A Smoothing Layer for SAR Image Semantic Segmentation with Fully Convolutional Networks.
JURSE(2023)
摘要
The publicly accessible Sentinel 1 satellite has paved the way for numerous applications such as environmental monitoring, military surveillance, and land cover mapping. This has increased the need for efficient and high-performance semantic segmentation methods for SAR images. However, even though the existing semantic segmentation networks use pixel- and feature-level spatial information, they neglect neighborhood label consistency. A new smoothing layer composed of symmetric kernels is proposed to overcome this problem. A smoothing layer is added to a segmentation network’s output layer to obtain a new end-to-end trainable segmentation network. The proposed layer has been validated with U-Net and DeeplabV3+, and its contribution to segmentation performance is reported. The experiments have been conducted on two real SAR datasets. The contribution of the smoothing layer to segmentation performance has been demonstrated via metrics such as overall accuracy, kappa, and mean intersection over union. The source code for the smoothing layer is publicly available
1
.
更多查看译文
关键词
Sentinel 1,SAR image,semantic segmentation,U-Net,DeeplabV3+
AI 理解论文
溯源树
样例
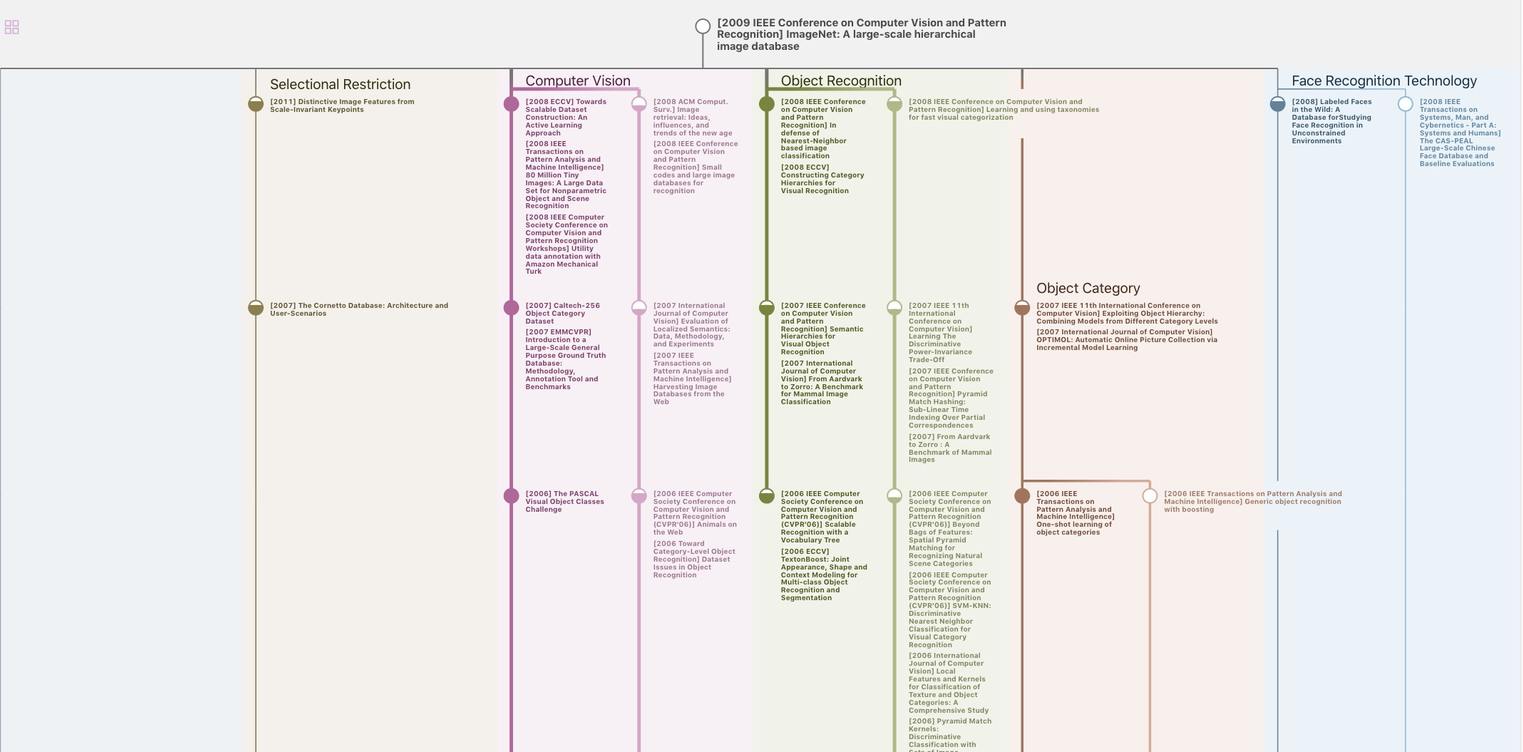
生成溯源树,研究论文发展脉络
Chat Paper
正在生成论文摘要