MOTRv3: Release-Fetch Supervision for End-to-End Multi-Object Tracking
CoRR(2023)
摘要
Although end-to-end multi-object trackers like MOTR enjoy the merits of simplicity, they suffer from the conflict between detection and association seriously, resulting in unsatisfactory convergence dynamics. While MOTRv2 partly addresses this problem, it demands an additional detection network for assistance. In this work, we serve as the first to reveal that this conflict arises from the unfair label assignment between detect queries and track queries during training, where these detect queries recognize targets and track queries associate them. Based on this observation, we propose MOTRv3, which balances the label assignment process using the developed release-fetch supervision strategy. In this strategy, labels are first released for detection and gradually fetched back for association. Besides, another two strategies named pseudo label distillation and track group denoising are designed to further improve the supervision for detection and association. Without the assistance of an extra detection network during inference, MOTRv3 achieves impressive performance across diverse benchmarks, e.g., MOT17, DanceTrack.
更多查看译文
关键词
supervision,release-fetch,end-to-end,multi-object
AI 理解论文
溯源树
样例
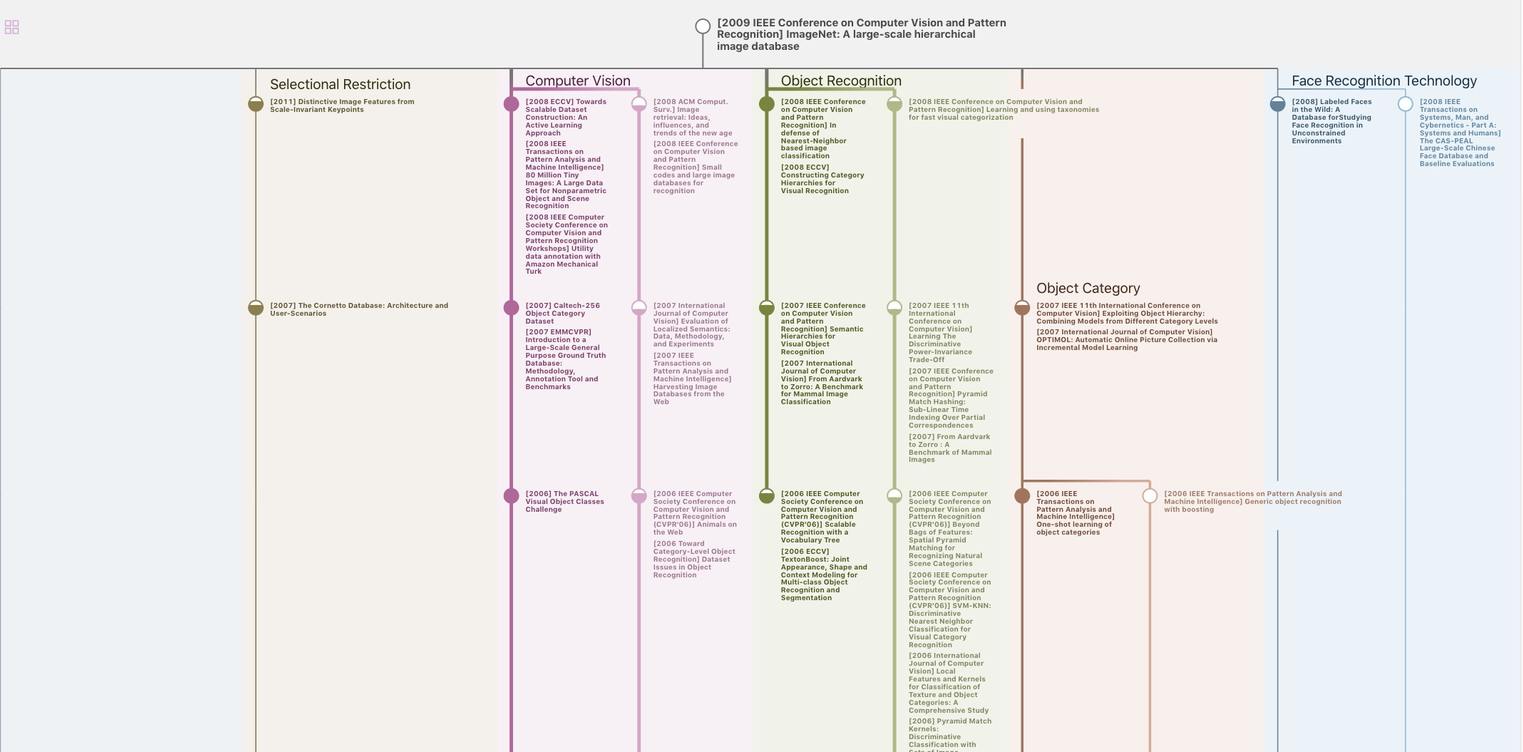
生成溯源树,研究论文发展脉络
Chat Paper
正在生成论文摘要