A slice classification neural network for automated classification of axial PET/CT slices from a multi-centric lymphoma dataset
MEDICAL IMAGING 2023(2023)
摘要
Automated slice classification is clinically relevant since it can be incorporated into medical image segmentation workflows as a preprocessing step that would flag slices with a higher probability of containing tumors, thereby directing physicians' attention to the important slices. In this work, we train a ResNet-18 network to classify axial slices of lymphoma PET/CT images (collected from two institutions) depending on whether the slice intercepted a tumor (positive slice) in the 3D image or if the slice did not (negative slice). Various instances of the network were trained on 2D axial datasets created in different ways: (i) slice-level split and (ii) patient-level split; inputs of different types were used: (i) only PET slices and (ii) concatenated PET and CT slices; and different training strategies were employed: (i) center-aware (CAW) and (ii) center-agnostic (CAG). Model performances were compared using the area under the receiver operating characteristic curve (AUROC) and the area under the precision-recall curve (AUPRC), and various binary classification metrics. We observe and describe a performance overestimation in the case of slice-level split as compared to the patient-level split training. The model trained using patient-level split data with the network input containing only PET slices in the CAG training regime was the best performing/generalizing model on a majority of metrics. Our models were additionally more closely compared using the sensitivity metric on the positive slices from their respective test sets.
更多查看译文
关键词
Lymphoma, F-18-FDG PET/CT, binary classification, ResNet-18, Focal loss, center-aware training, center-agnostic training, SUVmax
AI 理解论文
溯源树
样例
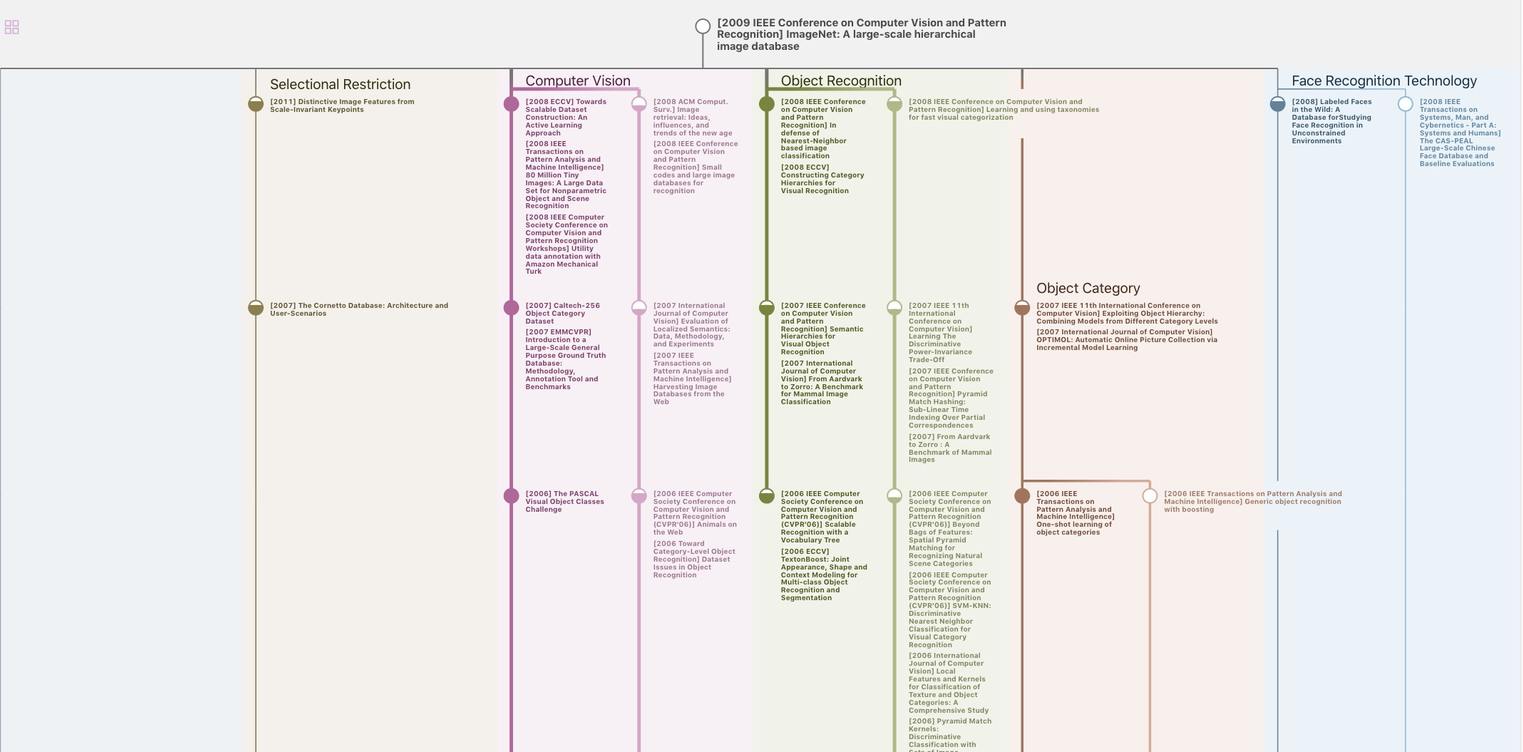
生成溯源树,研究论文发展脉络
Chat Paper
正在生成论文摘要