Learning dynamic image representations for self-supervised cell cycle annotation
bioRxiv (Cold Spring Harbor Laboratory)(2023)
摘要
Time-based comparisons of single-cell trajectories are challenging due to their intrinsic heterogeneity, autonomous decisions, dynamic transitions and unequal lengths. In this paper, we present a self-supervised framework combining an image autoencoder with dynamic time series analysis of latent feature space to represent, compare and annotate cell cycle phases across singlecell trajectories. In our fully data-driven approach, we map similarities between heterogeneous cell tracks and generate statistical representations of single-cell trajectory phase durations, onset and transitions. This work is a first effort to transform a sequence of learned image representations from cell cycle-specific reporters into an unsupervised sequence annotation.
### Competing Interest Statement
The authors have declared no competing interest.
更多查看译文
关键词
dynamic image representations,cell cycle,learning,self-supervised
AI 理解论文
溯源树
样例
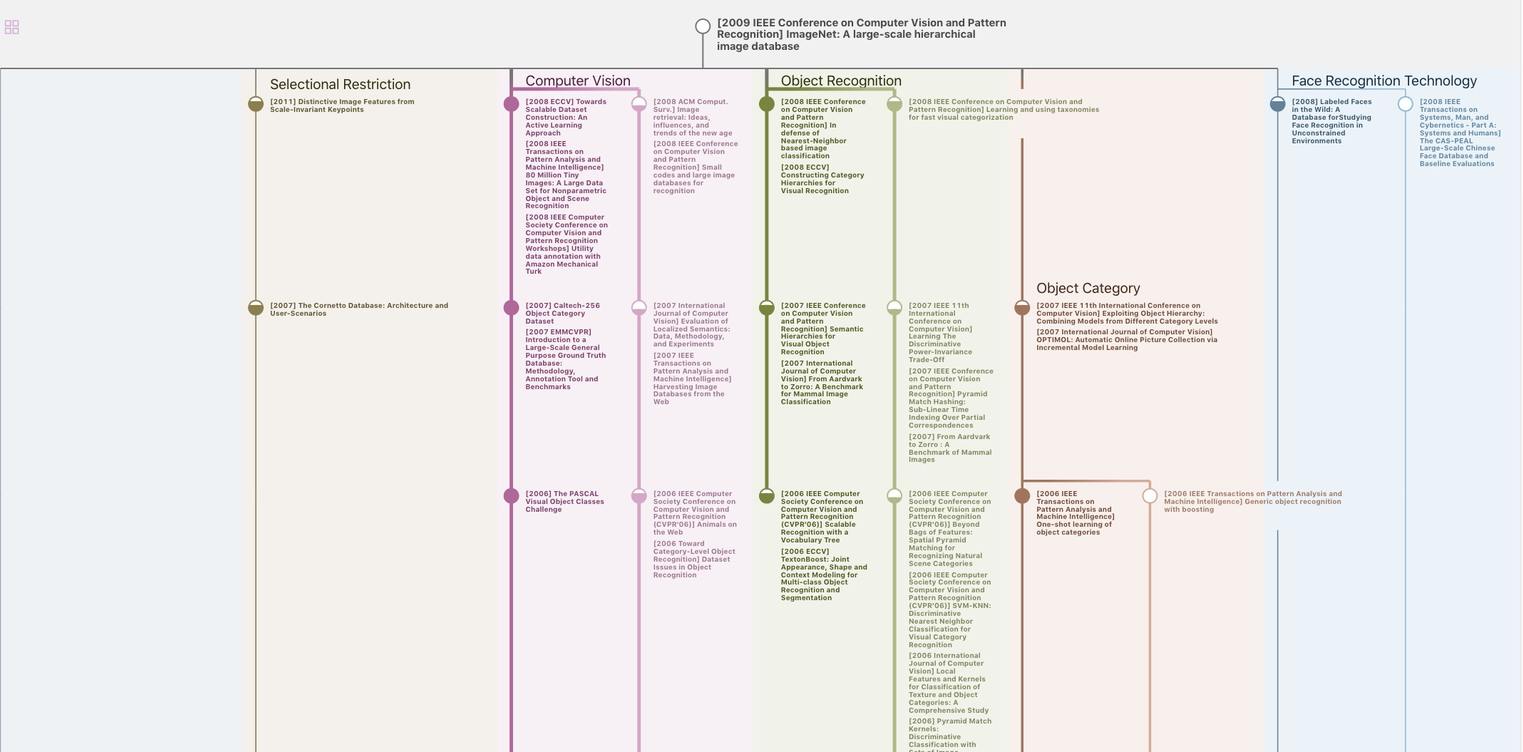
生成溯源树,研究论文发展脉络
Chat Paper
正在生成论文摘要