Self-Supervised Reinforcement Learning that Transfers using Random Features
NeurIPS(2023)
摘要
Model-free reinforcement learning algorithms have exhibited great potential in solving single-task sequential decision-making problems with high-dimensional observations and long horizons, but are known to be hard to generalize across tasks. Model-based RL, on the other hand, learns task-agnostic models of the world that naturally enables transfer across different reward functions, but struggles to scale to complex environments due to the compounding error. To get the best of both worlds, we propose a self-supervised reinforcement learning method that enables the transfer of behaviors across tasks with different rewards, while circumventing the challenges of model-based RL. In particular, we show self-supervised pre-training of model-free reinforcement learning with a number of random features as rewards allows implicit modeling of long-horizon environment dynamics. Then, planning techniques like model-predictive control using these implicit models enable fast adaptation to problems with new reward functions. Our method is self-supervised in that it can be trained on offline datasets without reward labels, but can then be quickly deployed on new tasks. We validate that our proposed method enables transfer across tasks on a variety of manipulation and locomotion domains in simulation, opening the door to generalist decision-making agents.
更多查看译文
关键词
learning,reinforcement,transfers,random
AI 理解论文
溯源树
样例
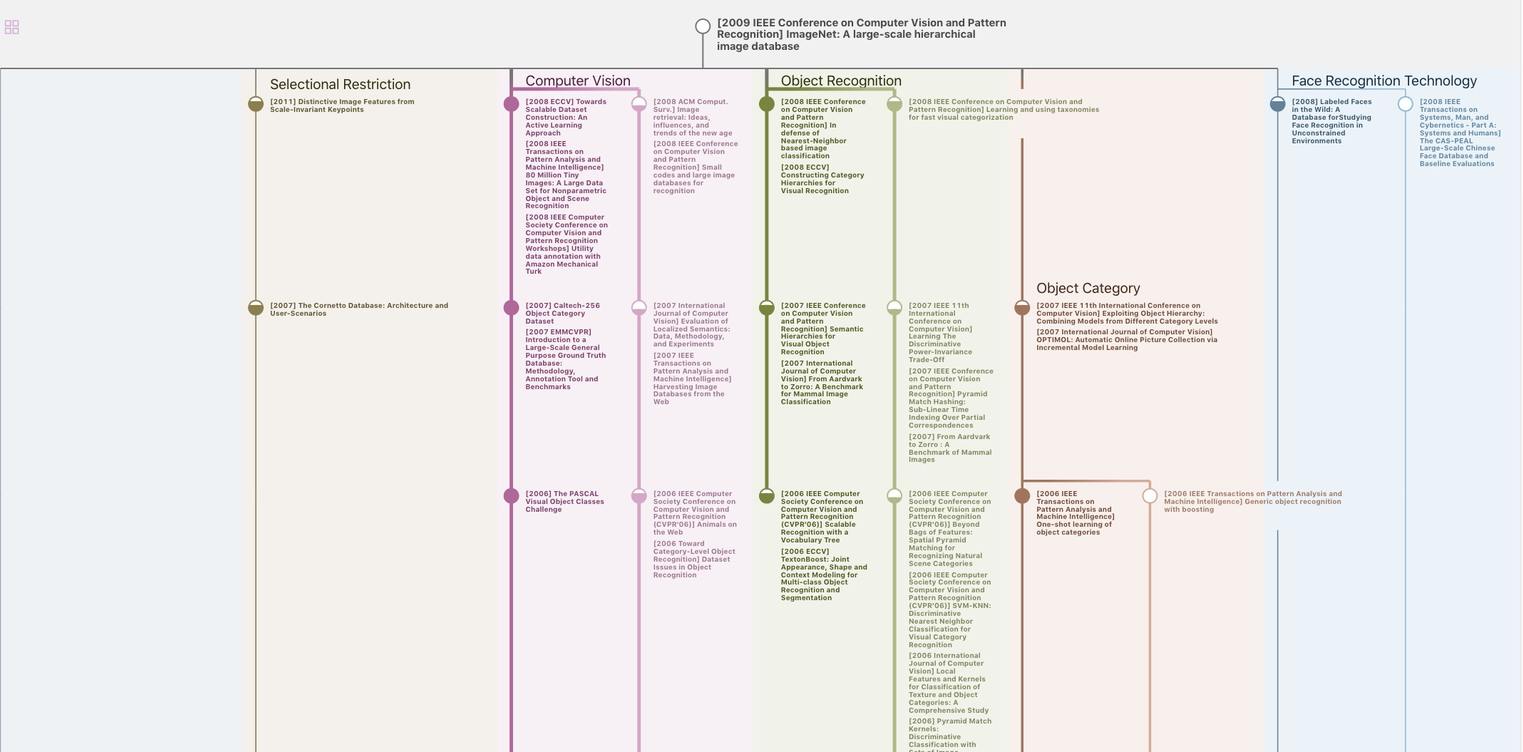
生成溯源树,研究论文发展脉络
Chat Paper
正在生成论文摘要