On turning Black- into Dark Gray-optimization with the Direct Empirical Linkage Discovery and Partition Crossover
PROCEEDINGS OF THE 2022 GENETIC AND EVOLUTIONARY COMPUTATION CONFERENCE (GECCO'22)(2022)
摘要
Gray-box optimization employs the knowledge about the true direct gene dependencies represented by the Variable Interaction Graph (VIG). This knowledge is utilized in many ways, e.g., for improving the fitness computation efficiency and proposing more effective operators for Genetic Algorithms (GAs). In the Black-box optimization, the underlying problem structure is not known. Therefore, linkage learning techniques were proposed to at least approximate gene relations and improve the evolutionary search. However, since these techniques are based on predictions, they were not suitable for building VIG. The proposition of Empirical Linkage Learning (ELL) has changed the situation. In ELL, the prediction that two genes are dependent is replaced by certainty. Additionally, ELL techniques are proven never to mark two independent genes as dependent. Therefore, we use ELL to build the empirical VIG and propose the fusion of ELL and the Gray-box operators. On this base, we propose two new mechanisms that detect (with certainty) the missing linkage between two groups of genes and the fact that the population is stuck. We integrate these mechanisms with a Gray-box operator (partition crossover) in the proposed Dark Gray Genetic Algorithm that is shown highly competitive to other state-of-the-art GAs.
更多查看译文
关键词
Linkage Learning, Model Building, Empirical linkage learning, Genetic Algorithms, Gray-box Optimization, Dark Gray Box Optimization
AI 理解论文
溯源树
样例
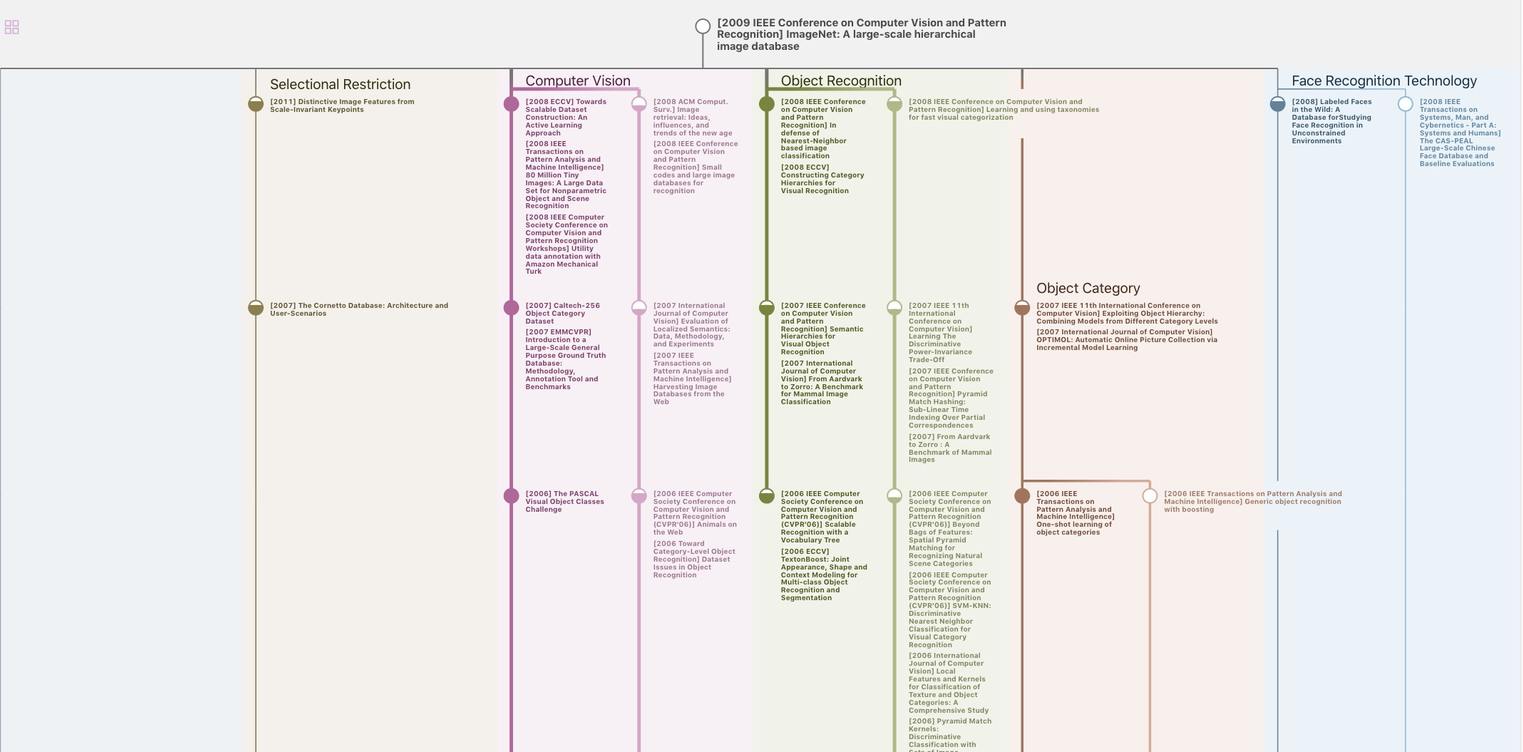
生成溯源树,研究论文发展脉络
Chat Paper
正在生成论文摘要