Spatial Upsampling of Head-Related Transfer Functions Using a Physics-Informed Neural Network
arXiv (Cornell University)(2023)
摘要
Head-related transfer function (HRTF) capture the information that a person uses to localize sound sources in space, and thus is crucial for creating personalized virtual acoustic experiences. However, practical HRTF measurement systems may only measure a person's HRTFs sparsely, and this necessitates HRTF upsampling. This paper proposes a physics-informed neural network (PINN) method for HRTF upsampling. The PINN exploits the Helmholtz equation, the governing equation of acoustic wave propagation, for regularizing the upsampling process. This helps the generation of physically valid upsamplings which generalize beyond the measured HRTF. Furthermore, the size (width and depth) of the PINN is set according to the Helmholtz equation and its solutions, the spherical harmonics (SHs). This makes the PINN have an appropriate level of expressive power and thus does not suffer from the over-fitting problem. Since the PINN is designed independent of any specific HRTF dataset, it offers more generalizability compared to pure data-driven methods. Numerical experiments confirm the better performance of the PINN method for HRTF upsampling in both interpolation and extrapolation scenarios in comparison with the SH method and the HRTF field method.
更多查看译文
关键词
neural network,physics,transfer function,head-related
AI 理解论文
溯源树
样例
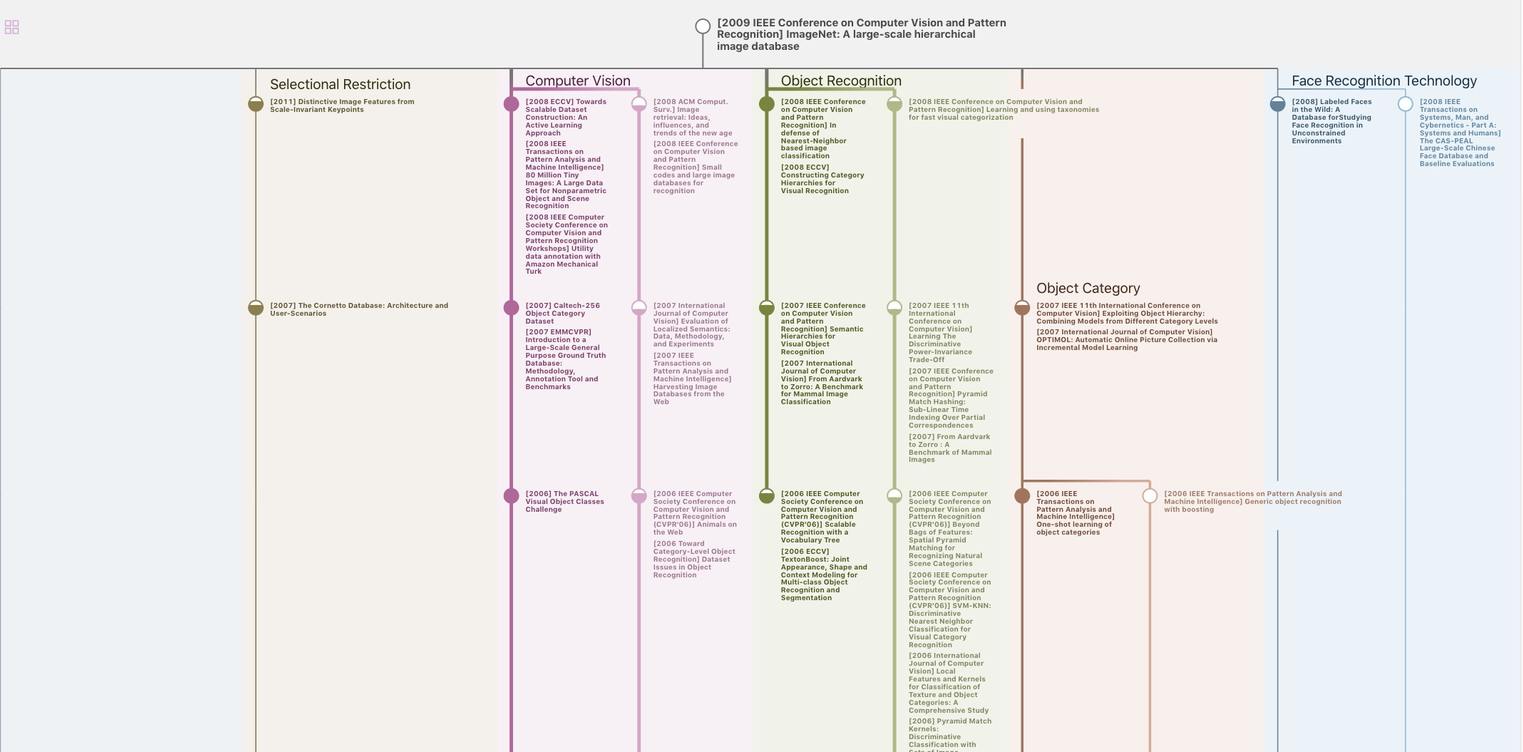
生成溯源树,研究论文发展脉络
Chat Paper
正在生成论文摘要