A Thermodynamical Model of Non-Deterministic Computation in Cortical Neural Networks
Physical biology(2023)
摘要
Neuronal populations in the cerebral cortex engage in probabilistic coding, effectively encoding the state of the surrounding environment with high accuracy and extraordinary energy efficiency. A new approach models the inherently probabilistic nature of cortical neuron signaling outcomes as a thermodynamic process of non-deterministic computation. A mean field approach is used, with the trial Hamiltonian maximizing available free energy and minimizing the net quantity of entropy, compared with a reference Hamiltonian. Thermodynamic quantities are always conserved during the computation; free energy must be expended to produce information, and free energy is released during information compression, as correlations are identified between the encoding system and its surrounding environment. Due to the relationship between the Gibbs free energy equation and the Nernst equation, any increase in free energy is paired with a local decrease in membrane potential. As a result, this process of thermodynamic computation adjusts the likelihood of each neuron firing an action potential. This model shows that non-deterministic signaling outcomes can be achieved by noisy cortical neurons, through an energy-efficient computational process that involves optimally redistributing a Hamiltonian over some time evolution. Calculations demonstrate that the energy efficiency of the human brain is consistent with this model of non-deterministic computation, with net entropy production far too low to retain the assumptions of a classical system.
更多查看译文
关键词
cortical neuron,neural computation,probabilistic coding,two-state quantum systems,quantum information,von Neumann entropy
AI 理解论文
溯源树
样例
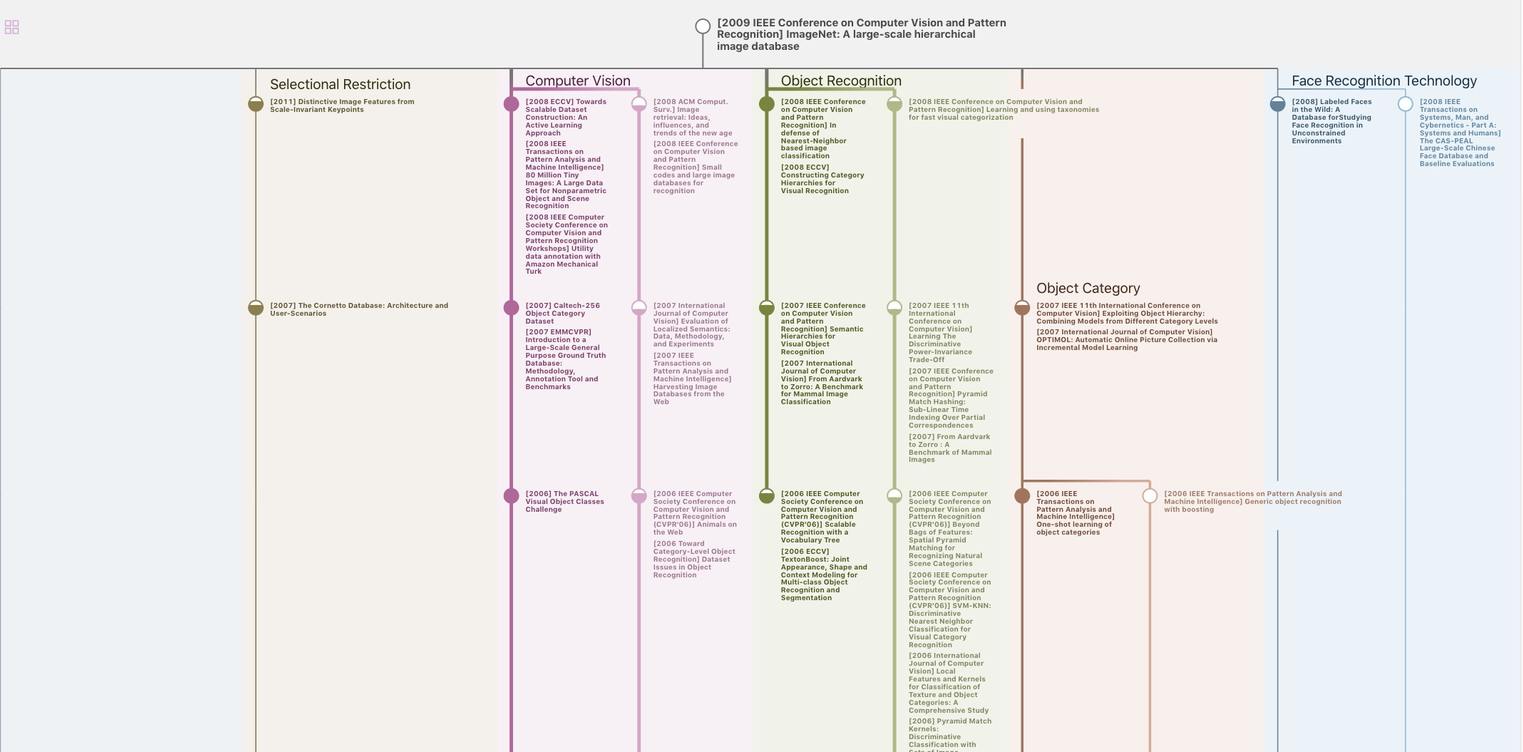
生成溯源树,研究论文发展脉络
Chat Paper
正在生成论文摘要