Sparse Measurement Data Driven Air-to-Ground Path Loss Prediction over Vegetation Area.
VTC Fall(2022)
摘要
In this paper, a novel path loss (PL) prediction model is proposed for the obstructed-line-of-sight (OLoS) and non-line-of-sight (NLoS) paths in unmanned aerial vehicle (UAV) communication over vegetation areas. The proposed PL prediction model is designed based on a deep neural network (DNN) with a pre-training module (PTM). We pre-train the DNN by ray tracing (RT) simulation data and then optimize the network by sparse measurement data, which can significantly reduce the demand for measurement data. Moreover, PL measurements over vegetation areas are carried out at 2 GHz on the campus to validate the proposed model. It is shown that the prediction results of the proposed model are in good agreement with the measurement data and the ones of the fitted International Telecommunication Union recommendation (FITU-R) model under the OLoS case. Moreover, the proposed model is more general and suitable for air-to-ground (A2G) communications by considering the impact of the wide range of reflection angle (RA) variations on the PL.
更多查看译文
关键词
Unmanned aerial vehicle (UAV), path loss (PL), vegetation area, deep neural network (DNN), reflection angle (RA).
AI 理解论文
溯源树
样例
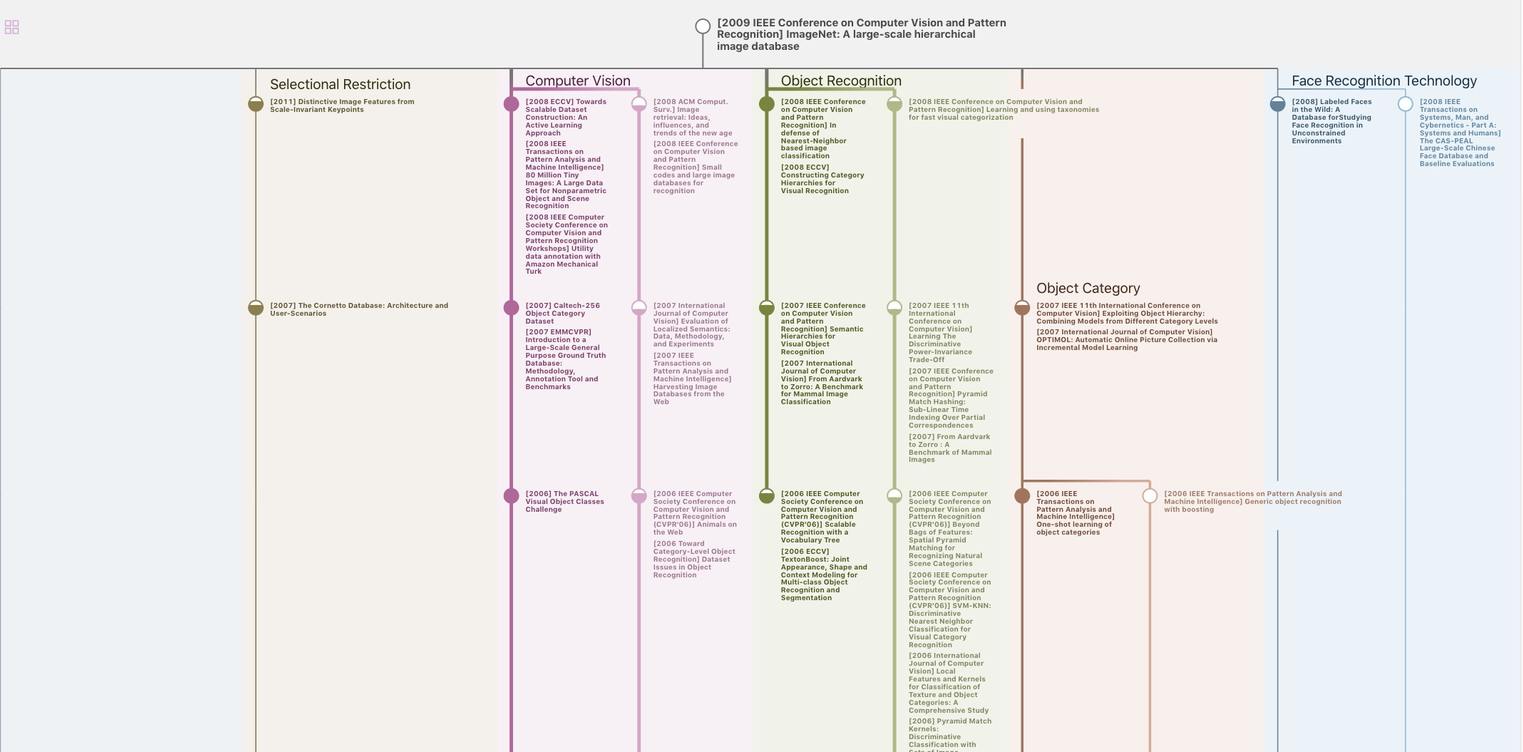
生成溯源树,研究论文发展脉络
Chat Paper
正在生成论文摘要