CRMRL: Collaborative Relationship Meta Reinforcement Learning for Effectively Adapting to Type Changes in Multi-Robotic System
IEEE ROBOTICS AND AUTOMATION LETTERS(2022)
摘要
Multi-agent reinforcement learning methods have been widely used for multi-robotic systems, and meta-learning methods are also applied to help robots reuse prior experiences to guide new tasks learning. But in some multi-robotic tasks, the robot types cannot he determined in advance or may dynamically change in practical application, bringing urgent challenges. When the robot types change, the prior experiences may he outdated and useless for new robots, even leading to negative transfer. It significantly limits the performance of most existing meta reinforcement learning methods. We get inspiration from the structure of bees, ants, and neurons. These colonies can overcome the interference caused by individual replacement, and always maintain dynamic stability. We find the relationship among individuals is more important than individuals themselves in these colonies, and propose a collaborative relationship meta reinforcement learning method (CRMRL). It concentrates on the relationship among robots, and reuses the collective knowledge to alleviate the interference caused by robot changes, dealing with the challenge of different combinations and dynamic changes in multi-robotic systems. The experiments are carried out on the StarCraft II platform and Webots simulator. The extensive results indicate that our method has noticeable improvement on many indicators compared with the traditional meta reinforcement learning methods.
更多查看译文
关键词
Distributed robot systems, multi-robot system, reinforcement learning
AI 理解论文
溯源树
样例
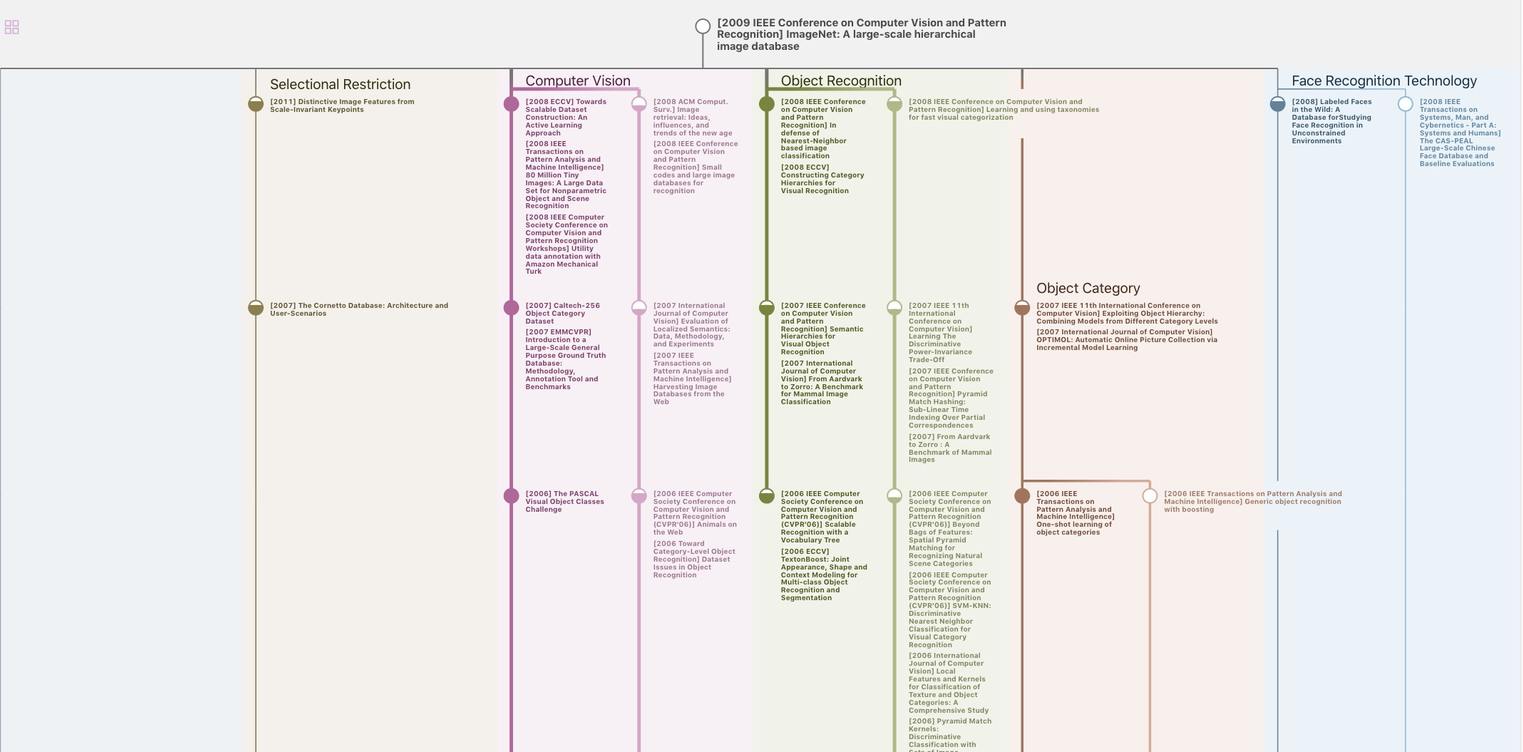
生成溯源树,研究论文发展脉络
Chat Paper
正在生成论文摘要