Federated learning in smart cities: Privacy and security survey.
Inf. Sci.(2023)
Abstract
Over the last decade, smart cities (SC) have been developed worldwide. Implementing big data and the internet of things improves the monitoring and integration of different infrastructure systems in SC and thus makes our cities more efficient, livable, and sustainable. However, big data is more vulnerable to attacks and hacking, posing significant challenges to the SC's privacy and security. Moreover, more regulations and rules about protecting user data are being enforced worldwide. Compared with centralized machine learning, federated learning (FL) provides a natural method to protect users' privacy by distributing learning over decentralized data and offers artificial intelligence advantages for sensitive, heterogeneous data domains. Researchers have recently implemented FL, motivated by the improvement in privacy and security of integrated systems in SC. However, many technological problems and risks have been identified. This study provides a comprehensive review of FL's application to improve privacy and security in SC systems (i.e., transportation, healthcare, communication, etc.). The benefits, drawbacks, open research issues and future directions of the implementation of FL in SC are thoroughly discussed. The study concluded that the existing FL systems required comprehensive testing for different types of attacks, further improving the data protection and performance in SC.
MoreTranslated text
Key words
Federated learning, Smart cities, Security and privacy
AI Read Science
Must-Reading Tree
Example
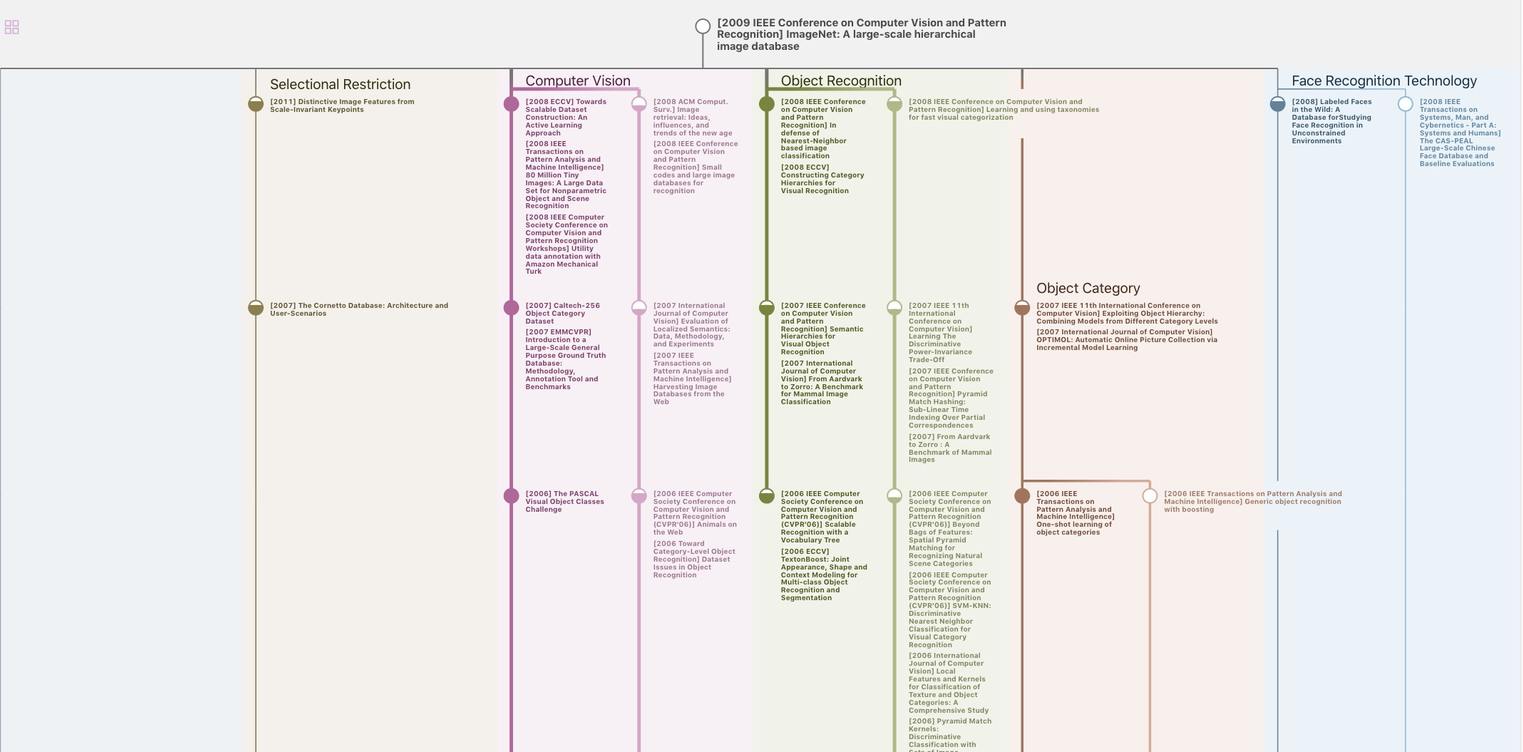
Generate MRT to find the research sequence of this paper
Chat Paper
Summary is being generated by the instructions you defined