A Multilabel Active Learning Framework for Microcontroller Performance Screening
IEEE Transactions on Computer-Aided Design of Integrated Circuits and Systems(2023)
摘要
In safety-critical applications, microcontrollers have to be tested to satisfy strict quality and performance constraints. It has been demonstrated that on-chip ring oscillators can be used as speed monitors to reliably predict the performances. However, any machine-learning (ML) model is likely to be inaccurate if trained on an inadequate dataset, and labeling data for training is quite a costly process. In this article, we present a methodology based on active learning to select the best samples to be included in the training set, significantly reducing the time and cost required. Moreover, since different speed measurements are available, we designed a multilabel technique to take advantage of their correlations. Experimental results demonstrate that the approach halves the training-set size, with respect to a random-labeling, while it increases the predictive accuracy, with respect to standard single-label ML models.
更多查看译文
关键词
Active learning (AL),device testing,Fmax,machine learning (ML),performance screening,speed monitors (SMONs)
AI 理解论文
溯源树
样例
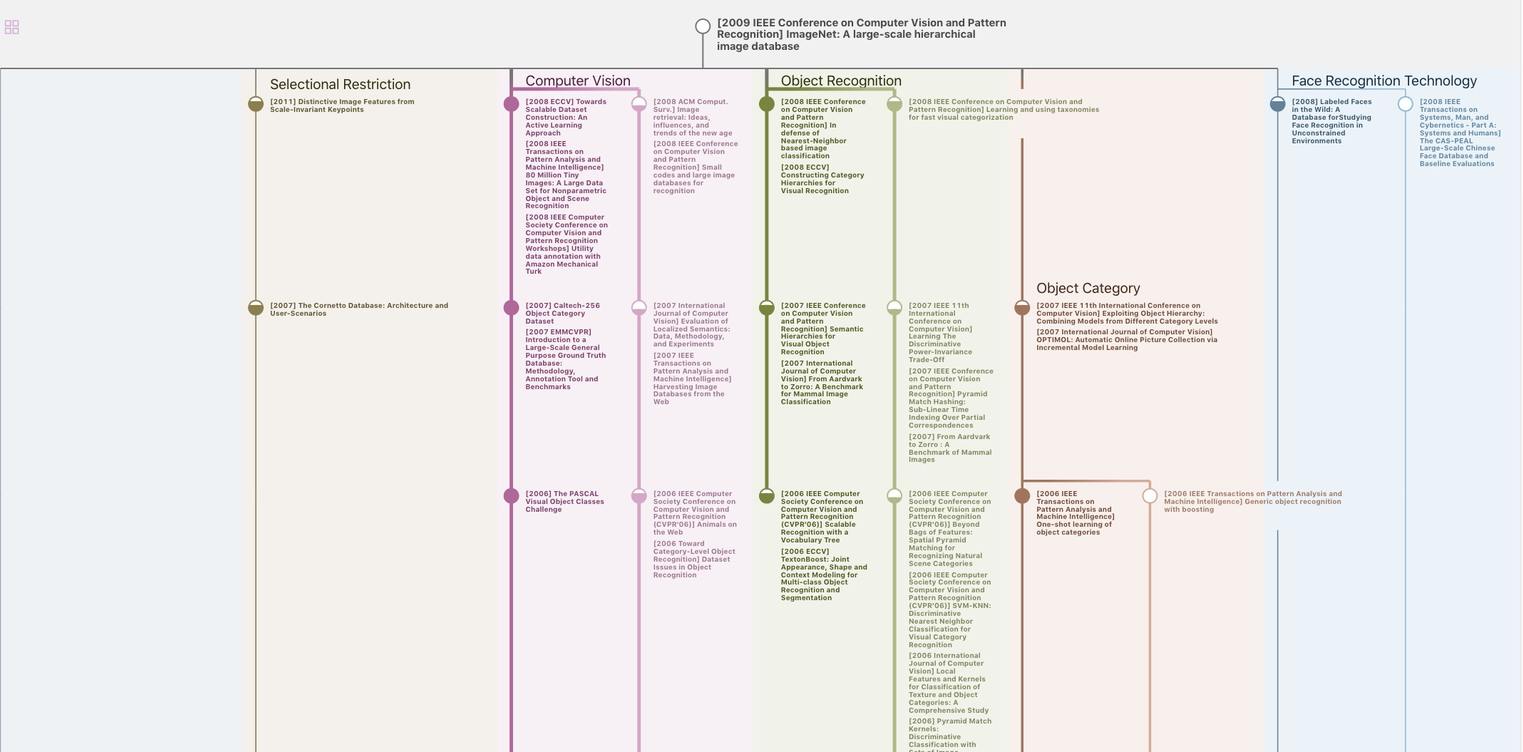
生成溯源树,研究论文发展脉络
Chat Paper
正在生成论文摘要