Sparse Waypoint Validity Checking for Self-Entanglement-Free Tethered Path Planning.
CoRR(2023)
Abstract
A novel mechanism to derive self-entanglement-free (SEF) path for tethered differential-driven robots is proposed in this work. The problem is tailored to the deployment of tethered differential-driven robots in situations where an omni-directional tether re-tractor is not available. This is frequently encountered when it is impractical to concurrently equip an omni-directional tether retracting mechanism with other geometrically intricate devices, such as a manipulator, which is notably relevant in applications like disaster recovery, spatial exploration, etc. Without specific attention to the spatial relation between the shape of the tether and the pose of the mobile unit, the issue of self-entanglement arises when the robot moves, resulting in unsafe robot movements and the risk of damaging the tether. In this paper, the SEF constraint is first formulated as the boundedness of a relative angle function which characterises the angular difference between the tether stretching direction and the robot's heading direction. Then, a constrained searching-based path planning algorithm is proposed which produces a path that is sub-optimal whilst ensuring the avoidance of tether self-entanglement. Finally, the algorithmic efficiency of the proposed path planner is further enhanced by proving the conditioned sparsity of the primitive path validity checking module. The effectiveness of the proposed algorithm is assessed through case studies, comparing its performance against untethered differential-driven planners in challenging planning scenarios. A comparative analysis is further conducted between the normal node expansion module and the improved node expansion module which incorporates sparse waypoint validity checking. Real-world tests are also conducted to validate the algorithm's performance. An open-source implementation has also made available for the benefit of the robotics community.
MoreTranslated text
Key words
planning,path,self-entanglement-free
AI Read Science
Must-Reading Tree
Example
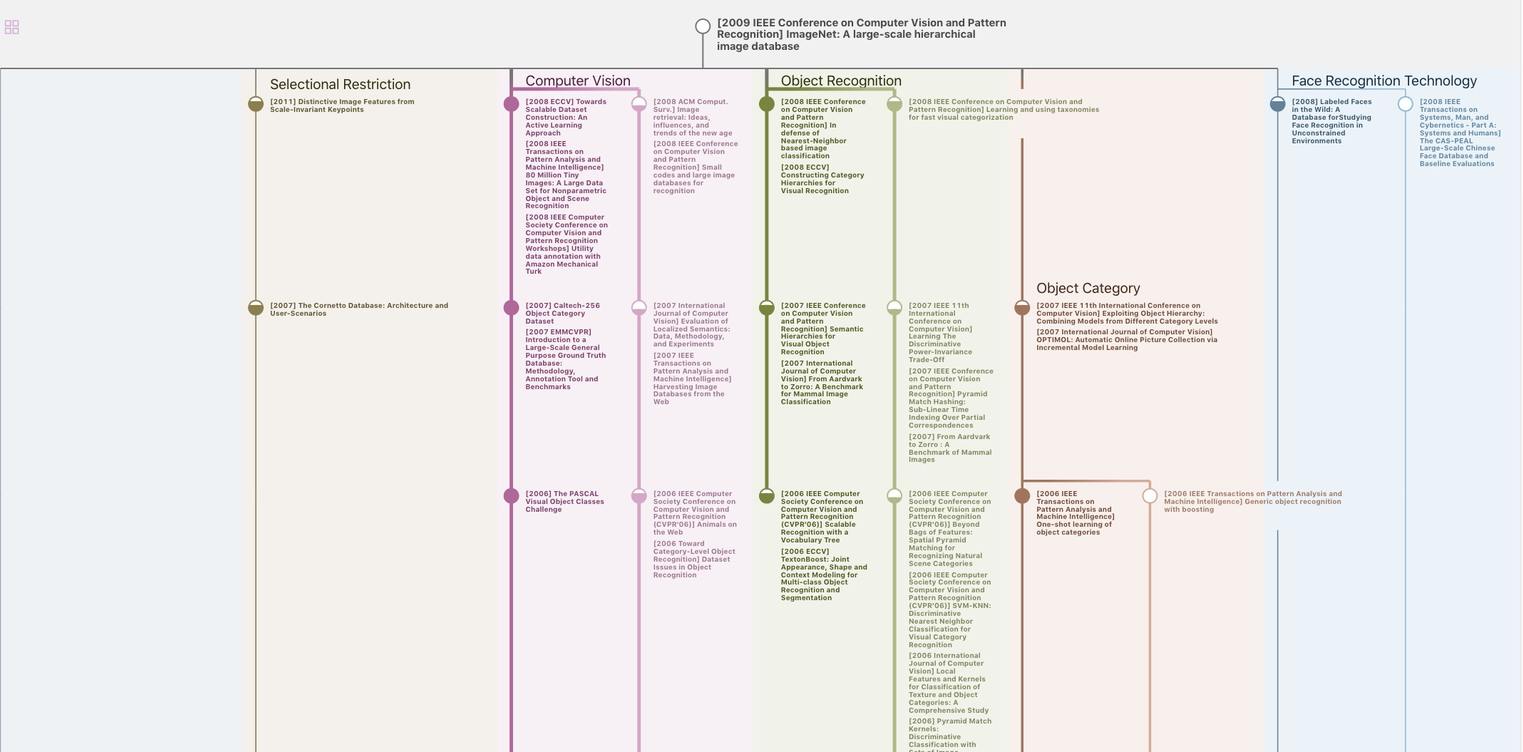
Generate MRT to find the research sequence of this paper
Chat Paper
Summary is being generated by the instructions you defined