Study of RRAM-Based Binarized Neural Networks Inference Accelerators Using an RRAM Physics-Based Compact Model
Neuromorphic Computing [Working Title]Artificial Intelligence(2023)
摘要
In-memory computing hardware accelerators for binarized neural networks based on resistive RAM (RRAM) memory technologies represent a promising solution for enabling the execution of deep neural network algorithms on resource-constrained devices at the edge of the network. However, the intrinsic stochasticity and nonidealities of RRAM devices can easily lead to unreliable circuit operations if not appropriately considered during the design phase. In this chapter, analysis and design methodologies enabled by RRAM physics-based compact models of LIM and mixed-signal BNN inference accelerators are discussed. As a use case example, the UNIMORE RRAM physics-based compact model calibrated on an RRAM technology from the literature, is used to determine the performance vs. reliability trade-offs of different in-memory computing accelerators: i) a logic-in-memory accelerator based on the material implication logic, ii) a mixed-signal BNN accelerator, and iii) a hybrid accelerator enabling both computing paradigms on the same array. Finally, the performance of the three accelerators on a BNN inference task is compared and benchmarked with the state of the art.
更多查看译文
关键词
rram-based,physics-based
AI 理解论文
溯源树
样例
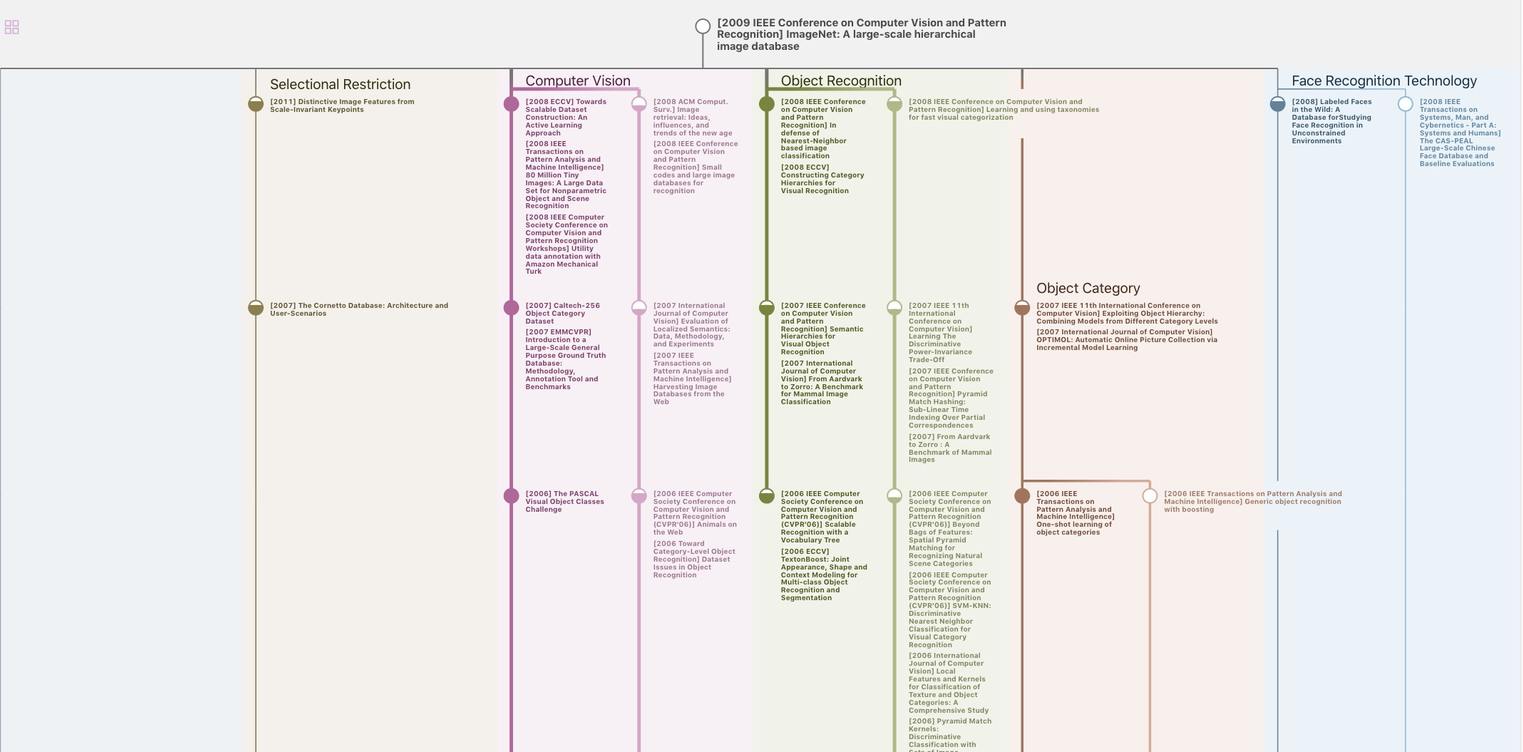
生成溯源树,研究论文发展脉络
Chat Paper
正在生成论文摘要