A Charge Domain SRAM Compute-in-Memory Macro With C-2C Ladder-Based 8-Bit MAC Unit in 22-nm FinFET Process for Edge Inference
IEEE Journal of Solid-State Circuits(2023)
摘要
Compute-in-memory (CiM) is one promising solution to address the memory bottleneck existing in traditional computing architectures. However, the tradeoff between energy efficiency and computing precision plagues most CiM implementations, and the low precision imposes a major limitation on CiM’s ability to support practical computational workloads. In this article, a static random access memory (SRAM)-based analog CiM macro is presented with the Intel 22FFL process. By introducing a 1-to-2 ratioed capacitor ladder (C-2C)-based charge domain computing scheme, the proposed CiM prototype chip demonstrates a maximum of 2k multiply-accumulate (MAC) operations in one clock cycle and achieves 32.2-TOPS/W peak power efficiency with 8-bit precision in both input activation and weight while ensuring accurate on-chip matrix–vector multiplications (MVMs) with a computation error less than 0.5%. A 4.0-TOPS/mm2 peak area efficiency is attained by adopting a local weight multiplexing scheme with a 9T SRAM cell, which improves the memory density and reduces the need to refresh the weight stored in the SRAM array. A variety of analog impairment factors, including parasitics, mismatch, and noise, were analyzed to guarantee a sufficiently high multibit linearity. The proposed passive analog computing mechanism ensures the computation accuracy over process–voltage–temperature (PVT), with the measured MVM error deviation of less than 1% over PVT variations.
更多查看译文
关键词
Analog computing,charge domain computing,CMOS,compute-in-memory (CiM),machine learning (ML) accelerator,mixed signal,multiply-accumulate (MAC) operation,neural networks (NNs),static random access memory (SRAM)
AI 理解论文
溯源树
样例
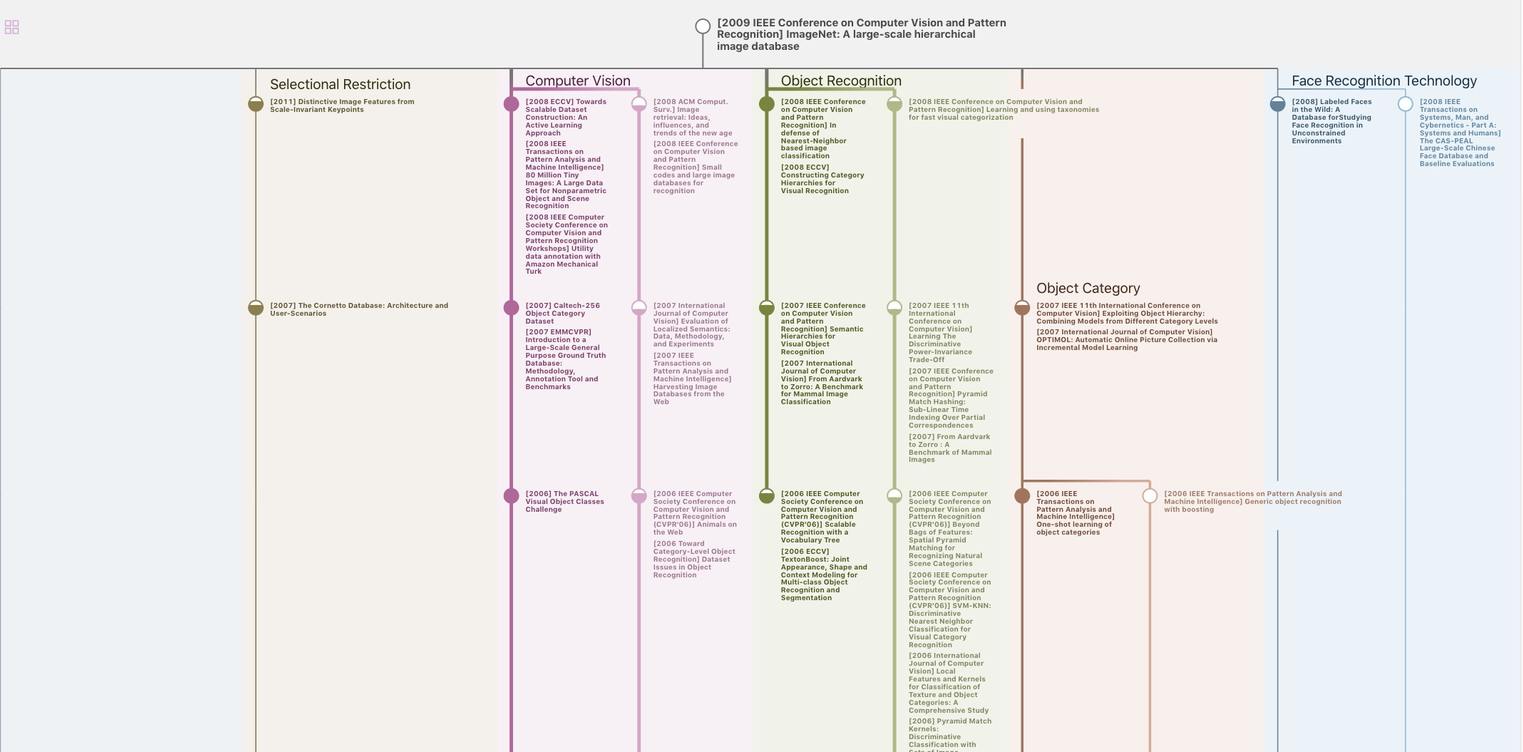
生成溯源树,研究论文发展脉络
Chat Paper
正在生成论文摘要