Graph-based Fusion of Imaging and Non-Imaging Data for Disease Trajectory Prediction
2023 11th International IEEE/EMBS Conference on Neural Engineering (NER)(2023)
摘要
This study proposes a graph convolutional neural networks (GCN) architecture for fusion of radiological imaging and non-imaging tabular electronic health records (EHR) for the purpose of clinical event prediction. We focused on a cohort of hospitalized patients with positive RT-PCR test for COVID-19 and developed GCN based models to predict three dependent clinical events (discharge from hospital, admission into ICU, and mortality) using demographics, billing codes for procedures and diagnoses and chest X-rays. We hypothesized that the two-fold learning opportunity provided by the GCN is ideal for fusion of imaging information and tabular data as node and edge features, respectively. Our experiments indicate the validity of our hypothesis where GCN based predictive models outperform single modality and traditional fusion models. We compared the proposed models against two variations of imaging-based models, including DenseNet-121 architecture with learnable classification layers and Random Forest classifiers using disease severity score estimated by pre-trained convolutional neural network. GCN based model outperforms both imaging-only methods. We also validated our models on an external dataset where GCN showed valuable generalization capabilities. We noticed that edgeformation function can be adapted even after training the GCN model without limiting application scope of the model. Our models take advantage of this fact for generalization to external data.
更多查看译文
关键词
clinical event prediction,graph convolutional neural network,multi,modal data fusion
AI 理解论文
溯源树
样例
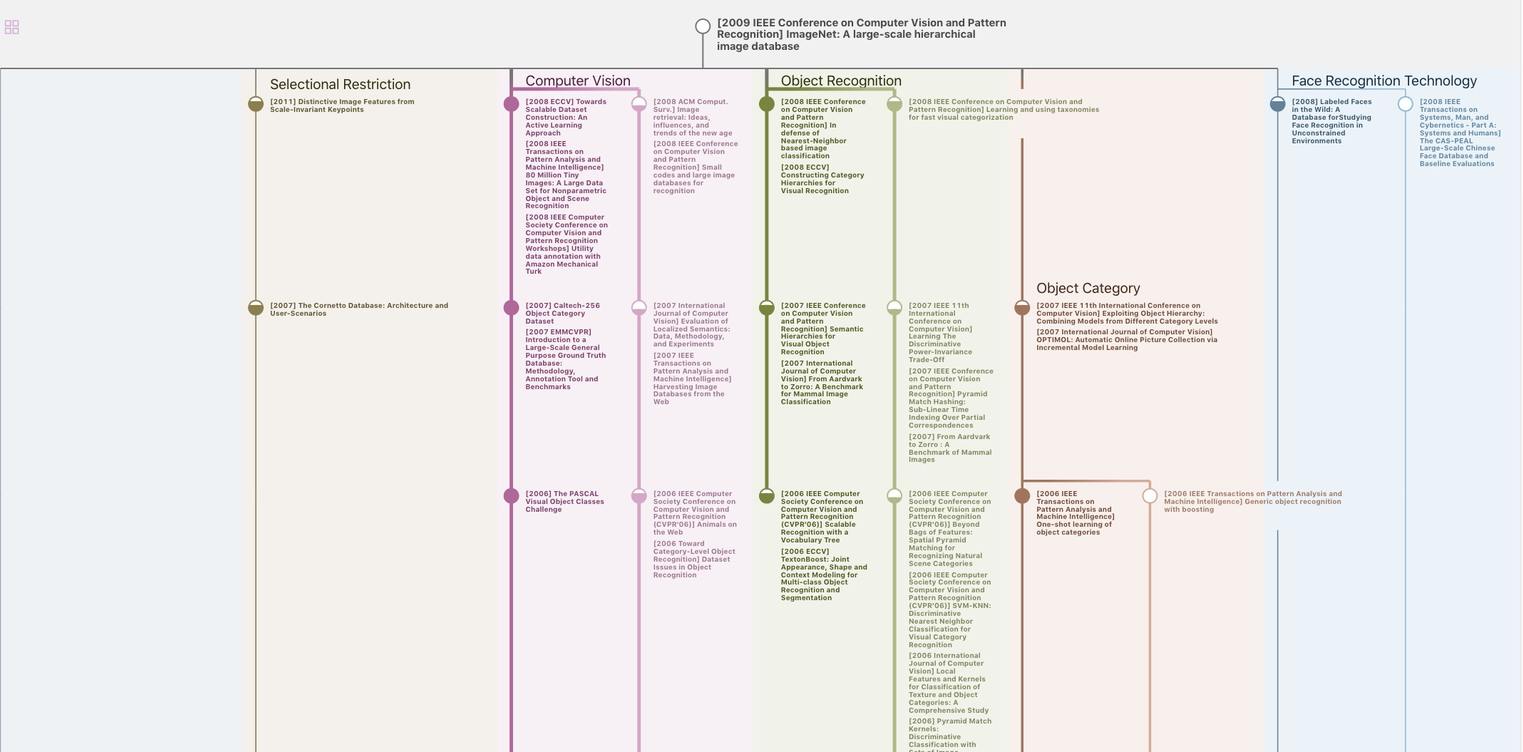
生成溯源树,研究论文发展脉络
Chat Paper
正在生成论文摘要