Study on Memristor-Based 3-D Convolutional Neural Network Adapting to Non-Ideal Effects
IEEE Transactions on Electron Devices(2023)
摘要
Memristor-based neural network (MNN) has made breakthroughs in image classification, but for complex video recognition, the required deep MNN will inevitably be more sensitive to non-ideal effects. In this work, we evaluate a memristor-based 3-D convolutional neural network (CNN) for action recognition and incorporate various non-ideal effects at the circuit and device level. Hardware-friendly schemes such as time division multiplexing (TDM) and updating with increasing thresholds are also described. Furthermore, a strategy combining forward averaging and mapping back is proposed to alleviate the IR drop effect. By setting the intersection conductance as the differential reference, deep MNNs are able to adapt to the updating asymmetry. Under the above adaptive strategies and in situ training, the network achieves an accuracy of 92.9% higher than the software and requires fewer updating pulses.
更多查看译文
关键词
Action recognition,in-memory computing,memristor,neural network,non-ideal effects
AI 理解论文
溯源树
样例
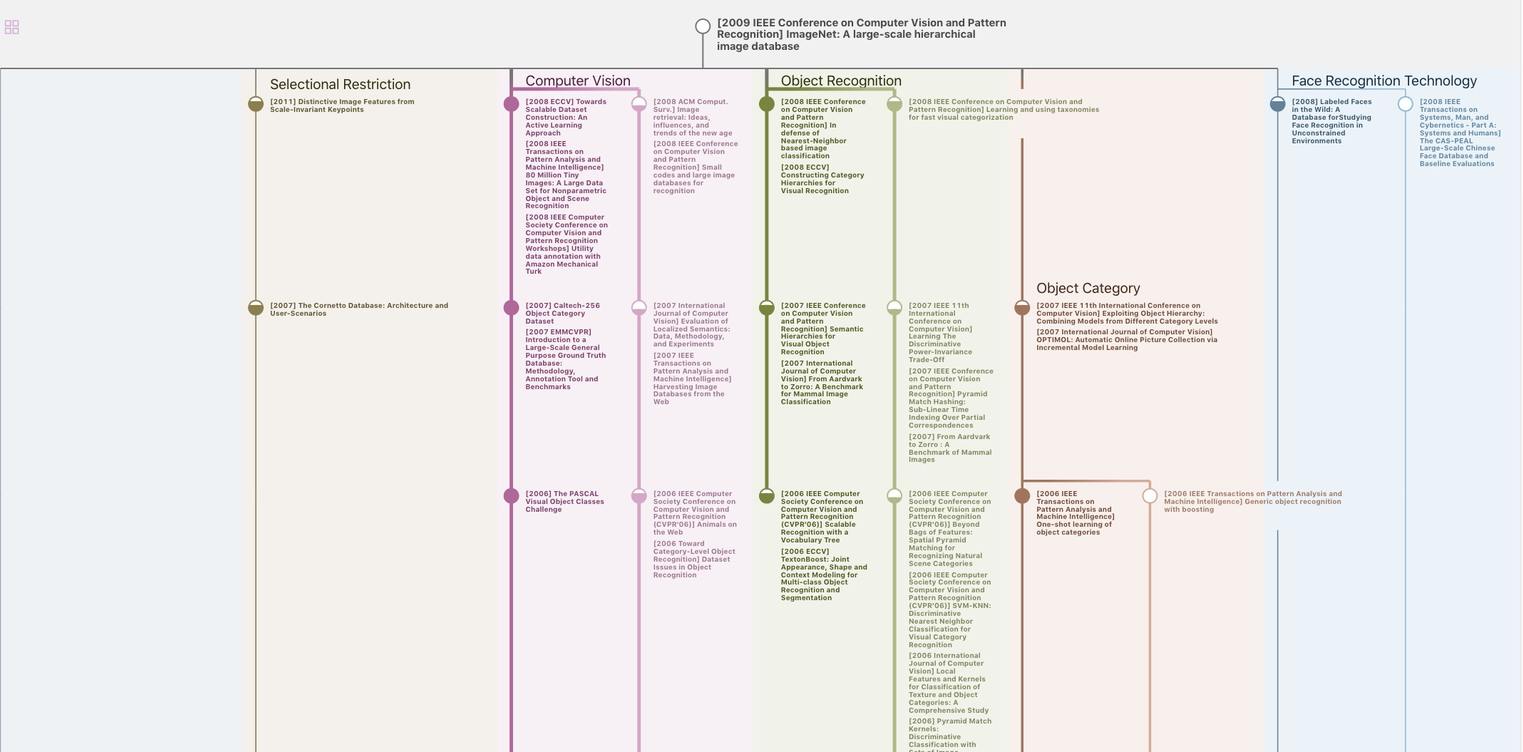
生成溯源树,研究论文发展脉络
Chat Paper
正在生成论文摘要