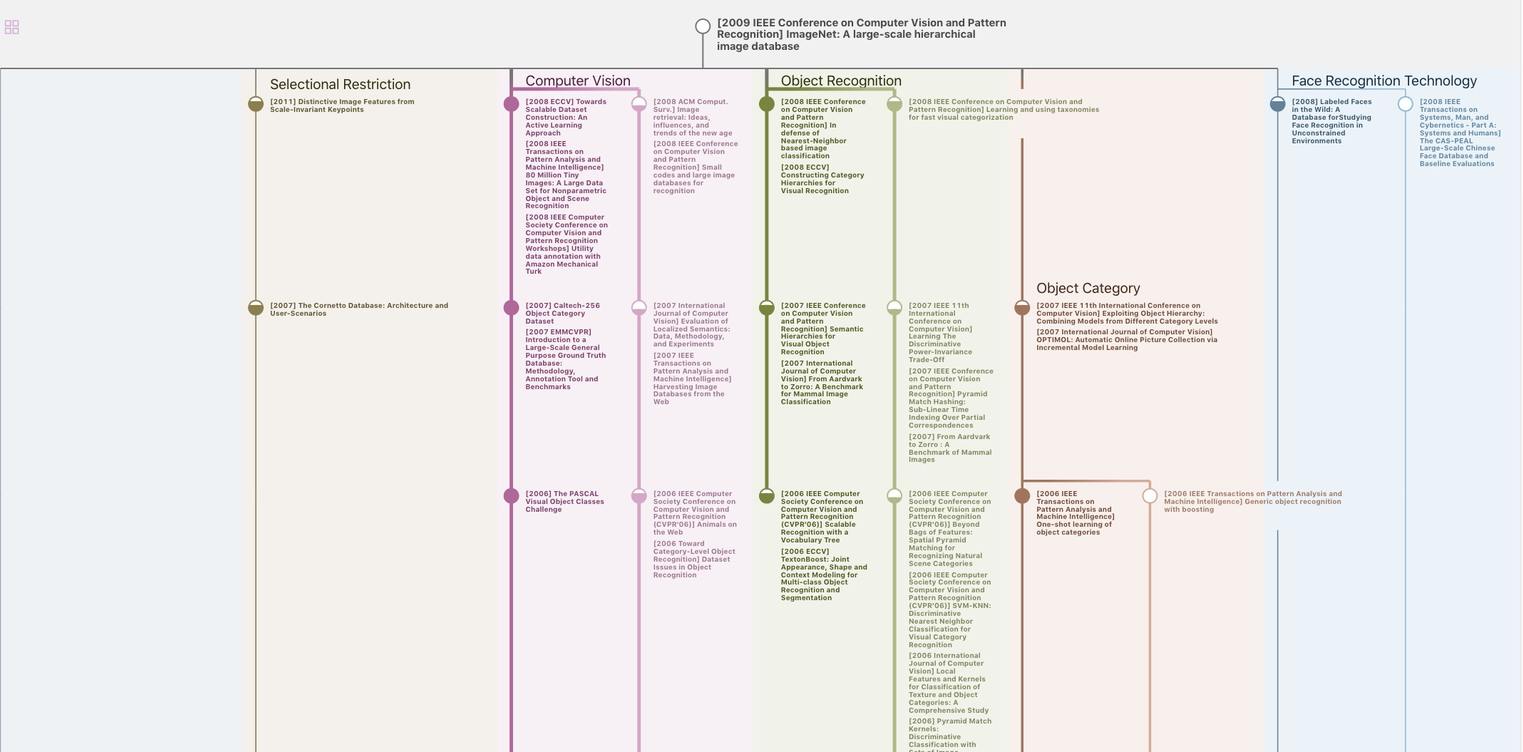
生成溯源树,研究论文发展脉络
Chat Paper
正在生成论文摘要