Mixed-Order Relation-Aware Recurrent Neural Networks for Spatio-Temporal Forecasting
IEEE Transactions on Knowledge and Data Engineering(2023)
摘要
Spatio-temporal forecasting has a wide range of applications in smart city efforts, such as traffic forecasting and air quality prediction. Graph Convolutional Recurrent Neural Networks (GCRNN) are the state-of-the-art methods for this problem, which learn temporal dependencies by RNNs and exploit pairwise node proximity to model spatial dependencies. However, the spatial relations in real data are not simply pairwise but sometimes in a higher order among multiple nodes. Moreover, spatio-temporal sequences deriving from nature are often regulated by known or unknown physical laws. GCRNNs rarely take into account the underlying physics in real-world systems, which may result in degenerated performance. To address these issues, we devise a general model called Mixed-Order Relation-Aware RNN (MixRNN+) for spatio-temporal forecasting. Specifically, our MixRNN+ captures the complex mixed-order spatial relations of nodes through a newly proposed building block called Mixer, and simultaneously addressing the underlying physics by the integration of a new residual update strategy. Experimental results on three forecasting tasks in smart city applications (including traffic speed, taxi flow, and air quality prediction) demonstrate the superiority of our model against the state-of-the-art methods. We have also deployed a cloud-based system using our method as the bedrock model to show its practicality.
更多查看译文
关键词
Physics-informed neural networks, reaction kinetics, spatio-temporal data mining, urban computing
AI 理解论文
溯源树
样例
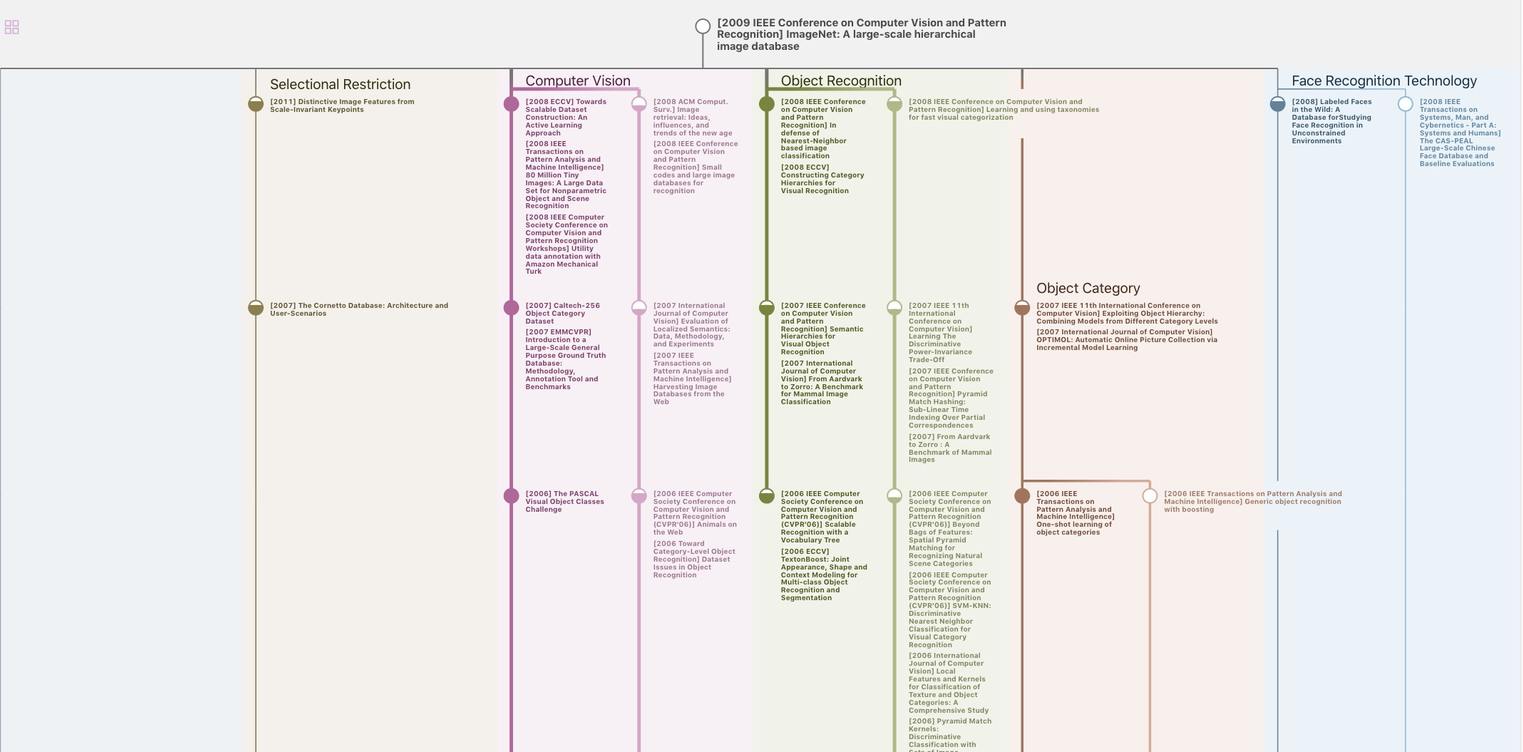
生成溯源树,研究论文发展脉络
Chat Paper
正在生成论文摘要