Learning to reconstruct the bubble distribution with conductivity maps using Invertible Neural Networks and Error Diffusion
CoRR(2023)
摘要
Electrolysis is crucial for eco-friendly hydrogen production, but gas bubbles
generated during the process hinder reactions, reduce cell efficiency, and
increase energy consumption. Additionally, these gas bubbles cause changes in
the conductivity inside the cell, resulting in corresponding variations in the
induced magnetic field around the cell. Therefore, measuring these gas
bubble-induced magnetic field fluctuations using external magnetic sensors and
solving the inverse problem of Biot-Savart Law allows for estimating the
conductivity in the cell and, thus, bubble size and location. However,
determining high-resolution conductivity maps from only a few induced magnetic
field measurements is an ill-posed inverse problem. To overcome this, we
exploit Invertible Neural Networks (INNs) to reconstruct the conductivity
field. Our qualitative results and quantitative evaluation using random error
diffusion show that INN achieves far superior performance compared to Tikhonov
regularization.
更多查看译文
关键词
invertible neural networks,bubble distribution,conductivity maps
AI 理解论文
溯源树
样例
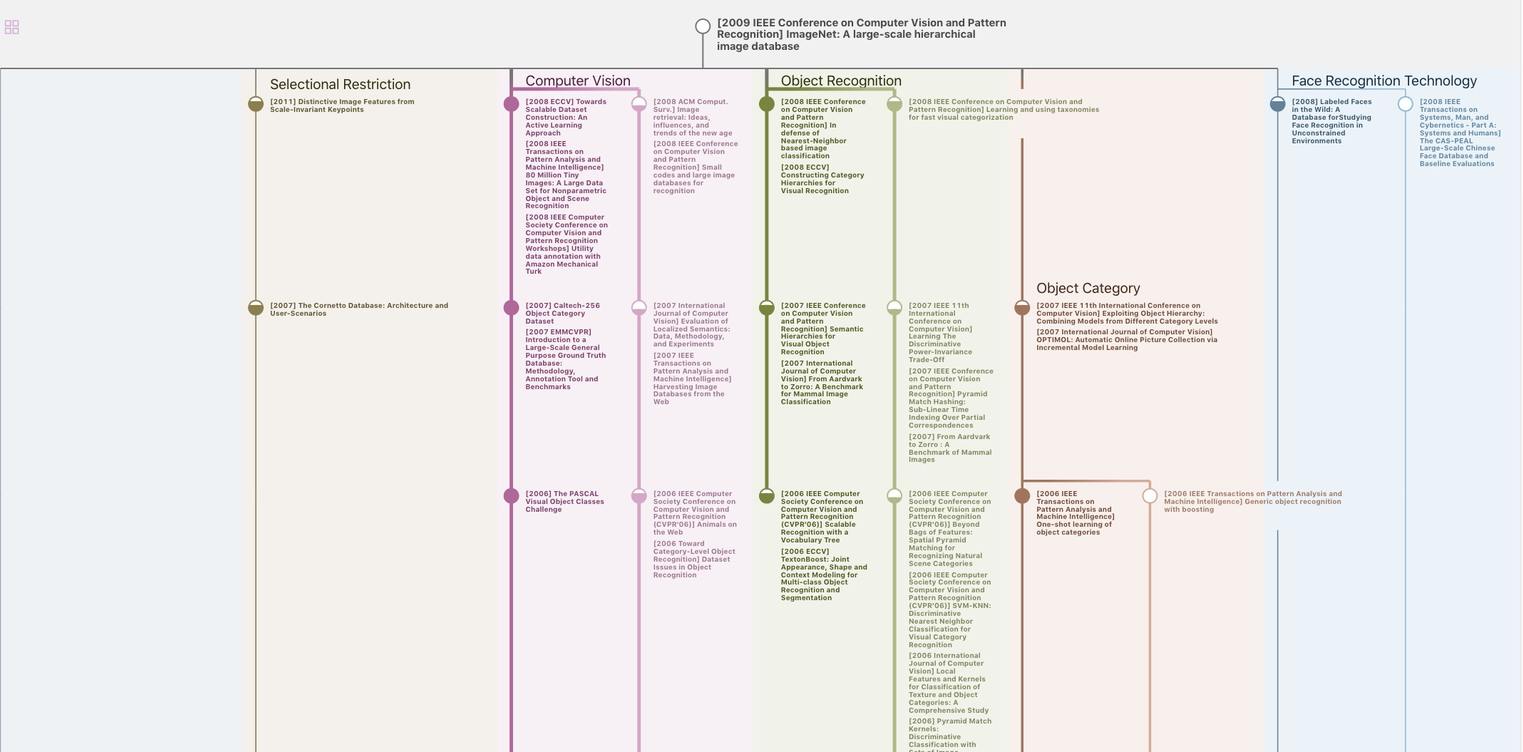
生成溯源树,研究论文发展脉络
Chat Paper
正在生成论文摘要