Budgeting and predicting pattern defects using edge placement error and machine learning
2023 7TH IEEE ELECTRON DEVICES TECHNOLOGY & MANUFACTURING CONFERENCE, EDTM(2023)
摘要
Edge Placement Error (EPE) is a metric based on extreme value statistics that combines all the variations both in CD and Overlay domains. This is needed to characterize and ultimately monitor process performance and yield. In this paper, a new metrology methodology, all-in-one (or AIO), is developed to measure multiple process indexes such as CD, overlay, and EPE in one image. The robustness of the AIO metrology is confirmed by comparing AIO indexes and unit indexes measured separately. Using the data collected by AIO metrology, process budget characterization and wafermap analysis of the edge placement error (EPE) are performed to understand the cause of pattern defects for the 1x nm DRAM device at SK hynix. In addition, defect prediction is studied using EPE data in multiple layers and machine learning. As a result, we demonstrate EPE measurement, budgeting, and trend monitoring are useful to monitor pattern defects. The root-causes of such pattern defects are confirmed by the help of decent machine learning algorithm and process domain knowledge.
更多查看译文
关键词
EPE,Vision ML,ML,Pattern Defect
AI 理解论文
溯源树
样例
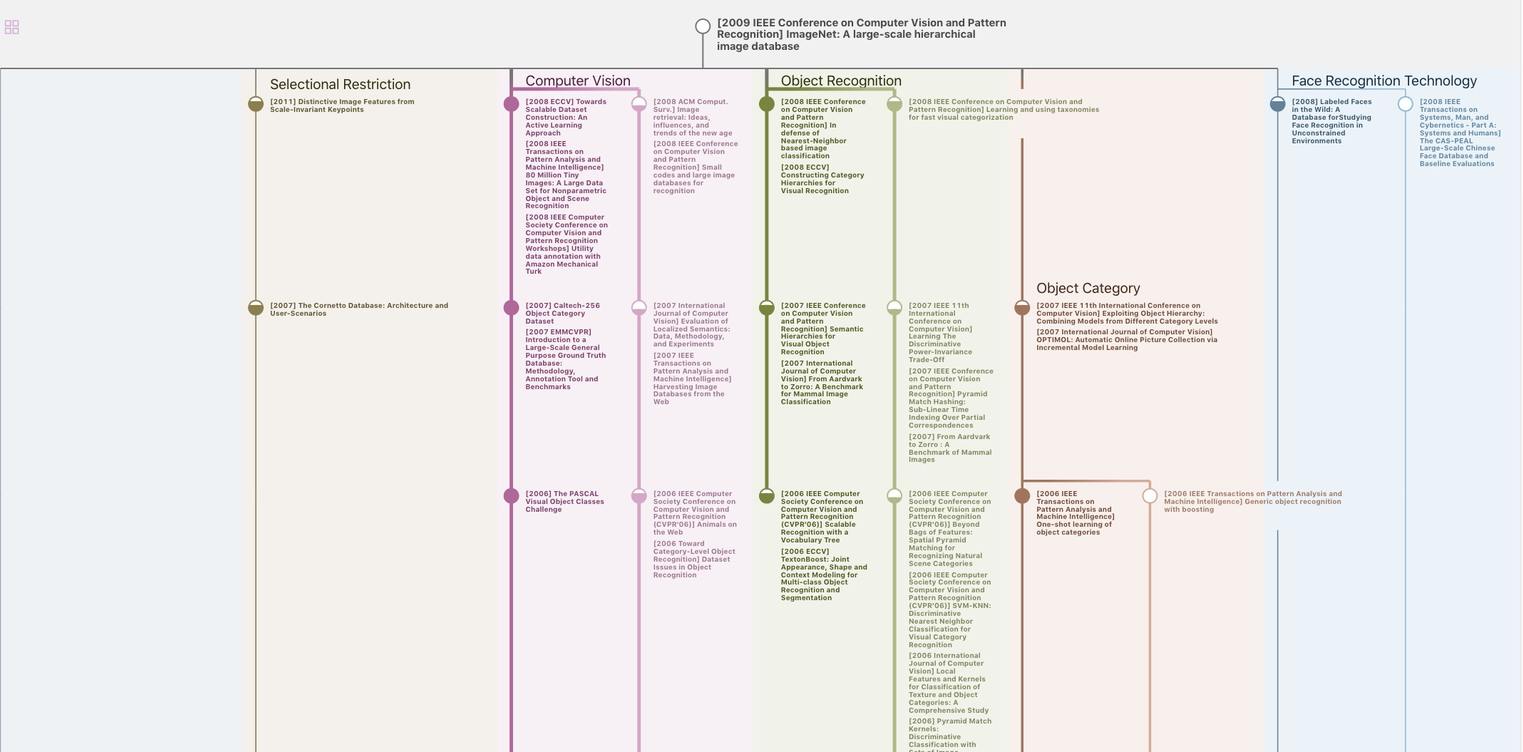
生成溯源树,研究论文发展脉络
Chat Paper
正在生成论文摘要