Data-Driven Multi-modal Partial Medical Image Preregistration by Template Space Patch Mapping
MEDICAL IMAGE COMPUTING AND COMPUTER ASSISTED INTERVENTION, MICCAI 2022, PT VI(2022)
摘要
Image registration is an essential part of Medical Image Analysis. Traditional local search methods (e.g., Mean Square Errors (MSE) and Normalized Mutual Information (NMI)) achieve accurate registration but require good initialization. However, finding a good initialization is difficult in partial image matching. Recent deep learning methods such as images-to-transformation directly solve the registration problem but need images of mostly same sizes and already roughly aligned. This work presents a learning-based method to provide good initialization for partial image registration. A light and efficient network learns the mapping from a small patch of an image to a position in the template space for each modality. After computing such mapping for a set of patches, we compute a rigid transformation matrix that maps the patches to the corresponding target positions. We tested our method to register a 3DRA image of a partial brain to a CT image of a whole brain. The result shows that MSE registration with our initialization significantly outperformed baselines including naive initialization and recent deep learning methods without template. You can access our source code in https://github.com/ApisXia/PartialMedPreregistration.
更多查看译文
关键词
Multi-modal, Partial image, Preregistration, Patch mapping
AI 理解论文
溯源树
样例
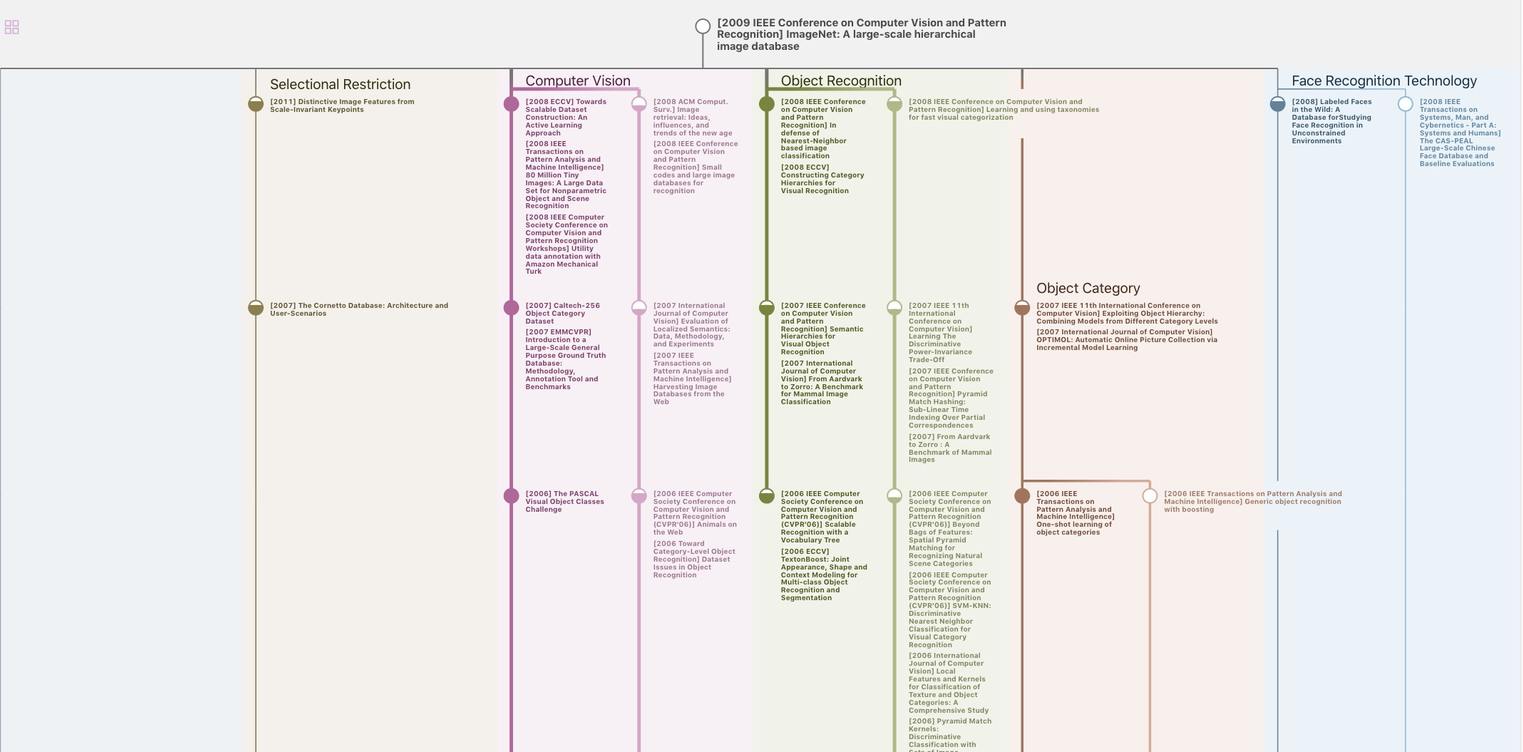
生成溯源树,研究论文发展脉络
Chat Paper
正在生成论文摘要