High spatial granularity residential heating load forecast based on Dendrite net model
Energy(2023)
摘要
With the application of smart meters, more information is available from residential buildings for support heat load forecast. Yet, there is still a lack of an effective method to exploit the value of the high spatial granularity information, particularly for residential communities with high randomness in human behaviors. To fill this gap, this paper proposes a data-driven heat load forecast method based on the field measurements of smart meters A white-box machine learning algorithm, namely Dendritic Network, is employed to aggregate and analyze data obtained from different locations of district heating systems. An online correction mechanism is then proposed to regulate the forecast horizon and ensure the adaptiveness of the machine learning model under different sce-narios. The results demonstrate that the dendritic network employed in the proposed machine learning method shows higher forecast accuracy, the root-mean-square error is 0.0029, in some research cases the coefficient of determination can reach 0.99-1, and wide scope of application in the area of heat load forecast.
更多查看译文
关键词
Residential heating load,Machine learning,Dendritic network,District heating,Systems,High spatial granularity
AI 理解论文
溯源树
样例
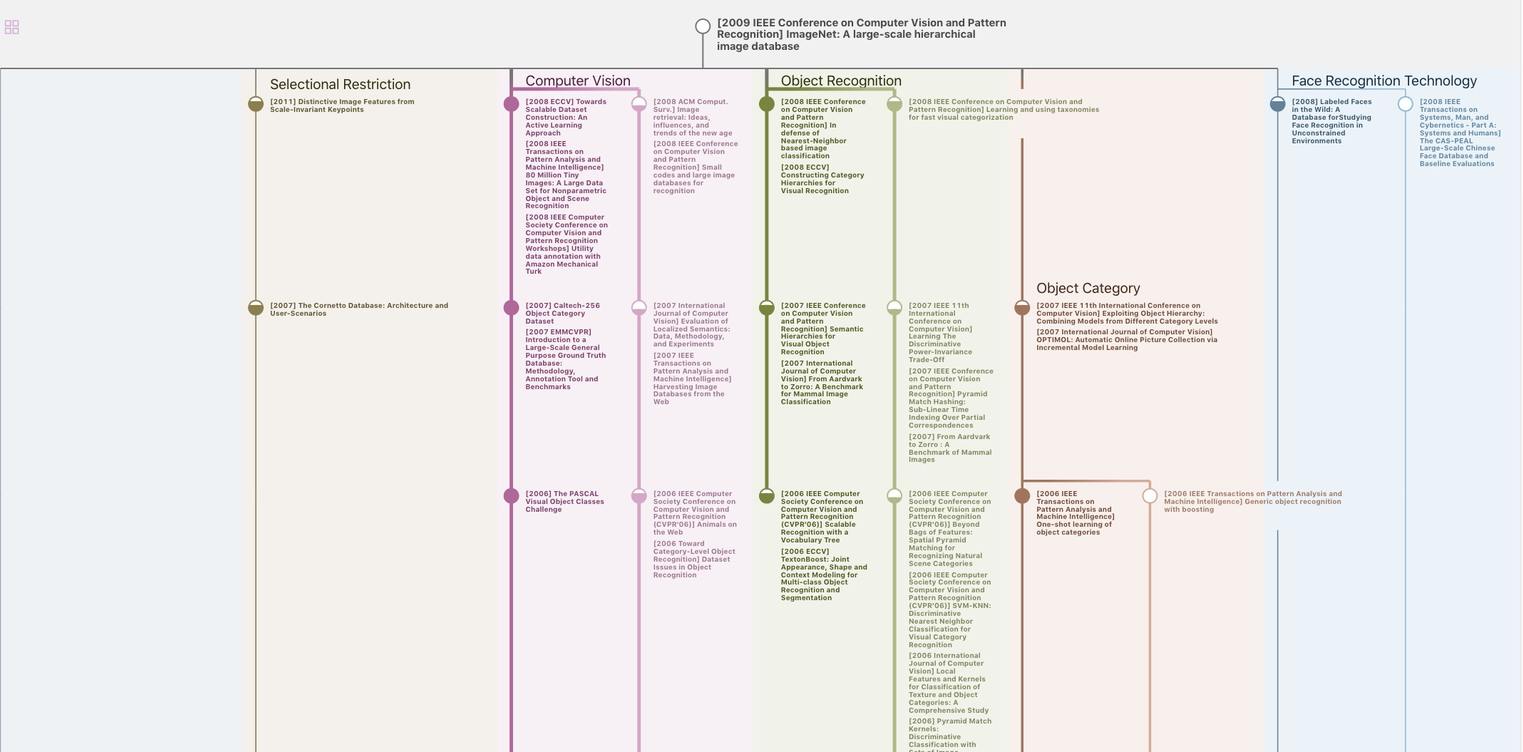
生成溯源树,研究论文发展脉络
Chat Paper
正在生成论文摘要